A Critical Feature Of Multiple-baseline Designs Is That:
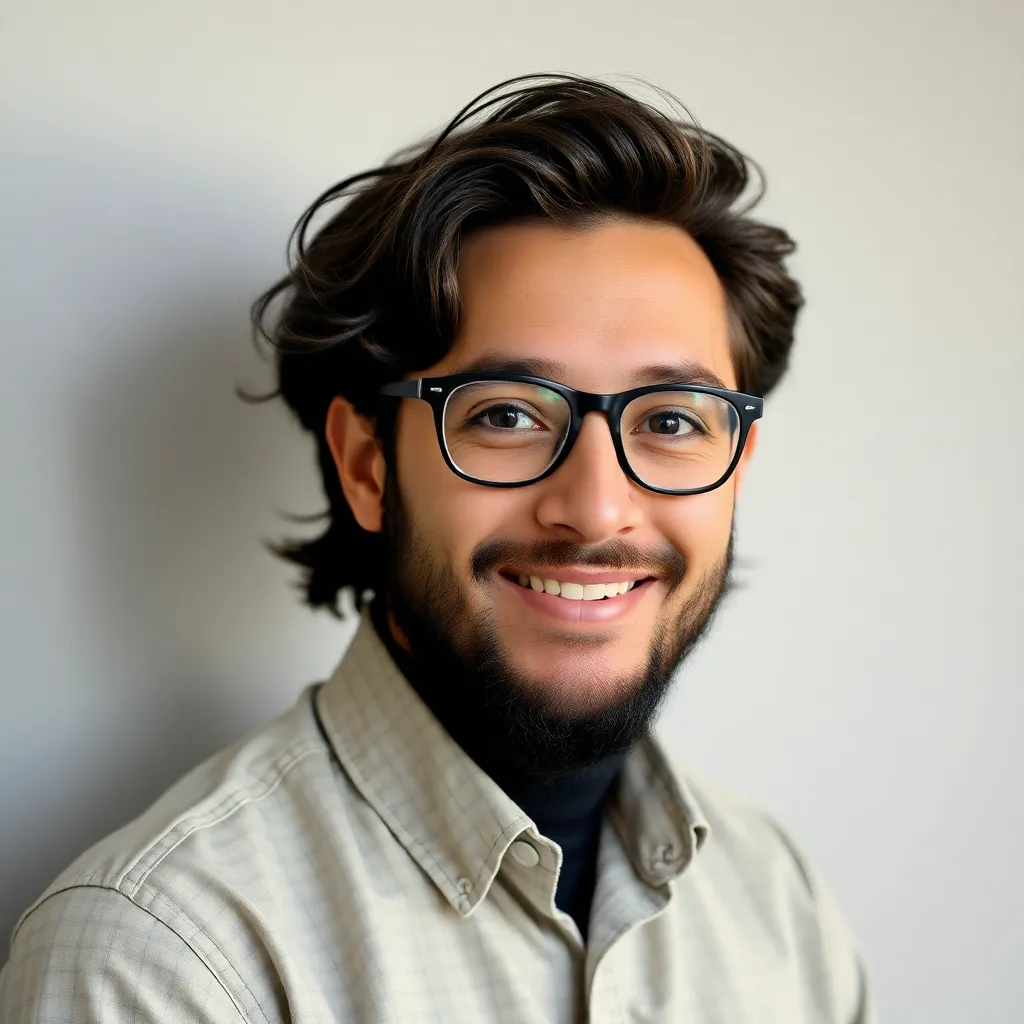
Onlines
May 11, 2025 · 7 min read
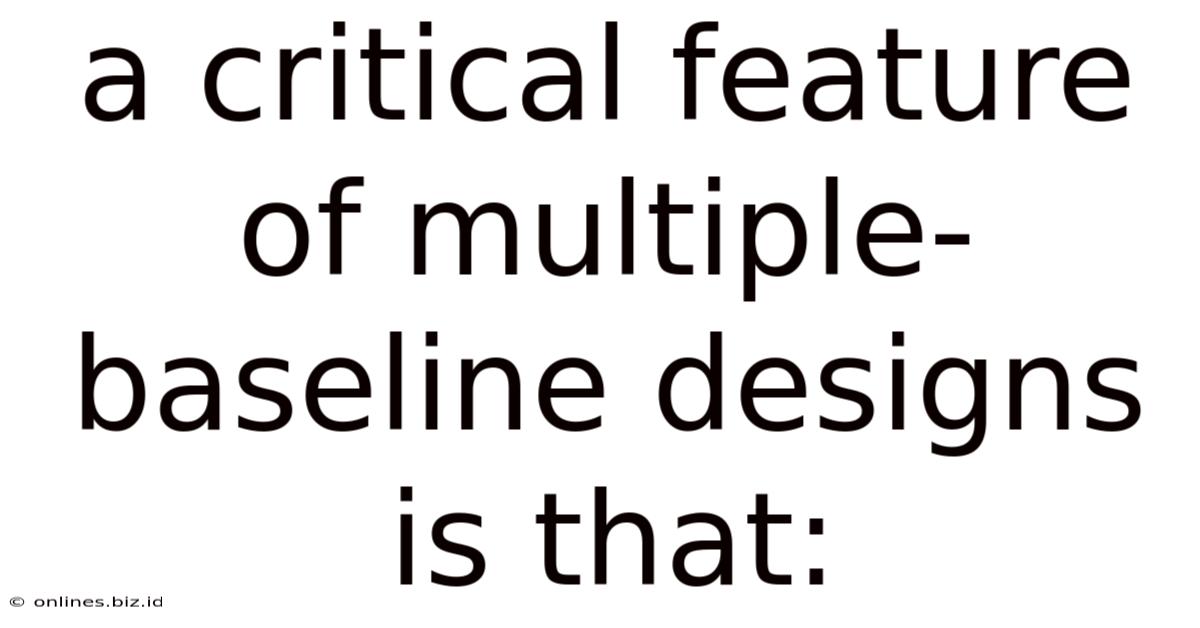
Table of Contents
A Critical Feature of Multiple-Baseline Designs: Demonstrating Functional Relationships
Multiple-baseline designs are a powerful tool in applied behavior analysis (ABA) and research methodologies across various fields. They allow researchers to demonstrate functional relationships between an intervention and a target behavior without the need for a traditional reversal design. A critical feature of multiple-baseline designs is their ability to demonstrate a strong, convincing causal relationship between the intervention and the change in behavior. This is achieved through the staggered introduction of the intervention across multiple baselines, which strengthens the internal validity of the study and minimizes the potential for confounding variables to influence the results. This article will delve deep into this critical feature, exploring the different types of multiple-baseline designs, their advantages and disadvantages, and the crucial role they play in establishing causal inferences.
Understanding Multiple-Baseline Designs
Multiple-baseline designs are experimental designs used to evaluate the effectiveness of an intervention. They involve measuring a target behavior across multiple baselines before introducing an intervention. These baselines can be across different:
Types of Multiple-Baseline Designs
-
Multiple-Baseline Across Subjects: This design involves measuring the same target behavior in several different subjects. The intervention is introduced to one subject at a time, while the other subjects continue to serve as controls. This design is particularly useful when it's unethical or impractical to withdraw an effective intervention.
-
Multiple-Baseline Across Behaviors: This design involves measuring different target behaviors in the same subject. The intervention is introduced to one behavior at a time, while the other behaviors remain unchanged. This design is useful when assessing the effectiveness of an intervention on multiple behaviors displayed by a single individual.
-
Multiple-Baseline Across Settings: This design involves measuring the same target behavior in the same subject across different settings. The intervention is introduced to one setting at a time, while the other settings serve as controls. This allows researchers to determine if the intervention is generalizable across different contexts.
