A Significant Weakness Of The Ordinal Scale Is
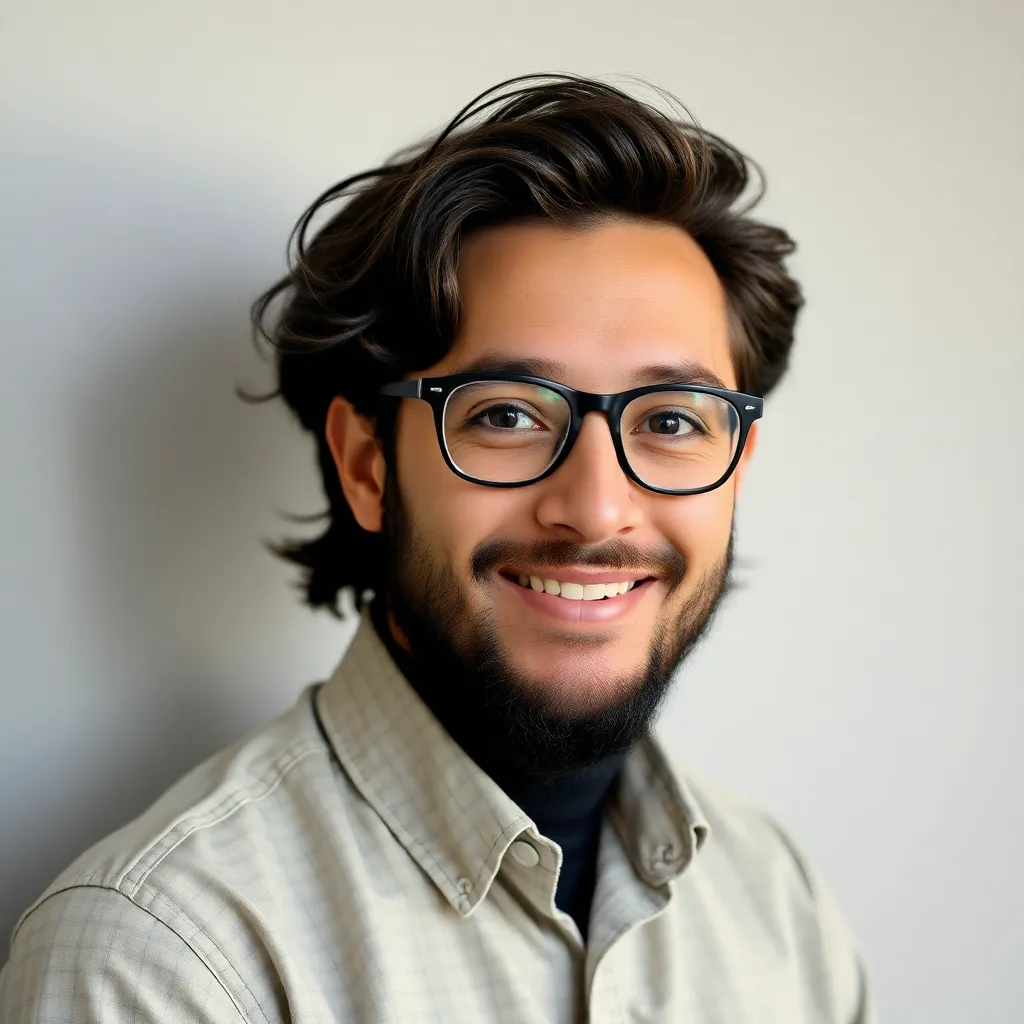
Onlines
May 12, 2025 · 6 min read
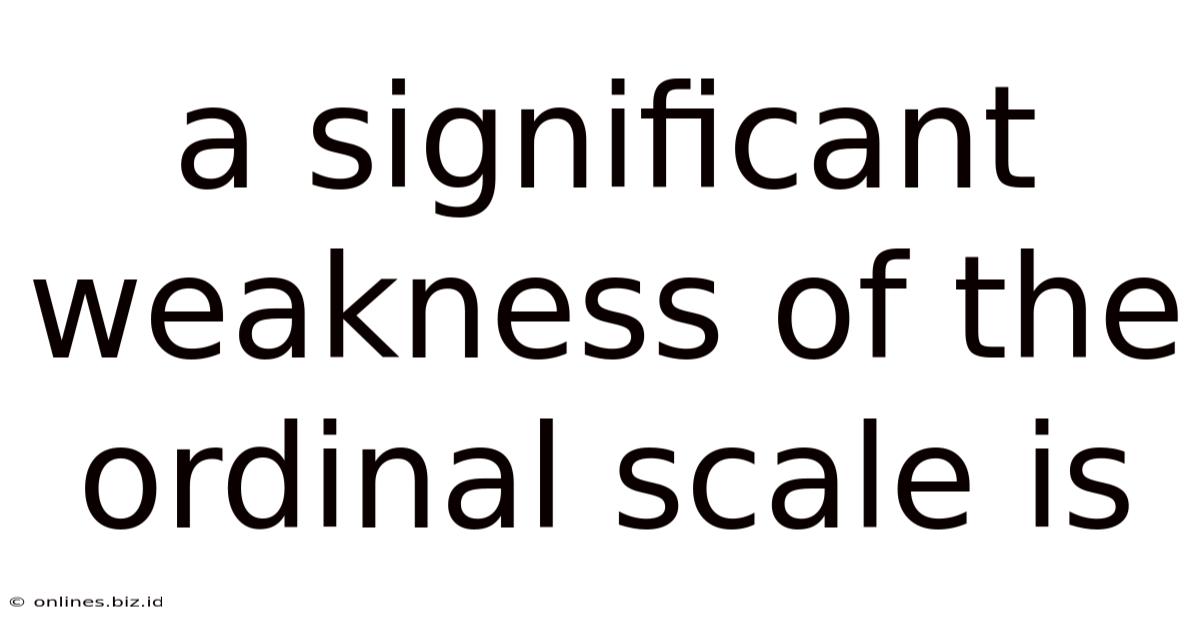
Table of Contents
A Significant Weakness of the Ordinal Scale: Lack of Equal Intervals and the Limitations of Statistical Analysis
The ordinal scale, a common type of measurement in social sciences and market research, ranks data according to order or preference. While offering a valuable step beyond nominal data (which simply categorizes data without order), the ordinal scale possesses a crucial weakness: the lack of equal intervals between ranks. This limitation significantly restricts the types of statistical analyses that can be meaningfully applied and impacts the interpretations drawn from the data. This article delves deep into this significant weakness, exploring its implications and suggesting alternative approaches when dealing with ordinal data.
Understanding the Ordinal Scale and Its Limitations
Before exploring the limitations, let's briefly recap what an ordinal scale represents. Ordinal data provides information about the relative ranking of observations. For example, consider a survey asking participants to rate their satisfaction with a product on a scale of 1 to 5, where 1 represents "Very Dissatisfied" and 5 represents "Very Satisfied." This is ordinal data because it establishes an order (1 < 2 < 3 < 4 < 5), but the difference between "Very Dissatisfied" (1) and "Dissatisfied" (2) isn't necessarily the same as the difference between "Satisfied" (4) and "Very Satisfied" (5). The intervals are not equal.
This lack of equal intervals is the core weakness of the ordinal scale and fundamentally limits its analytical capabilities. Unlike interval and ratio scales (where the differences between values are meaningful and consistent), the numerical values assigned in ordinal scales are merely labels indicating rank. Therefore, calculating means, standard deviations, or other descriptive statistics relying on equal intervals is misleading and inaccurate.
