A Light Bulb Manufacturer Uses Descriptive Analytics
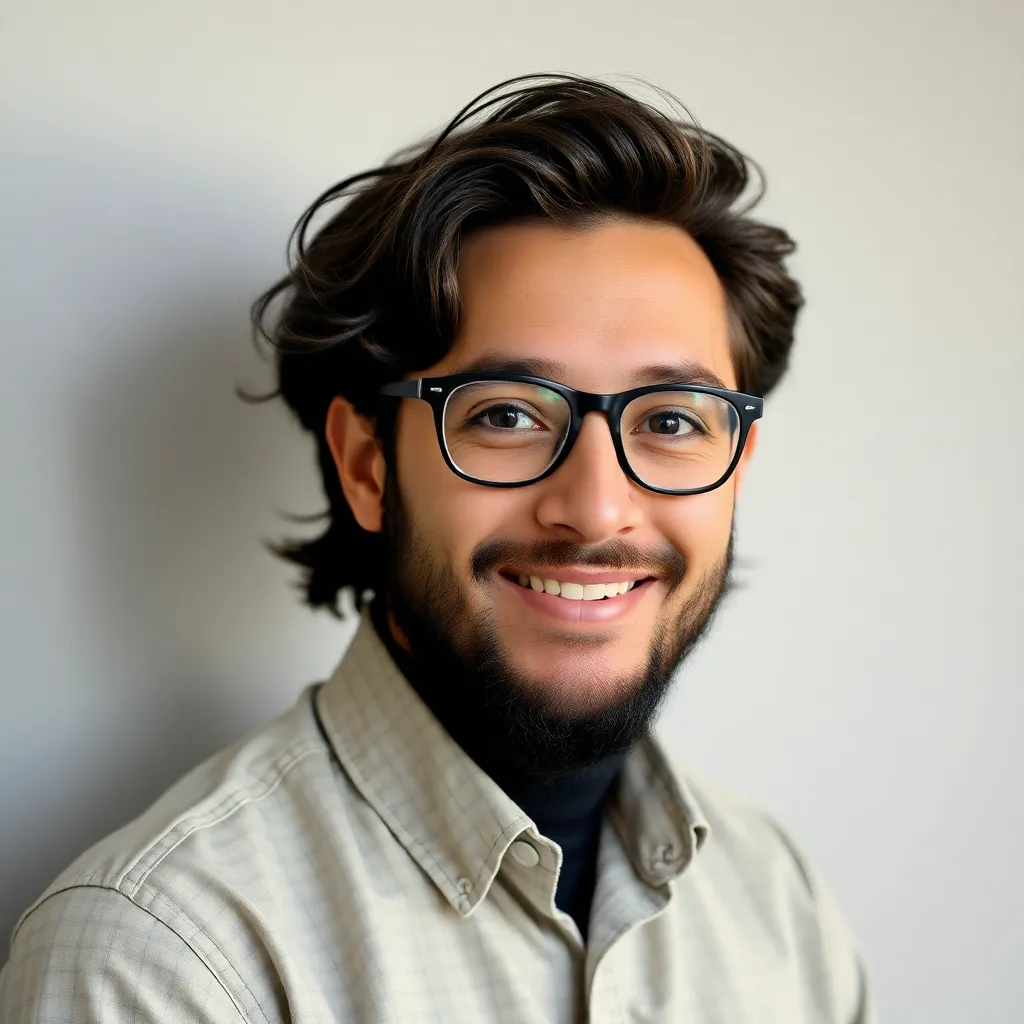
Onlines
May 08, 2025 · 6 min read
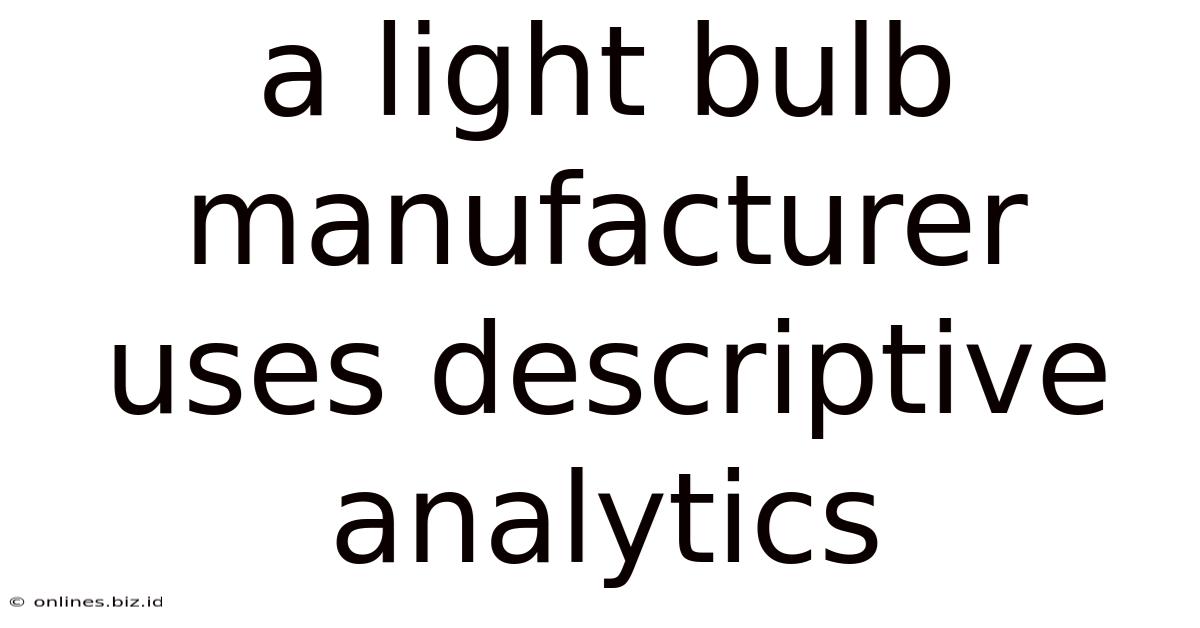
Table of Contents
Illuminating Insights: How a Light Bulb Manufacturer Uses Descriptive Analytics to Shine Brighter
The world of light bulb manufacturing is far from dim. Competition is fierce, consumer preferences are evolving, and staying ahead requires more than just a bright idea – it requires data-driven insights. This is where descriptive analytics steps in, illuminating the path to success for manufacturers like LuminaGlow, a fictional but representative example. LuminaGlow leverages the power of descriptive analytics to understand its past performance, identify key trends, and make informed decisions that drive growth and profitability.
Understanding the Power of Descriptive Analytics in Manufacturing
Descriptive analytics is the foundational layer of any data-driven strategy. It focuses on summarizing and interpreting historical data to understand what has happened. Unlike predictive or prescriptive analytics, which forecast future outcomes or recommend actions, descriptive analytics provides a clear picture of the past, enabling manufacturers to identify patterns, trends, and anomalies that can inform future strategies.
For LuminaGlow, this translates into analyzing vast amounts of data related to production, sales, marketing, and customer feedback. This data encompasses various aspects, providing a comprehensive view of their operations and market position.
Key Data Sources for LuminaGlow's Descriptive Analytics:
-
Production Data: This includes data on manufacturing output, defect rates, raw material usage, energy consumption, and machine downtime. Analyzing this data reveals areas for improvement in efficiency and cost reduction. For example, identifying recurring machine malfunctions can point towards preventative maintenance strategies.
-
