7.5.5 Coin Flip Fun: Number Of Heads And Tails
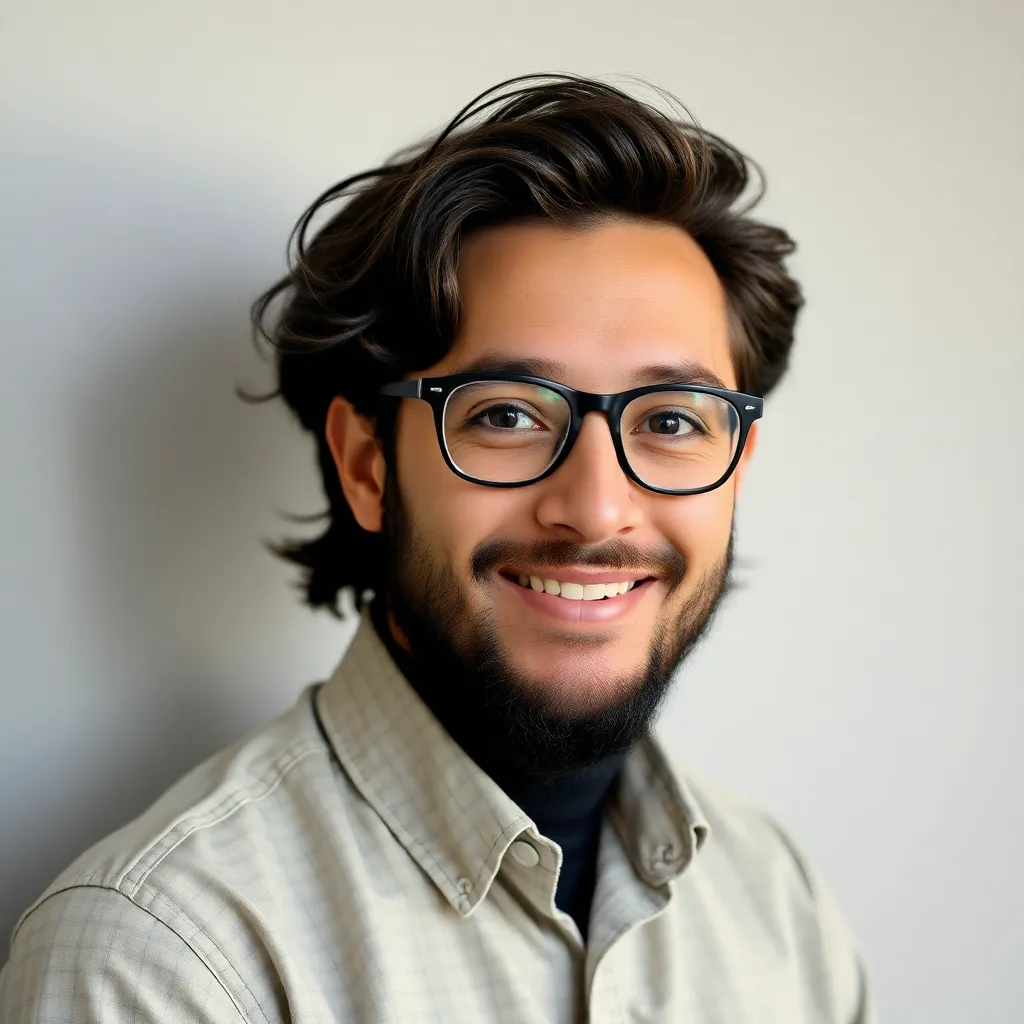
Onlines
Apr 08, 2025 · 6 min read

Table of Contents
7.5.5 Coin Flip Fun: Delving Deep into the Probabilities of Heads and Tails
Coin flipping. A seemingly simple act, yet within its seemingly random nature lies a fascinating world of probability and statistics. This article dives deep into the mathematics behind coin flips, exploring the probabilities of getting a specific number of heads or tails in a series of tosses. We'll go beyond the basic 50/50 chance and uncover the intricacies of binomial distribution, its applications, and how to calculate probabilities for various scenarios. Prepare for a fun and insightful journey into the world of coin flip statistics!
Understanding the Basics: Probability and the Binomial Distribution
Before we delve into the complexities of multiple coin flips, let's establish a solid foundation. The probability of getting heads (or tails) on a single, fair coin flip is 1/2 or 50%. This is because there are two equally likely outcomes: heads or tails.
However, things get more interesting when we consider multiple coin flips. This is where the binomial distribution comes into play. The binomial distribution is a probability distribution that describes the probability of getting a certain number of successes (in our case, heads or tails) in a fixed number of independent Bernoulli trials (coin flips).
A key characteristic of a binomial distribution is that each trial is independent. The outcome of one coin flip doesn't influence the outcome of another. This independence is crucial for accurately applying the binomial distribution.
The binomial distribution is defined by two parameters:
- n: The number of trials (coin flips).
- p: The probability of success on a single trial (probability of getting heads, which is 0.5 for a fair coin).
Calculating Probabilities: The Binomial Probability Formula
The probability of getting exactly k successes (heads, for instance) in n trials is given by the binomial probability formula:
P(X = k) = (nCk) * p^k * (1-p)^(n-k)
Where:
- P(X = k): The probability of getting exactly k successes.
