Accuracy Of Grouped Estimates Depends On
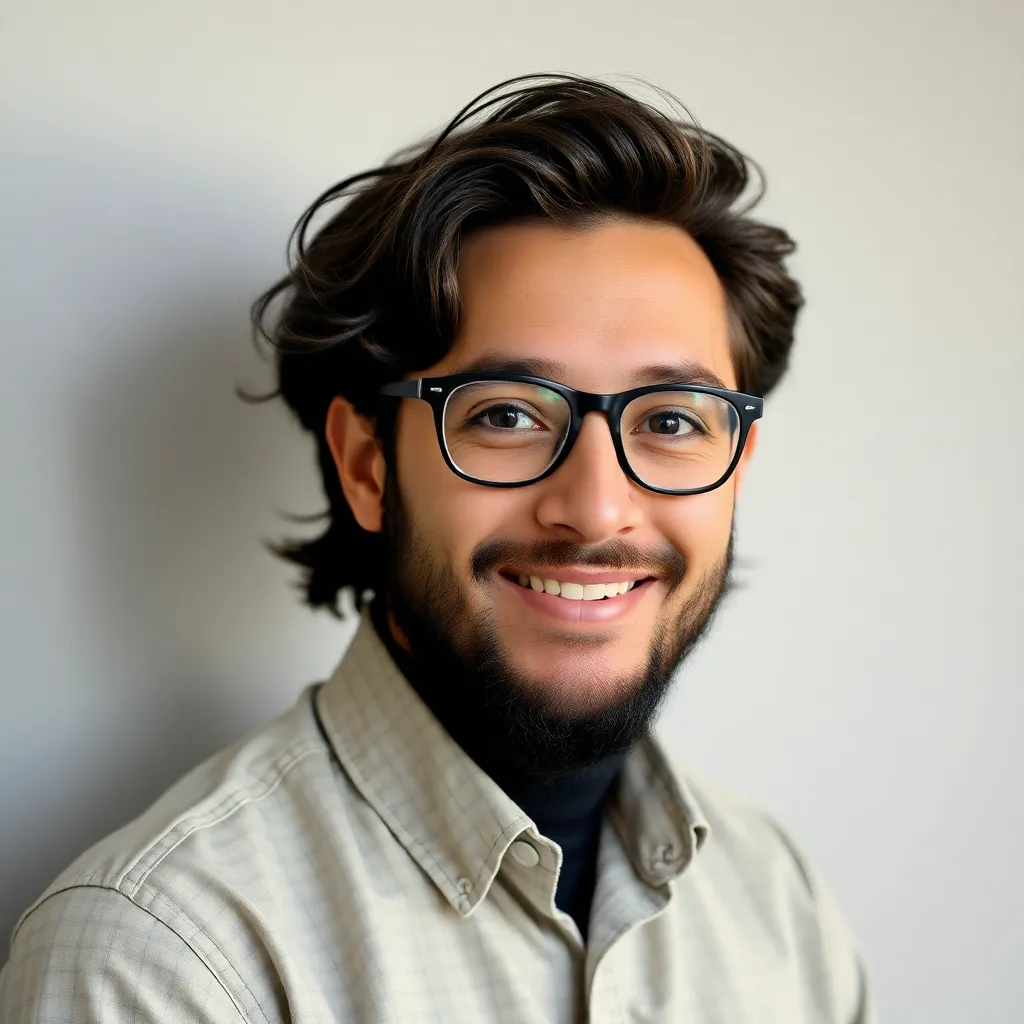
Onlines
Apr 15, 2025 · 6 min read

Table of Contents
The Accuracy of Grouped Estimates: A Deep Dive into Influencing Factors
The accuracy of grouped estimates is crucial in numerous fields, from statistical analysis and data science to business forecasting and public policy. Understanding the factors that influence this accuracy is paramount for drawing reliable conclusions and making informed decisions. This article delves deep into the complexities of grouped estimates, exploring the various factors that can impact their precision and providing insights into how to improve their reliability.