Sales Data: Sales figures, broken down by product type, region, sales channel (online, retail, wholesale), and customer segment, offer critical insights into market demand, product popularity, and sales performance. This data helps LuminaGlow understand which products are selling well, where they're selling best, and which marketing campaigns are most effective.
-
Marketing Data: This includes data on website traffic, social media engagement, advertising campaign performance (clicks, conversions, cost per acquisition), and customer acquisition costs. Analyzing this data helps LuminaGlow optimize its marketing spend and target its efforts more effectively.
-
Customer Feedback Data: Customer reviews, surveys, and support tickets offer invaluable qualitative and quantitative insights into customer satisfaction, product quality, and areas for improvement. This feedback loop is crucial for product development and ensuring customer loyalty.
-
Supply Chain Data: Tracking the movement of raw materials, inventory levels, and delivery times provides insights into the efficiency and resilience of LuminaGlow's supply chain. Identifying bottlenecks or delays can help optimize logistics and prevent production disruptions.
Putting Descriptive Analytics into Action: LuminaGlow's Case Study
LuminaGlow uses a variety of descriptive analytics techniques to analyze its data and gain valuable insights. Let's look at specific examples:
1. Analyzing Production Efficiency:
LuminaGlow uses summary statistics (mean, median, mode, standard deviation) to analyze its production data. This reveals average output per hour, defect rates, and variations in production time. By identifying periods of low production or high defect rates, LuminaGlow can pinpoint potential bottlenecks or problems in the manufacturing process. For instance, a sudden spike in defect rates for a specific product line might indicate a problem with a particular machine or raw material batch, allowing for immediate corrective action.
