According To The Gartner Analytic Ascendancy Model
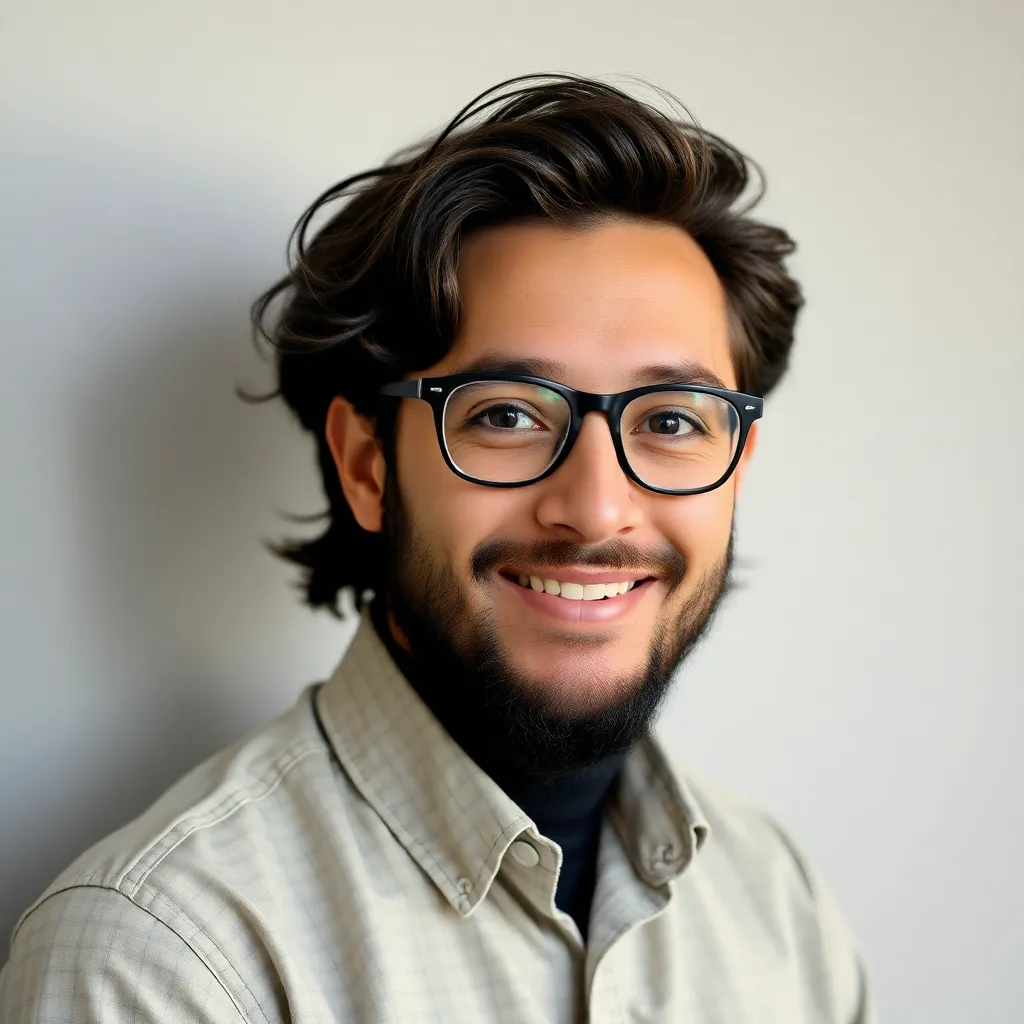
Onlines
May 11, 2025 · 5 min read
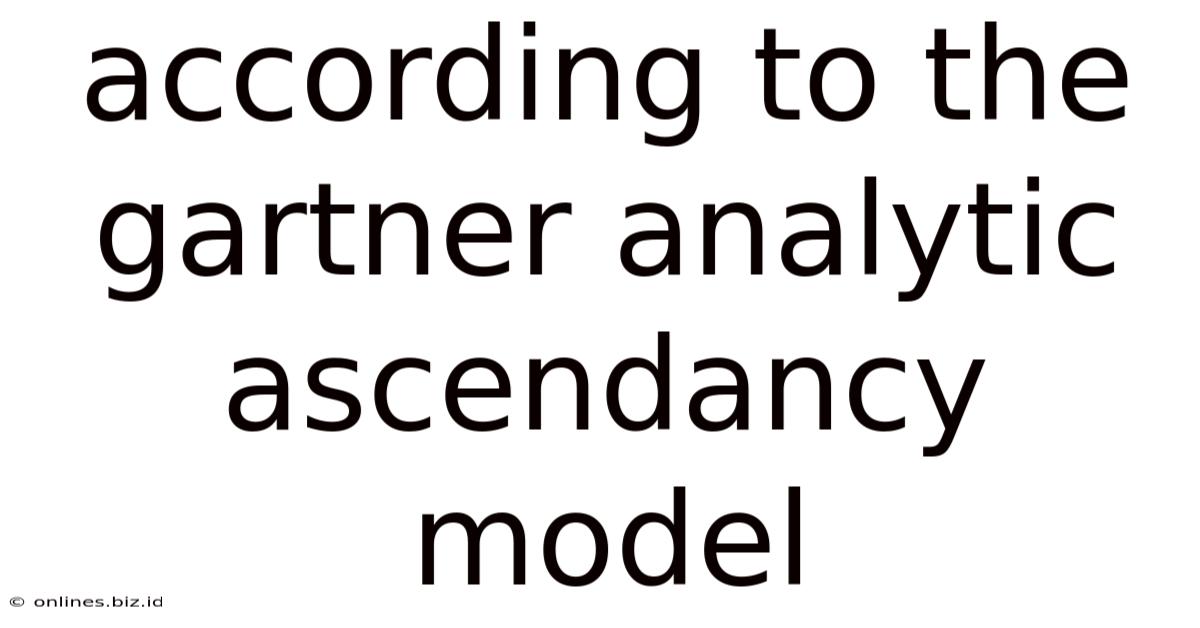
Table of Contents
Gartner's Analytic Ascendancy Model: A Comprehensive Guide to Data-Driven Decision Making
Gartner's Analytic Ascendancy Model provides a framework for organizations to understand and improve their analytical capabilities. It's not just about having data; it's about leveraging data to drive strategic decision-making and achieve a competitive advantage. This model outlines five levels of analytical maturity, each with its own characteristics, challenges, and opportunities. Understanding this model is crucial for organizations looking to unlock the full potential of their data.
The Five Levels of Analytic Ascendancy
Gartner's model depicts a journey, ascending through five distinct levels of analytical capability:
1. Descriptive Analytics: The Foundation of Insight
This foundational level focuses on understanding what happened in the past. Descriptive analytics uses historical data to identify trends, patterns, and anomalies. Think of it as the "what happened" stage. Key techniques include:
- Reporting: Generating standard reports on key performance indicators (KPIs).
- Data Aggregation: Combining data from various sources to create a unified view.
- Data Visualization: Using charts and graphs to present data in a clear and concise manner.
Example: Analyzing sales data to determine the best-selling products over the past year. This provides valuable insight but doesn't predict future performance.
