3.01 Quiz Introduction Connections Between Data
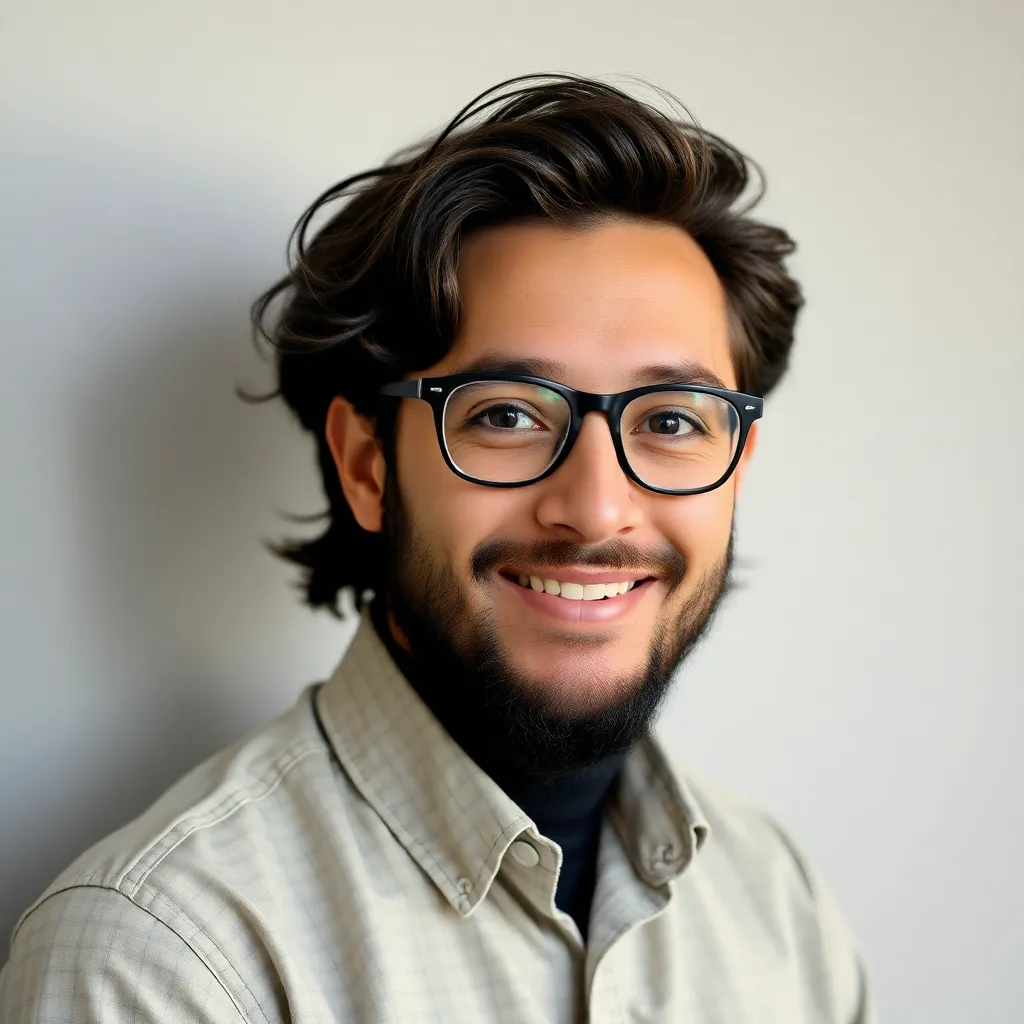
Onlines
Apr 27, 2025 · 6 min read

Table of Contents
3.01 Quiz Introduction: Connections Between Data
This comprehensive guide delves into the fascinating world of data connections, a crucial concept within the context of a 3.01 quiz (assuming this refers to an introductory data analysis or statistics course). We'll explore various types of data relationships, methods for identifying them, and their importance in drawing meaningful conclusions from datasets. This isn't just about memorizing formulas; it's about understanding the why behind the analysis, building a strong foundation for your data journey.
Understanding Data Relationships: Beyond the Obvious
Before we dive into specific types of connections, let's establish a common understanding. Data relationships, or correlations, describe how different variables within a dataset relate to each other. These relationships aren't always straightforward; sometimes they're subtle, requiring careful analysis to uncover. Understanding these relationships is fundamental to making informed decisions based on data. Ignoring these connections can lead to misleading conclusions and flawed interpretations.
The Importance of Context
One crucial aspect often overlooked is the importance of context. A strong correlation between two variables doesn't automatically imply causation. For example, a high correlation between ice cream sales and drowning incidents doesn't mean ice cream causes drowning. Both are likely influenced by a third variable: hot weather. This highlights the need for critical thinking and considering potential confounding factors when interpreting data relationships.
Types of Data Relationships: A Deep Dive
Let's explore some common types of data relationships you'll likely encounter in your 3.01 quiz and beyond:
1. Positive Correlation: Growing Together
A positive correlation means that as one variable increases, the other variable also tends to increase. This relationship is often represented visually by an upward-sloping line in a scatter plot. Examples include:
- Height and Weight: Taller individuals tend to weigh more.
- Study Time and Exam Scores: More study time often leads to better exam scores.
- Income and Spending: Higher income often correlates with higher spending.
The strength of the positive correlation can vary. A strong positive correlation shows a close relationship where the variables move together consistently, while a weak positive correlation indicates a less pronounced relationship, with more variability.
