A Representative Sample Is One That Accurately Reflects A Larger
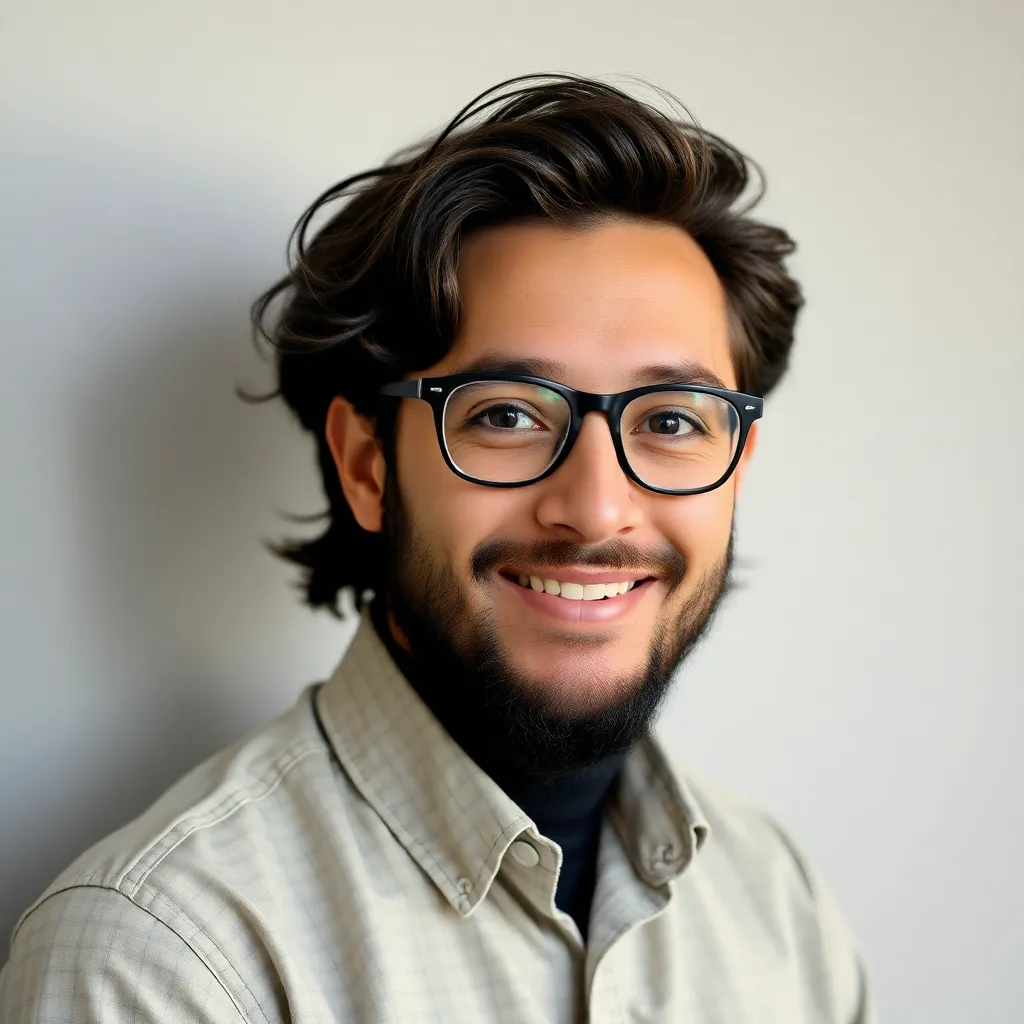
Onlines
May 08, 2025 · 6 min read
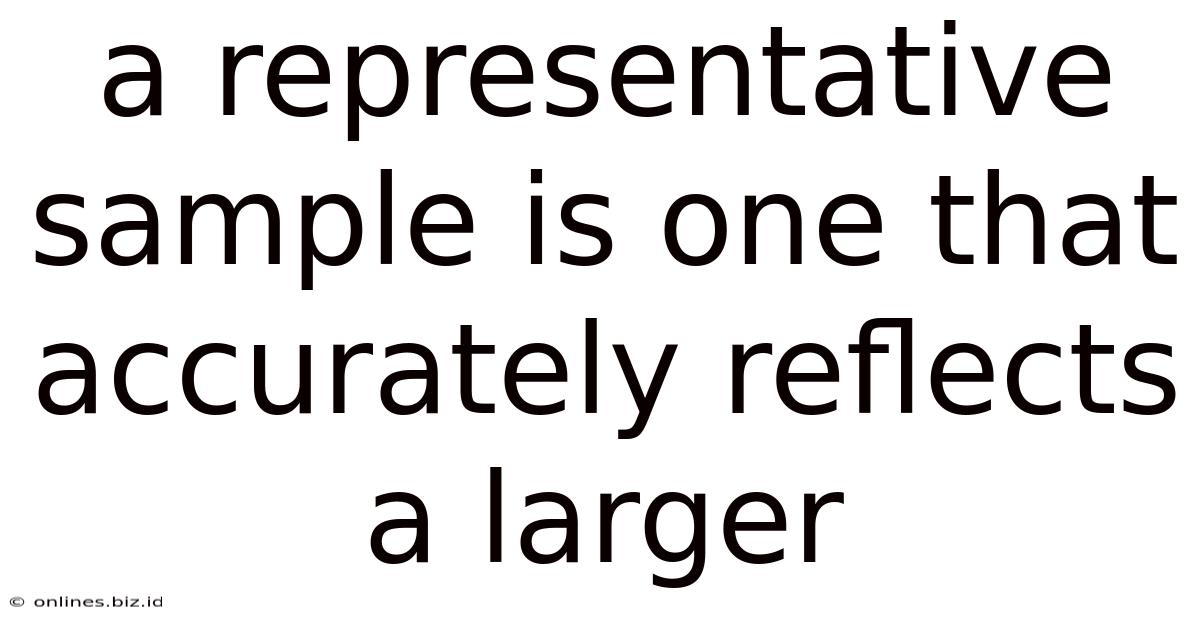
Table of Contents
A Representative Sample: Accurately Reflecting a Larger Population
Understanding how to obtain a truly representative sample is crucial for any research project aiming to draw meaningful conclusions about a larger population. A representative sample is a subset of a population that accurately reflects the characteristics of the entire group. Getting this right is paramount; a flawed sample can lead to inaccurate, misleading, and even harmful conclusions. This article delves into the complexities of representative sampling, exploring various methods, potential pitfalls, and best practices to ensure your research yields reliable results.
The Importance of Representativeness
The primary goal of sampling is to make inferences about a population based on the data collected from a smaller subset. If the sample is not representative, the inferences drawn will be biased and unreliable. Imagine trying to understand the voting preferences of a country by only surveying people in a single city – the results would be skewed and not reflective of the national sentiment.
Why is representativeness so crucial?
- Accuracy: Representative samples minimize sampling error, ensuring your findings are closer to the true population values.
- Generalizability: Results from a representative sample can be generalized to the broader population with greater confidence.
- Validity: A representative sample enhances the internal and external validity of your research, strengthening its credibility.
- Resource Efficiency: Studying a representative sample is significantly more efficient and cost-effective than studying an entire population.
Methods for Achieving Representativeness