Challenges at this level: Data silos, inconsistent data quality, and a lack of standardized reporting processes. Over-reliance on descriptive analytics without progressing to higher levels can limit an organization's ability to anticipate future trends.
2. Diagnostic Analytics: Unveiling the "Why"
Building upon descriptive analytics, this level delves into why certain events occurred. It moves beyond simply identifying trends to exploring the root causes behind them. Diagnostic analytics utilizes techniques such as:
- Drill-Down Analysis: Investigating data in greater detail to uncover underlying reasons.
- Data Mining: Discovering hidden patterns and relationships within large datasets.
- Correlation Analysis: Identifying relationships between different variables.
Example: Analyzing sales data to understand why certain products underperformed. This could reveal issues with marketing, pricing, or product quality.
Challenges at this level: The need for more sophisticated analytical techniques and the potential for biased interpretations of data. The ability to accurately pinpoint causation can be difficult, requiring careful consideration of confounding variables.
3. Predictive Analytics: Forecasting the Future
This level focuses on anticipating future outcomes by using historical data and statistical modeling. Predictive analytics enables organizations to make proactive decisions rather than simply reacting to events. Key techniques include:
- Regression Analysis: Predicting future values based on historical relationships between variables.
- Machine Learning: Utilizing algorithms to identify patterns and make predictions from complex data.