The Critical Feature: Demonstrating Functional Relationships
The core strength of multiple-baseline designs lies in their ability to convincingly demonstrate a functional relationship between the intervention and the target behavior. This is achieved through the staggered introduction of the intervention. By introducing the intervention to different baselines at different times, researchers can observe whether changes in behavior are directly linked to the intervention's implementation. If the behavior changes only after the introduction of the intervention, and remains stable before, it provides strong evidence for a causal relationship.
Why is this staggered introduction so critical?
It allows for a powerful demonstration of causality because it controls for several potential threats to internal validity:
-
History: The staggered introduction minimizes the impact of extraneous events that could have affected behavior across all baselines simultaneously. If a change in behavior is observed only after the intervention is introduced, and not in the other baselines before the introduction, it suggests that the intervention, and not historical events, caused the change.
-
Maturation: The natural changes in behavior over time are controlled for because the baselines are of varying lengths. Any maturational changes would likely affect all baselines. The fact that change is only seen after intervention introduction minimizes the effect of maturation as an alternative explanation for behavioral change.
-
Testing: Repeated measurement itself might influence behavior. However, because the intervention is introduced at different times, any effects of repeated measurement would not consistently correlate with the intervention's introduction.
-
Instrumentation: Changes in the accuracy or consistency of measurement procedures are less likely to confound results because any changes would impact all baselines rather than only those exposed to the intervention.
-
Regression to the Mean: The staggered introduction helps control for regression to the mean. This statistical phenomenon occurs when extreme scores on a variable tend to be followed by less extreme scores. By introducing the intervention at different times, the effect of regression to the mean is less likely to confound the results.