They further employ data visualization techniques, such as line charts showing production output over time and bar charts comparing defect rates across different product lines. Visualizing data makes it easier for LuminaGlow's team to spot trends and anomalies that might otherwise go unnoticed. This allows for a proactive approach, preventing potential issues from escalating into larger problems.
2. Understanding Sales Trends:
LuminaGlow uses sales dashboards to track key sales metrics such as total revenue, sales per product, and sales by region. These dashboards provide a real-time overview of sales performance, enabling quick identification of trends and anomalies. For example, a significant drop in sales for a specific product in a particular region might indicate a localized problem, such as increased competition or a negative review.
Further analysis using correlation analysis helps identify relationships between different variables. For example, LuminaGlow might find a positive correlation between online advertising spend and sales for a particular product line. This insight helps optimize marketing budgets and allocate resources more effectively. Regression analysis can then be used to predict future sales based on past performance and marketing spend.
3. Optimizing Marketing Campaigns:
LuminaGlow uses cohort analysis to segment its customer base and track the behavior of different groups over time. This allows them to understand customer lifetime value, identify high-value customer segments, and tailor marketing messages to specific groups. For example, by analyzing the behavior of customers who purchased specific product lines, LuminaGlow can identify which marketing channels are most effective in reaching those customers.
A/B testing helps evaluate the effectiveness of different marketing materials (e.g., website copy, email subject lines, ad creatives). By comparing the performance of different versions, LuminaGlow can optimize its marketing campaigns and improve conversion rates. This process is heavily reliant on descriptive analysis to interpret the results of the tests and determine which version performed better.
4. Enhancing Customer Satisfaction:
LuminaGlow employs text mining and sentiment analysis to analyze customer feedback from surveys, reviews, and social media. This allows them to identify recurring themes, positive and negative sentiments, and areas for product improvement. For instance, consistent negative feedback regarding the durability of a particular product line indicates a need for design improvements or quality control enhancements. This data is invaluable in proactive customer relationship management (CRM) efforts and product development cycles.
5. Improving Supply Chain Efficiency:
LuminaGlow utilizes descriptive analytics to analyze supply chain data, focusing on lead times, inventory levels, and transportation costs. By identifying bottlenecks and inefficiencies in the supply chain, they can optimize logistics and reduce costs. For example, identifying consistently late deliveries from a specific supplier might prompt LuminaGlow to explore alternative suppliers or negotiate better delivery terms. This proactive approach minimizes disruption and ensures a steady supply of raw materials.
The Benefits of Descriptive Analytics for LuminaGlow
The implementation of descriptive analytics has yielded significant benefits for LuminaGlow, including:
-
Improved Operational Efficiency: By identifying bottlenecks and inefficiencies in production, LuminaGlow has optimized its manufacturing processes, reduced waste, and increased output.
-
Enhanced Sales and Marketing Effectiveness: Data-driven insights have enabled LuminaGlow to target its marketing efforts more effectively, increasing sales conversions and return on investment (ROI).
-
Increased Customer Satisfaction: Analyzing customer feedback has enabled LuminaGlow to improve product quality and address customer concerns promptly, fostering loyalty and positive brand perception.
-
Optimized Supply Chain Management: Analyzing supply chain data has reduced lead times, minimized disruptions, and optimized transportation costs.
-
Data-Driven Decision Making: Descriptive analytics provides LuminaGlow's management team with the data-backed insights needed to make informed decisions across all aspects of the business.
Conclusion: Shining a Light on Success
Descriptive analytics is not just a tool; it's a strategic imperative for any light bulb manufacturer striving for success in a competitive market. For LuminaGlow, and other manufacturers like it, the ability to effectively analyze historical data provides a clear path to improved operational efficiency, enhanced customer satisfaction, and ultimately, greater profitability. By illuminating the path with data-driven insights, LuminaGlow shines brighter than ever before. The use of descriptive analytics is not a one-time implementation; it’s an ongoing process of continuous improvement, adapting to market changes and refining strategies based on the ever-evolving data landscape. The future of light bulb manufacturing, like the future of any successful business, is illuminated by the power of data.
Latest Posts
Latest Posts
-
Consider The Stereochemistry Of The Compound
May 08, 2025
-
Which One Of The Worldview Elements Listed Below
May 08, 2025
-
A 1000 Bond Trading At 102 5 Means That
May 08, 2025
-
Unit 2 Linear Functions Homework Answers
May 08, 2025
-
Which Of The Following Is True Of Globular Transfer
May 08, 2025
Related Post
Thank you for visiting our website which covers about A Light Bulb Manufacturer Uses Descriptive Analytics . We hope the information provided has been useful to you. Feel free to contact us if you have any questions or need further assistance. See you next time and don't miss to bookmark.