2. Negative Correlation: Moving in Opposite Directions
A negative correlation means that as one variable increases, the other variable tends to decrease. Visually, this is represented by a downward-sloping line in a scatter plot. Examples include:
- Exercise and Body Fat Percentage: Increased exercise often leads to a lower body fat percentage.
- Price and Demand: Higher prices often lead to lower demand for a product.
- Unemployment Rate and Consumer Confidence: Higher unemployment rates often correlate with lower consumer confidence.
Similar to positive correlations, the strength of negative correlations can vary, ranging from strong to weak.
3. No Correlation: Independent Variables
In this case, there's no discernible relationship between the two variables. Changes in one variable don't seem to affect the other. A scatter plot would show a random distribution of points with no clear pattern. Examples include:
- Shoe Size and IQ: There's no expected relationship between these two variables.
- Hair Color and Driving Ability: No inherent connection exists between these variables.
4. Non-Linear Correlation: Beyond Straight Lines
While positive and negative correlations are often depicted as straight lines, real-world relationships are not always linear. Non-linear correlations describe relationships where the association between variables isn't a simple straight line. These relationships can take many forms, such as:
- U-shaped: The relationship starts low, increases to a peak, then decreases.
- Inverted U-shaped: The opposite of a U-shaped relationship.
- Exponential: One variable increases rapidly in response to changes in the other.
- Logarithmic: One variable increases slowly in response to changes in the other.
Identifying non-linear correlations often requires more sophisticated analytical techniques and visual inspection of scatter plots.
Identifying Data Connections: Tools and Techniques
Several methods help identify data connections:
1. Scatter Plots: Visualizing Relationships
Scatter plots are invaluable tools for visualizing the relationship between two variables. Each point on the plot represents a data point, showing the values of both variables. The overall pattern of the points reveals the type and strength of the correlation.
2. Correlation Coefficients: Quantifying Relationships
Correlation coefficients, such as Pearson's r, provide a numerical measure of the strength and direction of a linear correlation. A value of +1 indicates a perfect positive correlation, -1 a perfect negative correlation, and 0 indicates no linear correlation. The closer the absolute value is to 1, the stronger the correlation.
3. Regression Analysis: Modeling Relationships
Regression analysis goes beyond simply measuring correlation. It aims to model the relationship between variables, allowing for prediction and understanding the impact of one variable on another. Simple linear regression models the relationship between two variables, while multiple linear regression extends this to incorporate multiple independent variables.
4. Statistical Significance Testing: Confirming Findings
Statistical significance testing helps determine if the observed correlation is likely due to chance or represents a true relationship within the population. P-values are often used to assess the significance of the correlation. A low p-value (typically below 0.05) indicates that the correlation is statistically significant, meaning it's unlikely to have occurred randomly.
Beyond Simple Relationships: Understanding Complexity
In many real-world scenarios, data relationships are far more complex than simple linear correlations. Factors to consider include:
- Causation vs. Correlation: A strong correlation doesn't necessarily imply causation. Always consider potential confounding variables and alternative explanations.
- Multicollinearity: In datasets with multiple variables, multicollinearity refers to a high correlation between independent variables, which can complicate regression analysis.
- Outliers: Extreme data points can significantly influence correlation measures. Careful examination for outliers is crucial.
- Data Transformation: Sometimes, transforming variables (e.g., using logarithms or square roots) can linearize non-linear relationships, making them easier to analyze.
Preparing for Your 3.01 Quiz: Key Strategies
To excel in your 3.01 quiz on data connections, focus on these key strategies:
- Master the Definitions: Ensure you thoroughly understand the definitions of positive, negative, and no correlation, including their visual representations in scatter plots.
- Practice Interpretation: Practice interpreting scatter plots and correlation coefficients to identify the type and strength of relationships.
- Understand Limitations: Recognize the limitations of correlation analysis, particularly the distinction between correlation and causation.
- Apply Techniques: Familiarize yourself with the methods used to identify data connections, including scatter plots, correlation coefficients, and regression analysis.
- Solve Practice Problems: Work through numerous practice problems to solidify your understanding and identify areas needing improvement.
Conclusion: Data Connections – A Foundation for Insight
Understanding data connections is a fundamental skill in data analysis and statistics. By mastering the concepts discussed in this guide, you'll be well-equipped to tackle your 3.01 quiz and confidently interpret data relationships in various contexts. Remember that data analysis is not just about numbers; it's about uncovering stories, insights, and knowledge hidden within the data. The ability to identify and understand connections between data points is the key to unlocking these hidden narratives. Keep practicing, keep questioning, and keep exploring the fascinating world of data!
Latest Posts
Latest Posts
-
Color By Number Food Webs Answer Key
Apr 27, 2025
-
To Kill A Mockingbird Chapter 11 Summary
Apr 27, 2025
-
8 2 8 Lab Using Wireshark To Examine Ethernet Frames
Apr 27, 2025
-
Tracing Bills Of Lading To Sales Invoices Provides Evidence That
Apr 27, 2025
-
Happy Go Lucky Electric Company Is The Only Company
Apr 27, 2025
Related Post
Thank you for visiting our website which covers about 3.01 Quiz Introduction Connections Between Data . We hope the information provided has been useful to you. Feel free to contact us if you have any questions or need further assistance. See you next time and don't miss to bookmark.