- nCk: The number of combinations of n items taken k at a time (also written as ⁿCₖ or C(n,k)). This is calculated as n! / (k! * (n-k)!), where ! denotes the factorial (e.g., 5! = 5 * 4 * 3 * 2 * 1).
- p: The probability of success on a single trial (0.5 for a fair coin flip).
- (1-p): The probability of failure (getting tails, in this case, also 0.5).
Let's illustrate this with an example:
Scenario: What's the probability of getting exactly 3 heads in 5 coin flips?
Here, n = 5, k = 3, and p = 0.5. Plugging these values into the formula:
P(X = 3) = (5C3) * (0.5)^3 * (0.5)^(5-3) = 10 * 0.125 * 0.25 = 0.3125
Therefore, the probability of getting exactly 3 heads in 5 coin flips is 31.25%.
Exploring Different Scenarios: Varying Number of Flips and Desired Outcomes
Now, let's explore various scenarios to understand how the probability changes with the number of coin flips and the desired number of heads or tails:
Scenario 1: 10 Coin Flips
Let's calculate the probabilities for different numbers of heads in 10 coin flips:
- P(X = 0 heads): Very low probability, as getting zero heads in ten flips is unlikely.
- P(X = 5 heads): This is the most likely outcome, representing the peak of the binomial distribution for this scenario.
- P(X = 10 heads): Similar to getting 0 heads, the probability is very low.
You can use the binomial probability formula to calculate the exact probabilities for each outcome (0 to 10 heads). You'll observe a bell-shaped curve, characteristic of the binomial distribution.
Scenario 2: The Impact of the Number of Flips
As the number of coin flips (n) increases, the binomial distribution becomes more symmetrical and approaches a normal distribution. This means the probabilities become more concentrated around the expected value (n * p), which is the average number of heads you'd expect to get. For a fair coin, this is simply n/2.
Scenario 3: Unfair Coins
So far, we've assumed a fair coin (p = 0.5). But what if the coin is biased? Let's say the probability of getting heads is 0.6 (a biased coin favoring heads). The binomial probability formula still applies, but with p = 0.6. This will shift the distribution, making outcomes with more heads more likely.
Beyond Calculation: Simulation and Visualization
While the binomial probability formula provides precise calculations, simulations can offer a more intuitive understanding. You can simulate coin flips using programming languages like Python or R. These simulations can visually represent the distribution of heads and tails over many trials, reinforcing the concepts we've discussed. Visualizations, like histograms, clearly illustrate the probabilities of different outcomes.
Real-World Applications of Binomial Distribution in Coin Flips and Beyond
The seemingly simple coin flip and its associated binomial distribution have surprisingly wide-ranging applications:
- Quality Control: Imagine testing a batch of manufactured products. Each product can be considered a "trial," and whether it's defective or not is the "success" or "failure." The binomial distribution helps determine the probability of finding a certain number of defective products in a sample.
- Medical Trials: In clinical trials, the binomial distribution is used to analyze the effectiveness of a treatment. Each patient is a trial, and a successful outcome (e.g., recovery) is a "success."
- Genetics: The inheritance of certain traits follows binomial probabilities. For example, the probability of a child inheriting a specific gene from their parents can be modeled using the binomial distribution.
- Sports Analytics: In sports, the binomial distribution can be used to analyze the probability of a team winning a series of games, considering the individual game win probabilities.
Advanced Concepts: Expected Value and Variance
Two important concepts associated with the binomial distribution are:
- Expected Value (E[X]): This represents the average number of successes you'd expect to get over many trials. For the binomial distribution, E[X] = n * p.
- Variance (Var(X)): This measures the spread or dispersion of the distribution. For the binomial distribution, Var(X) = n * p * (1-p). A higher variance indicates a wider spread of possible outcomes.
Conclusion: Coin Flips – A Gateway to Understanding Probability
The seemingly simple act of flipping a coin unveils a rich world of probability and statistics. By understanding the binomial distribution and its associated concepts, we can accurately predict the probabilities of various outcomes in a series of coin flips. These principles extend far beyond coin flips, finding application in numerous fields, from quality control to medical research. So, the next time you flip a coin, remember the intricate mathematics hidden beneath its seemingly random behavior. It's a testament to the power of probability and its ability to model and understand seemingly unpredictable events in our world. Further exploration into statistical concepts like confidence intervals and hypothesis testing will further enhance your understanding of probability and its applications. This journey into the world of coin flips is just the beginning of a deeper understanding of statistics and its role in various aspects of life.
Latest Posts
Latest Posts
-
Student Exploration Unit Conversions Gizmo Answers
Apr 17, 2025
-
Planning Scheduling And Tracking Cdbs Is Completed On What System
Apr 17, 2025
-
Modelo You Hear No Entiendo El Problema You Write Entender
Apr 17, 2025
-
Fact Value Policy Worksheet Answers Pdf
Apr 17, 2025
-
Icivics Sources Of Law Answer Key
Apr 17, 2025
Related Post
Thank you for visiting our website which covers about 7.5.5 Coin Flip Fun: Number Of Heads And Tails . We hope the information provided has been useful to you. Feel free to contact us if you have any questions or need further assistance. See you next time and don't miss to bookmark.