3-3 Discussion Confidence Intervals And Hypothesis Testing
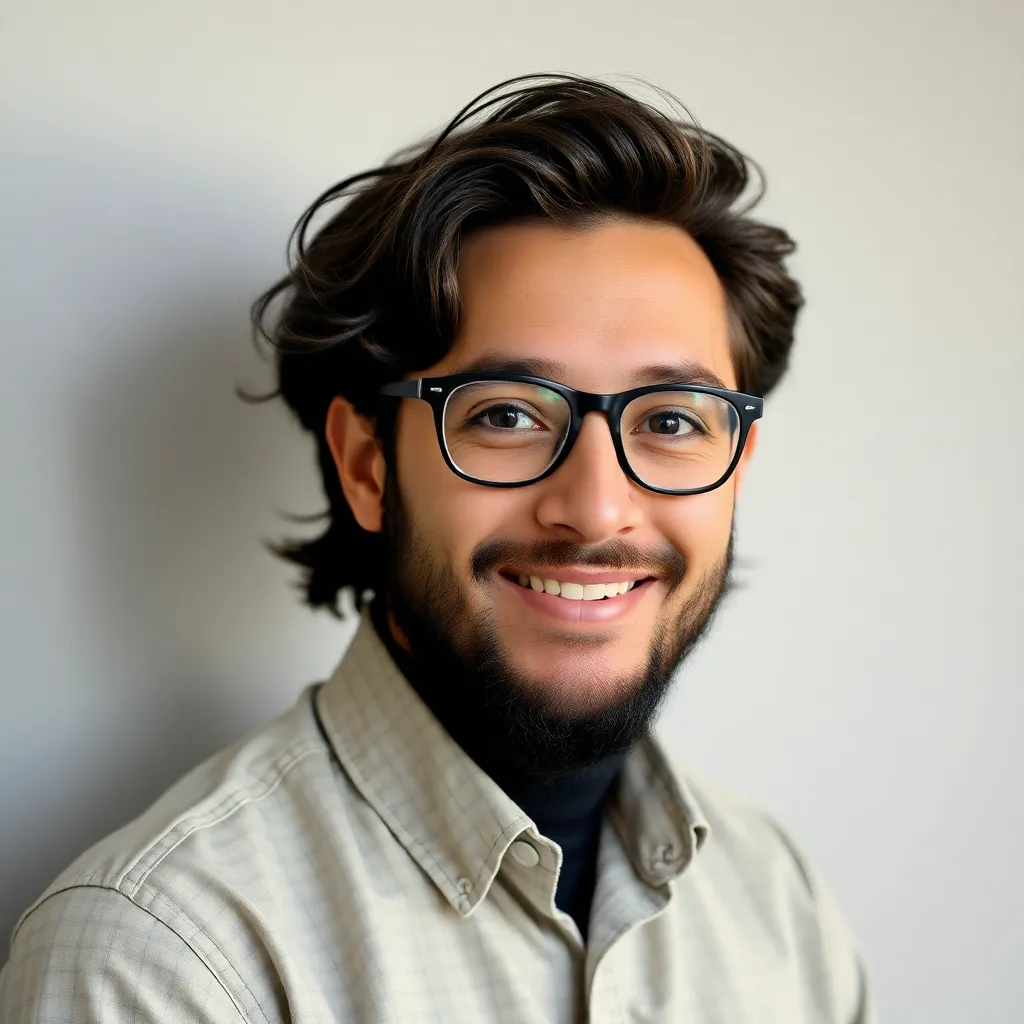
Onlines
Apr 08, 2025 · 8 min read

Table of Contents
3-3 Discussion: Confidence Intervals and Hypothesis Testing
This article delves into the crucial concepts of confidence intervals and hypothesis testing, focusing on their relationship and practical applications in statistical analysis. We'll explore the core principles, underlying assumptions, and the crucial differences between these two essential inferential statistical methods. Understanding these concepts is vital for anyone working with data, whether in research, business, or any other field that relies on data-driven decision-making.