- Forecasting Models: Developing models to estimate future trends and outcomes.
Example: Forecasting future sales based on historical data, seasonality, and economic indicators. This enables organizations to optimize inventory levels and allocate resources more effectively.
Challenges at this level: The need for accurate and complete data, the selection of appropriate models, and the interpretation of model outputs. Overfitting models to historical data can lead to inaccurate predictions.
4. Prescriptive Analytics: Optimizing Decisions
This advanced level goes beyond prediction to recommend optimal actions. Prescriptive analytics uses optimization techniques to identify the best course of action in a given situation. It involves:
- Simulation: Modeling different scenarios to assess potential outcomes.
- Optimization Algorithms: Identifying the best solution among a range of possibilities.
- Decision Support Systems: Providing tools and insights to aid in decision-making.
Example: Optimizing pricing strategies to maximize revenue while considering customer sensitivity and competitor pricing. This involves sophisticated modeling and simulations to determine the optimal price point.
Challenges at this level: The complexity of developing and implementing prescriptive models, the need for real-time data, and the integration of models into decision-making processes. The computational cost of complex optimization can also be significant.
5. Cognitive Analytics: Augmenting Human Intelligence
This is the pinnacle of the Analytic Ascendancy Model, leveraging artificial intelligence (AI) and machine learning to augment human capabilities. Cognitive analytics enables organizations to address complex problems that are beyond the scope of traditional analytical techniques. Key aspects include:
- Natural Language Processing (NLP): Understanding and interpreting human language.
- Machine Learning (ML): Automatically learning patterns and insights from data.
- Deep Learning (DL): Using artificial neural networks to analyze complex data.
Example: Using AI-powered chatbots to provide personalized customer service, or employing machine learning to detect fraud in real-time. Cognitive analytics enables organizations to automate tasks, gain deeper insights, and improve decision-making across the enterprise.
Challenges at this level: The ethical implications of AI, the need for specialized skills and infrastructure, and the potential for bias in algorithms. Ensuring data privacy and security is also paramount.
Navigating the Ascendancy: Key Considerations
Moving through the levels of the Analytic Ascendancy Model requires a strategic and phased approach. Several key factors contribute to successful ascension:
- Data Quality: High-quality, accurate, and reliable data is the foundation of any analytical initiative. Investing in data governance and management is crucial.
- Technology Infrastructure: The right technology is essential for supporting different levels of analytics. This includes data warehouses, data lakes, and advanced analytics platforms.
- Talent and Skills: Organizations need skilled analysts, data scientists, and data engineers to effectively leverage data. Investing in training and development is vital.
- Culture and Change Management: A data-driven culture requires buy-in from all levels of the organization. Change management processes are crucial for ensuring successful adoption of new analytical techniques.
- Business Alignment: Analytical initiatives should be aligned with business goals and objectives. Defining clear KPIs and metrics is crucial for measuring success.
The Benefits of Analytic Ascendancy
The journey through Gartner's Analytic Ascendancy Model offers significant benefits for organizations, including:
- Improved Decision-Making: Data-driven decisions are more informed, leading to better outcomes.
- Increased Efficiency: Automating processes and optimizing workflows improves operational efficiency.
- Enhanced Customer Experience: Understanding customer needs and preferences enables personalized experiences.
- Competitive Advantage: Leveraging data to anticipate market trends and gain insights ahead of competitors.
- Innovation: Data analytics drives innovation by uncovering new opportunities and insights.
Conclusion: Embarking on the Data-Driven Journey
Gartner's Analytic Ascendancy Model provides a valuable roadmap for organizations seeking to leverage the power of data. While the journey requires investment and commitment, the benefits of achieving a high level of analytical maturity are substantial. By focusing on data quality, technology infrastructure, talent development, and business alignment, organizations can transform themselves into truly data-driven enterprises and achieve sustained competitive advantage in today's dynamic business environment. The key is not to strive for the pinnacle immediately, but to strategically progress through each level, solidifying foundations before tackling more complex analytical challenges. This methodical approach will ensure a sustainable and impactful transformation.
Latest Posts
Related Post
Thank you for visiting our website which covers about According To The Gartner Analytic Ascendancy Model . We hope the information provided has been useful to you. Feel free to contact us if you have any questions or need further assistance. See you next time and don't miss to bookmark.