The Implications of Unequal Intervals
The unequal intervals inherent in ordinal data have several significant implications for researchers and analysts:
1. Misleading Averages:
Calculating the average of ordinal data can produce a meaningless result. For instance, averaging satisfaction ratings (1-5) might yield a value of 3.5, which seems to indicate "moderately satisfied." However, this average is only a numerical average of ranks, not a true reflection of the underlying satisfaction levels. The distance between each rank may be vastly different, rendering the mean statistically meaningless.
2. Limited Statistical Tests:
Many powerful statistical tests, such as t-tests, ANOVA, and Pearson's correlation, require data with equal intervals. Applying these tests to ordinal data violates their underlying assumptions and can lead to incorrect conclusions. The results obtained would be invalid and may not accurately reflect the relationships within the data.
3. Inability to Measure Magnitude of Differences:
Ordinal data only reveals the order; it doesn't quantify the magnitude of the differences between ranks. For example, the difference between "Satisfied" (4) and "Very Satisfied" (5) could be substantial for one respondent but minimal for another. Ordinal scales cannot capture this nuance.
4. Difficulty in Comparing Groups:
While you can compare the distributions of ordinal data across groups using non-parametric tests, interpreting the results can be challenging due to the unequal intervals. The differences observed might not accurately reflect the true differences in the underlying phenomena.