What are Confidence Intervals?
Confidence intervals provide a range of values within which a population parameter is likely to fall, with a certain level of confidence. Instead of providing a single point estimate (like the sample mean), a confidence interval offers a margin of error, acknowledging the inherent uncertainty associated with estimating population parameters from sample data.
Key Components of a Confidence Interval:
- Point Estimate: This is the best guess of the population parameter based on the sample data. For example, the sample mean is a point estimate of the population mean.
- Margin of Error: This quantifies the uncertainty associated with the point estimate. A larger margin of error indicates greater uncertainty.
- Confidence Level: This represents the probability that the true population parameter lies within the calculated interval. Common confidence levels include 90%, 95%, and 99%. A 95% confidence interval, for example, means that if we were to repeat the sampling process many times, 95% of the resulting confidence intervals would contain the true population parameter.
How Confidence Intervals are Calculated:
The calculation of a confidence interval depends on the parameter being estimated (mean, proportion, etc.), the sample size, the sample standard deviation (or variance), and the chosen confidence level. The general formula involves the point estimate plus or minus a critical value (obtained from a relevant distribution like the t-distribution or z-distribution) multiplied by the standard error.
Example: Imagine we're estimating the average height of adult women in a city. We take a sample of 100 women and find the sample mean height to be 5'4" with a standard deviation of 3". Using a 95% confidence level, we calculate a confidence interval, which might be 5'3" to 5'5". This means we are 95% confident that the true average height of all women in the city lies between 5'3" and 5'5".
Interpreting Confidence Intervals:
It's crucial to correctly interpret confidence intervals. A common misconception is that a 95% confidence interval means there's a 95% probability that the true population parameter lies within the calculated interval. Instead, it means that if we were to repeatedly sample and calculate confidence intervals using the same method, 95% of those intervals would contain the true population parameter. The true parameter is fixed; it is the interval that varies.
What is Hypothesis Testing?
Hypothesis testing is a statistical method used to make inferences about a population parameter based on sample data. It involves formulating a hypothesis (a statement about the population parameter), collecting data, and then using statistical tests to determine whether the data provides enough evidence to reject the hypothesis.
Key Components of Hypothesis Testing:
- Null Hypothesis (H0): This is the statement that we are trying to disprove. It usually represents the status quo or a default assumption. For example, the null hypothesis might be that there is no difference between the means of two groups.
- Alternative Hypothesis (H1 or Ha): This is the statement that we are trying to prove. It is the opposite of the null hypothesis. For example, the alternative hypothesis might be that there is a difference between the means of two groups.
- Test Statistic: This is a numerical value calculated from the sample data that is used to assess the evidence against the null hypothesis.
- P-value: This is the probability of observing the obtained results (or more extreme results) if the null hypothesis were true. A small p-value provides evidence against the null hypothesis.
- Significance Level (α): This is a predetermined threshold (typically 0.05 or 5%) used to decide whether to reject the null hypothesis. If the p-value is less than the significance level, the null hypothesis is rejected.
Types of Hypothesis Tests:
There are various types of hypothesis tests, depending on the type of data and the research question. Some common types include:
- One-sample t-test: Used to compare the mean of a single sample to a known population mean.
- Two-sample t-test: Used to compare the means of two independent samples.
- Paired t-test: Used to compare the means of two related samples (e.g., before and after measurements).
- Chi-square test: Used to analyze categorical data and assess the association between two categorical variables.
- ANOVA (Analysis of Variance): Used to compare the means of three or more groups.
Example: Let's say we want to test whether a new drug is effective in lowering blood pressure. The null hypothesis would be that the drug has no effect (mean blood pressure remains the same). The alternative hypothesis would be that the drug lowers blood pressure (mean blood pressure decreases). We would collect data from a sample of patients, calculate a test statistic (e.g., a t-statistic), and determine the p-value. If the p-value is less than the significance level (e.g., 0.05), we would reject the null hypothesis and conclude that the drug is effective.