Several methods aim to create representative samples. The choice depends heavily on the research question, the nature of the population, and available resources.
1. Probability Sampling
Probability sampling methods ensure that every member of the population has a known, non-zero chance of being selected. This significantly reduces the risk of bias. Key probability sampling techniques include:
-
Simple Random Sampling: Each member of the population has an equal chance of selection. This is often achieved using random number generators. While straightforward, it can be impractical for large populations.
-
Stratified Random Sampling: The population is divided into strata (subgroups) based on relevant characteristics (e.g., age, gender, location). A random sample is then drawn from each stratum, proportionally representing the stratum's size in the population. This ensures representation across different subgroups.
-
Cluster Sampling: The population is divided into clusters (e.g., geographical areas, schools). A random sample of clusters is selected, and then all or a subset of individuals within those selected clusters are surveyed. This is efficient for geographically dispersed populations but can lead to higher sampling error than stratified sampling.
-
Systematic Sampling: Every kth member of the population is selected after a random starting point. This is simple to implement but requires a well-ordered population list and can be problematic if there's a pattern in the list that coincides with k.
2. Non-Probability Sampling
Non-probability sampling methods do not guarantee that every member of the population has a chance of being selected. While easier and often cheaper, they increase the risk of bias and limit the generalizability of findings. These methods include:
-
Convenience Sampling: Selecting participants readily available (e.g., surveying shoppers at a mall). This is highly susceptible to bias and should be avoided whenever possible in serious research.
