A Restaurant Manager Collected Data To Predict Monthly Sales
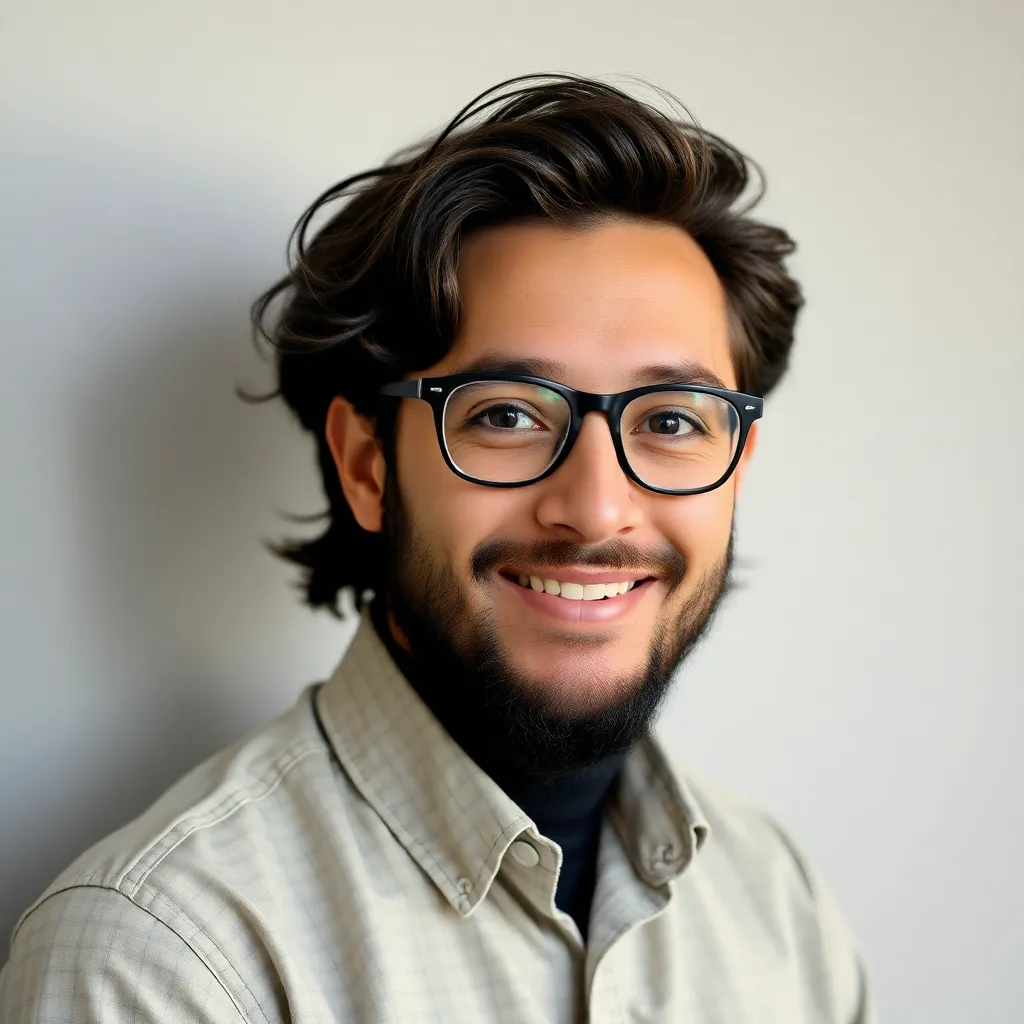
Onlines
Apr 19, 2025 · 6 min read

Table of Contents
A Restaurant Manager's Guide to Predicting Monthly Sales Using Data
Predicting monthly sales is crucial for any restaurant's success. Accurate forecasting allows for better inventory management, optimized staffing levels, and informed marketing strategies. Instead of relying on gut feeling, a restaurant manager can leverage data analysis to create a robust sales prediction model. This article delves into the process, from data collection to model interpretation, equipping restaurant managers with the tools to enhance their forecasting accuracy.
The Power of Data: What to Collect and Why
Before any prediction can be made, comprehensive data collection is paramount. The following data points are essential for creating an effective predictive model:
1. Historical Sales Data:
- Daily/Weekly Sales: This forms the foundation of your predictive model. Record total revenue, broken down by day of the week and time of day. Identifying peak hours and days can significantly improve scheduling and resource allocation.
- Sales by Menu Item: Tracking individual item sales provides insights into popular dishes and less profitable options. This informs menu engineering decisions, highlighting opportunities for upselling and cross-selling.
- Sales by Payment Type: Analyzing sales based on cash, credit card, and other payment methods can inform marketing strategies and assist in financial forecasting.
2. External Factors:
- Seasonality: Restaurant sales are often highly seasonal. Consider holidays, weather patterns, and school breaks, as these factors can dramatically impact customer traffic and sales volume.
- Local Events: Major events such as concerts, festivals, or sporting events in the vicinity can significantly influence sales. Note down dates and expected customer influx.
- Economic Indicators: Track local economic trends. Changes in unemployment rates or consumer confidence can influence spending habits and restaurant revenue.
- Marketing Campaigns: Document the timing and success of your marketing initiatives. Analyze the impact of promotions, social media campaigns, or loyalty programs on sales figures. This data will help you to measure ROI (Return on Investment).
- Competitor Analysis: Monitor your competitors' activities. New restaurant openings, price changes, or promotional campaigns can all affect your sales.