Relationship Between Confidence Intervals and Hypothesis Testing
Confidence intervals and hypothesis testing are closely related but address different aspects of statistical inference. They both use sample data to make inferences about population parameters. Importantly, a confidence interval can be used to perform a hypothesis test, and vice-versa.
Confidence Interval Approach to Hypothesis Testing:
Consider a hypothesis test about a population mean (μ). The null hypothesis might be that μ = μ0 (where μ0 is a specified value). A 95% confidence interval for μ is calculated. If the value μ0 lies outside the 95% confidence interval, then the null hypothesis would be rejected at the 5% significance level. This is because the observed data provides strong evidence that the true population mean is different from μ0. If μ0 falls within the confidence interval, we would fail to reject the null hypothesis.
Hypothesis Testing Approach to Confidence Interval Construction:
Conversely, information from a hypothesis test can inform the construction of a confidence interval. The test statistic and p-value provide information about the location and width of a plausible confidence interval for the parameter of interest.
Key Differences:
While closely linked, confidence intervals and hypothesis testing have some key differences:
- Focus: Confidence intervals focus on estimating the range of plausible values for a population parameter, whereas hypothesis testing focuses on testing a specific hypothesis about a population parameter.
- Output: Confidence intervals provide a range of values, while hypothesis testing yields a decision (reject or fail to reject the null hypothesis).
- Interpretation: Confidence intervals are interpreted as a range of plausible values, while hypothesis testing results are interpreted in terms of statistical significance.
Assumptions Underlying Confidence Intervals and Hypothesis Testing
Both confidence intervals and hypothesis testing rely on certain assumptions regarding the data. These assumptions are crucial for the validity of the results. Violations of these assumptions can lead to inaccurate conclusions. The specific assumptions vary depending on the statistical method being used, but some common assumptions include:
- Random Sampling: The data should be collected using a random sampling method to ensure that the sample is representative of the population.
- Independence: The observations in the sample should be independent of each other. This means that the value of one observation should not influence the value of another.
- Normality: For many methods, particularly those involving means, the data should be approximately normally distributed or the sample size should be sufficiently large for the central limit theorem to apply.
- Homogeneity of Variance (for some tests): In tests comparing means from multiple groups, the variances of the groups should be roughly equal.
Practical Applications
Confidence intervals and hypothesis testing are widely used in numerous fields, including:
- Medical Research: Testing the effectiveness of new drugs or treatments.
- Business and Marketing: Analyzing customer satisfaction, market trends, and the effectiveness of advertising campaigns.
- Social Sciences: Studying the relationships between social variables and testing hypotheses about social phenomena.
- Environmental Science: Assessing environmental impacts and monitoring changes in ecological systems.
- Engineering: Evaluating the reliability and performance of products and systems.
Conclusion
Confidence intervals and hypothesis testing are fundamental tools in statistical inference. They provide valuable methods for drawing conclusions about population parameters based on sample data. Understanding their strengths, limitations, and interrelationships is crucial for anyone involved in data analysis and interpretation. Remembering the assumptions and correctly interpreting the results are key to making informed decisions based on statistical evidence. While both methods serve to analyze data, their focus and outputs differ, allowing researchers to choose the method best suited to their specific needs and research questions. The choice often depends on whether the goal is to estimate a range of plausible values (confidence intervals) or to test a specific hypothesis (hypothesis testing). Proficiency in both is vital for robust statistical analysis and evidence-based decision-making.
Latest Posts
Latest Posts
-
The Table Represents A Function What Is F 5
Apr 16, 2025
-
What Are The Themes Of The Scarlet Letter
Apr 16, 2025
-
To Kill A Mockingbird Plot Graph
Apr 16, 2025
-
Po Box 70219 Philadelphia Pa 19176
Apr 16, 2025
-
Deep History Of Life On Earth Worksheet Answers
Apr 16, 2025
Related Post
Thank you for visiting our website which covers about 3-3 Discussion Confidence Intervals And Hypothesis Testing . We hope the information provided has been useful to you. Feel free to contact us if you have any questions or need further assistance. See you next time and don't miss to bookmark.