Analyzing Multiple-Baseline Data
Analyzing data from a multiple-baseline design involves visually inspecting graphs of the data for each baseline. The key is to look for:
-
Stability of baseline data: Before the intervention, the data in each baseline should show a relatively stable trend (though fluctuations are expected).
-
Immediate change after intervention: After the intervention is introduced, there should be a clear and immediate change in the target behavior. This change should be consistently different from the baseline data.
-
Replication across baselines: The pattern of change should replicate across all baselines after the intervention is introduced. This replication strongly supports the conclusion that the intervention caused the change.
While visual inspection is crucial, statistical analysis can provide further support for the findings. However, there’s no single universally accepted statistical test for multiple-baseline designs. Often, researchers use visual inspection alongside descriptive statistics to make interpretations.
Advantages of Multiple-Baseline Designs
-
Ethical considerations: Multiple-baseline designs avoid the ethical concerns associated with withdrawing a potentially beneficial intervention, as required in reversal designs.
-
Practical applicability: They are adaptable to many real-world settings where it’s difficult or impossible to withdraw an intervention.
-
Generalizability: The staggered introduction and replication across baselines contribute to the generalizability of findings.
-
Flexibility: The design can be applied across various contexts (subjects, behaviors, settings) and to different target populations.
Disadvantages of Multiple-Baseline Designs
-
Lengthy data collection: The staggered introduction of the intervention necessitates longer data collection periods compared to reversal designs.
-
Limited control over extraneous variables: While it controls for many factors, multiple-baseline designs cannot completely eliminate the influence of extraneous variables.
-
Potential for interaction effects: The intervention's introduction to one baseline might influence other baselines. However, careful experimental design can minimize this risk.
-
Weak statistical power: The absence of a universally agreed-upon statistical test can limit the statistical power to detect effects compared to some other experimental designs.
Interpreting Results: What Constitutes Strong Evidence?
Strong evidence of a functional relationship in a multiple-baseline design is characterized by:
-
Clear and immediate changes in behavior following intervention introduction in each baseline. The greater the difference between baseline and post-intervention data, the stronger the evidence.
-
Consistent replication across all baselines. The more baselines that show consistent changes following intervention, the more confident the researcher can be that the intervention caused the behavioral change.
-
Minimal overlap between baseline and intervention data. This minimizes the possibility that the observed changes might be due to chance or other extraneous factors.
-
Absence of any noticeable changes in behavior in baselines before the intervention is introduced. This helps rule out alternative explanations.
Researchers should consider the context of the study when interpreting results. The stronger the evidence supporting the functional relationship, the more likely the findings are generalizable.
Multiple-Baseline Designs in Practice: Examples
Consider the following scenarios:
Scenario 1: Multiple-Baseline Across Subjects - A researcher wants to evaluate the effectiveness of a new teaching method for improving reading comprehension in three students with dyslexia. Each student receives the new teaching method at different points in time, allowing the researcher to assess whether improvements are due to the teaching method itself.
Scenario 2: Multiple-Baseline Across Behaviors - A therapist wants to reduce self-injurious behavior (biting, hitting, and scratching) in a child with autism. The therapist introduces an intervention (e.g., positive reinforcement) to address one behavior at a time, while monitoring the other behaviors. The consistent reduction of each target behavior only after the intervention is introduced would support the effectiveness of the intervention.
Scenario 3: Multiple-Baseline Across Settings - A researcher wants to examine the effectiveness of a classroom management strategy to improve student on-task behavior. The strategy is implemented in different classrooms at different times, allowing for an assessment of its generalizability.
Conclusion
Multiple-baseline designs offer a robust approach to investigating functional relationships between interventions and behavior. The staggered introduction of the intervention is the crucial element that allows for the strong demonstration of causality, minimizing the influence of alternative explanations. By carefully selecting the type of multiple-baseline design appropriate for the research question, ensuring sufficient baseline stability, and analyzing results systematically, researchers can generate compelling evidence of intervention effectiveness and contribute significantly to the understanding of behavior change. While limitations exist, multiple-baseline designs remain a valuable and widely used methodology in various fields, particularly those focused on improving human behavior. Their strength lies in their pragmatic applicability and the strong internal validity they provide when implemented correctly.
Latest Posts
Latest Posts
-
Family Business Consultants Typically Advise Parents To
May 12, 2025
-
A Car Travels Clockwise Once Around The Track Shown Below
May 12, 2025
-
A Nurse Is Reported To The Bon For Cashing Checks
May 12, 2025
-
Which Of The Following Best Exemplifies The Process Of Self Selection
May 12, 2025
-
Chapter 5 Ten Words In Context
May 12, 2025
Related Post
Thank you for visiting our website which covers about A Critical Feature Of Multiple-baseline Designs Is That: . We hope the information provided has been useful to you. Feel free to contact us if you have any questions or need further assistance. See you next time and don't miss to bookmark.