3. Operational Data:
- Staffing Levels: Correlate sales figures with the number of staff members on duty. This helps optimize staffing levels based on predicted sales volume, avoiding overstaffing or understaffing.
- Inventory Levels: Monitor inventory costs and sales figures to pinpoint potential stock-outs or overstocking of ingredients. This is crucial for minimizing waste and optimizing procurement.
- Customer Feedback: Track customer satisfaction through surveys, online reviews, or feedback forms. Negative reviews can highlight areas for improvement that could directly impact future sales.
Data Analysis and Model Selection: Turning Data into Insights
Once you've collected the necessary data, the next step is to analyze it and choose an appropriate predictive model. Several methods can be employed, each with its strengths and weaknesses:
1. Simple Moving Average:
This is a straightforward method that averages sales figures over a specific period. For example, a 3-month moving average takes the average sales for the past three months to predict the next month's sales. It's simple to implement but doesn't account for seasonality or trends.
- Pros: Easy to understand and calculate.
- Cons: Ignores trends and seasonality; less accurate for volatile sales data.
2. Weighted Moving Average:
This method assigns different weights to past sales figures, giving more importance to recent data. For instance, the most recent month might have a weight of 0.4, the previous month 0.3, and the month before that 0.3. This enhances accuracy by emphasizing recent trends.
- Pros: More responsive to recent trends than the simple moving average.
- Cons: Still doesn't account for seasonality or external factors.
3. Exponential Smoothing:
This technique assigns exponentially decreasing weights to older data. It's particularly useful for data with trends and seasonality. Different variations exist, such as single exponential smoothing (for data with trends) and double exponential smoothing (for data with trends and seasonality).
- Pros: Accounts for trends and seasonality; relatively simple to implement.
- Cons: Can be sensitive to parameter choices.
4. Regression Analysis:
This statistical method helps to identify the relationship between sales and other variables (independent variables). For example, you might use regression analysis to determine the relationship between sales and temperature, day of the week, or marketing spending.
- Pros: Allows for the inclusion of multiple independent variables; identifies the strength of relationships between variables.
- Cons: Requires a good understanding of statistical concepts; can be complex to implement.
5. Time Series Analysis:
This sophisticated technique is specifically designed for forecasting time-dependent data. It uses statistical models like ARIMA (Autoregressive Integrated Moving Average) to identify patterns and trends in historical sales data.
- Pros: Highly accurate for complex time series data; accounts for seasonality and trends.
- Cons: Requires expertise in statistical modeling; can be computationally intensive.
Implementing and Refining Your Model
Once you’ve selected a model, the implementation process involves:
- Data Cleaning: Ensure your data is accurate, complete, and consistent. Handle missing values appropriately.
- Model Training: Use historical data to train your chosen model. This involves feeding the model with your collected data so it can learn the patterns and relationships.
- Model Validation: Test the model's accuracy using a separate set of data (data not used for training). This helps evaluate how well the model generalizes to new data. Common metrics include Mean Absolute Error (MAE), Root Mean Squared Error (RMSE), and R-squared.
- Model Refinement: Based on the validation results, fine-tune the model's parameters or consider alternative models to improve accuracy. This iterative process is key to achieving optimal performance.
Integrating Predictions into Restaurant Operations
Accurate sales predictions should be integrated into various aspects of restaurant management:
- Inventory Management: Optimize inventory levels based on predicted demand, minimizing waste and ensuring sufficient stock.
- Staff Scheduling: Adjust staffing levels based on predicted customer traffic, optimizing labor costs and ensuring adequate service.
- Marketing Strategies: Develop targeted marketing campaigns based on seasonal trends and predicted demand.
- Financial Planning: Use sales predictions to create accurate budgets and financial forecasts.
Beyond the Numbers: Qualitative Factors
While quantitative data is crucial, remember that qualitative factors can significantly impact sales. These include:
- Customer Service: Excellent service fosters loyalty and positive word-of-mouth marketing, influencing future sales.
- Menu Innovation: Regularly updating your menu with new and exciting dishes can attract new customers and boost sales.
- Location and Ambiance: The restaurant's location and ambiance play a significant role in attracting customers.
- Reputation and Reviews: Positive online reviews and a strong reputation can significantly impact sales.
Continuous Monitoring and Adaptation
Predictive modeling isn't a one-time task. Continuously monitor your model's performance and update it regularly. As new data becomes available and external factors change, adjustments are crucial to maintain accuracy. Regularly review your data collection process to ensure its completeness and accuracy. Consider incorporating new data points as needed.
By embracing data-driven decision-making, restaurant managers can transform their forecasting from guesswork into a powerful tool for improving profitability and sustainability. Remember that while the technical aspects are important, understanding your customer base and adapting to changing market conditions are equally crucial to success. Combine the power of data analysis with astute business acumen, and you’ll be well-equipped to steer your restaurant toward consistent growth and success.
Latest Posts
Latest Posts
-
What Ethical Ideologies Were Formed In Isolation
Apr 19, 2025
-
Which Two Parts Highlight The Psychological Consequences Of War
Apr 19, 2025
-
9 1 Skills Practice Graphing Quadratic Functions Answer Key
Apr 19, 2025
-
Summary Of The Giver Chapter 16
Apr 19, 2025
-
What Should Developers Do If The Product Owner Is Unavailable
Apr 19, 2025
Related Post
Thank you for visiting our website which covers about A Restaurant Manager Collected Data To Predict Monthly Sales . We hope the information provided has been useful to you. Feel free to contact us if you have any questions or need further assistance. See you next time and don't miss to bookmark.