-
Quota Sampling: Similar to stratified sampling, but the selection within each stratum is non-random. Researchers select participants until they meet pre-determined quotas for each stratum. While it aims for representation, the non-random selection introduces bias.
-
Snowball Sampling: Participants are asked to recommend other potential participants. Useful for studying hard-to-reach populations, but it risks over-representing certain subgroups and under-representing others.
-
Purposive Sampling: Researchers handpick participants based on their knowledge of the population and the research question. This is often used in qualitative research but lacks the generalizability of probability sampling.
Factors Affecting Representativeness
Several factors can compromise the representativeness of a sample, even when using probability sampling methods:
-
Sampling Frame Error: The sampling frame is the list from which the sample is drawn. If the sampling frame is incomplete or inaccurate (e.g., outdated phone directories), the sample will not accurately represent the population.
-
Non-response Bias: When a significant portion of selected participants fail to respond, it can skew the results. Non-respondents may differ systematically from respondents, introducing bias. Strategies to minimize non-response bias include follow-up contacts, incentives, and careful questionnaire design.
-
Coverage Error: Occurs when the sampling frame doesn't cover the entire population of interest. For example, excluding certain demographic groups from the frame.
-
Measurement Error: Errors in data collection, such as poorly worded questions or interviewer bias, can affect the accuracy of the results and reduce representativeness.
-
Sampling Error: Even with a well-designed probability sample, some level of sampling error is expected due to chance variation. Larger sample sizes generally reduce sampling error.
Best Practices for Ensuring Representativeness
To maximize the chances of obtaining a truly representative sample, consider these best practices:
-
Define the Population Clearly: Before starting, carefully define the population of interest. Be specific about inclusion and exclusion criteria.
-
Choose the Appropriate Sampling Method: Select the sampling method best suited to your research question, resources, and population characteristics.
-
Develop a Comprehensive Sampling Frame: Ensure the sampling frame is up-to-date, accurate, and complete.
-
Maximize Response Rates: Implement strategies to encourage participation and minimize non-response bias.
-
Pilot Test Your Instruments: Test your data collection instruments (e.g., questionnaires) on a small pilot sample to identify and rectify potential problems before the main study.
-
Analyze Data for Bias: After data collection, carefully analyze the data for potential biases. Consider using weighting techniques to adjust for any known imbalances in the sample.
-
Report Limitations: Acknowledge any limitations of your sampling method and potential sources of bias in your research report. Transparency is crucial for maintaining the integrity of your findings.
The Role of Sample Size
The sample size significantly impacts the representativeness of a sample. A larger sample size generally leads to a more precise estimate of population parameters and reduces sampling error. However, increasing sample size comes with increased costs and effort. Determining the appropriate sample size involves considering:
- Population Size: Larger populations require larger samples.
- Desired Precision: Higher precision demands larger samples.
- Expected Variability: Greater variability within the population requires larger samples.
- Confidence Level: Higher confidence levels (e.g., 99% vs. 95%) require larger samples.
Statistical power analysis can be used to determine the optimal sample size for a given research design. This involves specifying the desired power, significance level, and effect size to estimate the minimum sample size needed to detect a statistically significant effect.
Conclusion: The Pursuit of Accuracy
Obtaining a representative sample is a critical step in any research endeavor. By understanding the various sampling methods, potential biases, and best practices discussed in this article, researchers can significantly improve the accuracy and generalizability of their findings. Remember that the pursuit of a truly representative sample is an iterative process, requiring careful planning, rigorous execution, and a critical evaluation of potential limitations. While perfect representation is rarely attainable, striving for it is essential for producing credible and impactful research. The effort invested in achieving a representative sample is a direct investment in the validity and reliability of your conclusions, ensuring your research contributes meaningfully to the body of knowledge.
Latest Posts
Latest Posts
-
Amarillo Company Experienced The Following Events
May 08, 2025
-
Which Of The Following Statements About Free Radicals Is True
May 08, 2025
-
Compute Reaction Rates For All Seven Trials
May 08, 2025
-
Why Is Food Science Called An Integrated Course
May 08, 2025
-
Which Of The Following Is True Regarding Audience
May 08, 2025
Related Post
Thank you for visiting our website which covers about A Representative Sample Is One That Accurately Reflects A Larger . We hope the information provided has been useful to you. Feel free to contact us if you have any questions or need further assistance. See you next time and don't miss to bookmark.