Alternative Approaches and Solutions
Given the limitations of the ordinal scale, researchers often explore alternative approaches to extract meaningful insights from this type of data:
1. Non-parametric Tests:
Non-parametric statistical tests are designed specifically for ordinal data and do not rely on the assumption of equal intervals. Examples include:
- Mann-Whitney U test: Compares the distributions of two independent groups.
- Wilcoxon signed-rank test: Compares the distributions of two related groups.
- Kruskal-Wallis test: Compares the distributions of three or more independent groups.
- Spearman's rank correlation: Measures the monotonic relationship between two ordinal variables.
These tests analyze the ranks themselves rather than the numerical values, providing valid inferences even without equal intervals.
2. Transformation of Data:
In some cases, transforming ordinal data into a different scale might be considered. However, this needs careful consideration and justification. For instance, if the ordinal scale reasonably approximates an interval scale, certain transformations might be applied to improve the analysis. This approach is highly sensitive to the context of the study.
3. Visualizations:
Visualizations, such as bar charts, histograms, and box plots, can provide valuable insights into the distribution of ordinal data without relying on parametric tests. These visual representations can clearly show the frequency of each rank and the overall pattern of responses.
4. Considering Alternative Scales:
If the research question allows, it might be more appropriate to use a different scale, like an interval or ratio scale, which can offer more analytical flexibility. For example, instead of asking for a satisfaction rating on a 1-5 scale, a visual analog scale (VAS) might be used, where participants mark their level of satisfaction on a continuous line. This allows for more granular data and enables the use of parametric statistics.
5. Robust Statistical Methods:
Certain statistical methods are less sensitive to violations of assumptions, including the assumption of equal intervals. These robust methods may provide more reliable results even when applied to ordinal data. However, caution must be exercised when interpreting the results.
Case Studies Illustrating the Weakness
Let's consider a few hypothetical case studies to better understand the implications of using ordinal data:
Case Study 1: Customer Satisfaction Survey:
A company uses a 5-point Likert scale (1-Very Dissatisfied, 5-Very Satisfied) to measure customer satisfaction. They calculate the average satisfaction score. However, because the intervals aren't necessarily equal, a score of 3.5 might not truly represent "moderately satisfied." A non-parametric test, such as the Mann-Whitney U test, would be more appropriate to compare satisfaction levels between different customer segments.
Case Study 2: Movie Rating System:
A movie rating system uses stars (e.g., 1-star to 5-stars). While this provides an ordinal ranking, the difference between a 1-star and 2-star movie might not be the same as the difference between a 4-star and 5-star movie. Comparing average ratings across different genres would be misleading. Analyzing the distribution of ratings using visualizations or applying non-parametric correlation analysis would yield more meaningful results.
Case Study 3: Educational Assessment:
Students are ranked based on their performance on a test (e.g., 1st, 2nd, 3rd, etc.). While this provides an ordinal ranking, the difference in ability between the 1st and 2nd-ranked students isn't necessarily equal to the difference between the 10th and 11th-ranked students. Calculations involving averages or standard deviations would be inappropriate. Instead, comparing the distributions of ranks across different classes using non-parametric tests would be more valid.
Conclusion: A Cautious Approach to Ordinal Data
The ordinal scale is a valuable tool for measuring rank-ordered data; however, researchers must be acutely aware of its fundamental weakness: the absence of equal intervals. This limitation significantly constrains the types of statistical analyses that can be applied and impacts the reliability of conclusions drawn. By choosing appropriate non-parametric tests, employing suitable visualizations, and carefully considering alternative scales or robust methods, researchers can mitigate the issues stemming from the unequal intervals in ordinal data and extract meaningful insights while avoiding misleading interpretations. Always prioritize the selection of analytical methods appropriate to the nature of the data to ensure accurate and reliable findings.
Latest Posts
Latest Posts
-
According To The Chart When Did A Pdsa Cycle Occur
May 12, 2025
-
Bioflix Activity Gas Exchange The Respiratory System
May 12, 2025
-
Economic Value Creation Is Calculated As
May 12, 2025
-
Which Items Typically Stand Out When You Re Scanning Text
May 12, 2025
-
Assume That Price Is An Integer Variable
May 12, 2025
Related Post
Thank you for visiting our website which covers about A Significant Weakness Of The Ordinal Scale Is . We hope the information provided has been useful to you. Feel free to contact us if you have any questions or need further assistance. See you next time and don't miss to bookmark.