Understanding Grouped Estimates
Before examining the factors affecting accuracy, it's essential to define what grouped estimates are. In essence, a grouped estimate is a statistical summary calculated from data that has been organized into groups or categories. This grouping can be based on various criteria, such as age, income, location, or any other relevant variable. Instead of analyzing individual data points, grouped estimates provide an overview of the characteristics of each group. Examples include:
- Average income by age group: Calculating the mean income for different age brackets (e.g., 18-25, 26-35, etc.).
- Disease prevalence by region: Determining the percentage of the population affected by a specific disease in various geographical regions.
- Customer satisfaction scores by product type: Averaging customer satisfaction ratings for different product categories.
The accuracy of these grouped estimates is not merely a matter of technical precision; it directly impacts the validity of interpretations and the effectiveness of subsequent actions based on those interpretations.
Factors Affecting the Accuracy of Grouped Estimates
Several factors significantly influence the accuracy of grouped estimates. These factors can be broadly categorized into:
1. Data Quality: The Foundation of Accuracy
The accuracy of any statistical analysis, including grouped estimates, fundamentally depends on the quality of the underlying data. Poor data quality can lead to inaccurate and misleading results, regardless of the sophistication of the statistical methods employed. Key aspects of data quality impacting accuracy include:
-
Data Completeness: Missing data can bias estimates, particularly if the missingness is not random. For instance, if higher-income individuals are less likely to participate in a survey, the average income estimate for the entire population will be underestimated. Methods like imputation can be used to address missing data, but they introduce their own complexities and potential biases.
-
Data Accuracy: Errors in data collection, recording, or entry can significantly distort grouped estimates. Simple typos or inconsistencies in data formatting can have cascading effects, leading to erroneous conclusions. Rigorous data validation and cleaning processes are crucial to minimize such errors.
-
Data Representativeness: The accuracy of grouped estimates relies heavily on the representativeness of the sample used. If the sample doesn't accurately reflect the characteristics of the population of interest, the grouped estimates will be biased. Stratified sampling techniques, where the sample is proportionally representative of different subgroups, are essential for achieving better representativeness.
2. Grouping Methodology: The Art of Categorization
The way data is grouped significantly affects the accuracy and interpretability of the results. Poorly chosen grouping variables or inappropriate grouping intervals can obscure important patterns or lead to misleading conclusions.
-
Choice of Grouping Variable: The selection of the grouping variable should be based on a clear understanding of the research question and the relationships between variables. Using an irrelevant grouping variable will not yield meaningful results.
-
Number of Groups: Too few groups can mask important variations within the data, while too many groups can lead to unstable estimates with large variances, particularly if some groups have small sample sizes. Finding the optimal number of groups often involves a trade-off between detail and stability. Techniques like hierarchical clustering can assist in finding appropriate groupings.
-
Group Boundaries: Arbitrary group boundaries can lead to artificial discontinuities and affect the interpretation of results. Consider using natural breaks or percentiles to define group boundaries whenever possible.
3. Statistical Methods: Choosing the Right Tools
The choice of statistical method used to calculate grouped estimates also affects accuracy. Different methods have different assumptions and sensitivities to data characteristics.
-
Measures of Central Tendency: The choice between the mean, median, or mode depends on the data's distribution and the research question. The mean is sensitive to outliers, while the median is more robust.
-
Measures of Dispersion: Understanding the variability within each group is crucial. Measures like standard deviation, interquartile range, or variance provide insights into the spread of data within each group and the reliability of the central tendency measure.
-
Dealing with Outliers: Outliers can disproportionately influence the accuracy of grouped estimates, especially when using the mean. Robust statistical methods are crucial in mitigating the impact of outliers.
4. Sample Size: The Power of Numbers
The accuracy of grouped estimates increases with the sample size. Larger samples provide more precise estimates and reduce the impact of sampling variability. However, even with large sample sizes, the other factors mentioned above can still affect accuracy.
-
Sufficient Sample Size per Group: It's equally critical to ensure an adequate sample size within each group. Groups with small sample sizes can lead to unstable and unreliable estimates. This often necessitates adjusting the grouping strategy to ensure sufficient data points in each category.
-
Power Analysis: Before data collection, performing a power analysis can determine the minimum sample size required to detect meaningful effects with a desired level of confidence.
5. External Factors: Context Matters
External factors beyond the data and methodology can also impact the accuracy of grouped estimates.
-
Changes in the Environment: Grouped estimates based on historical data may become inaccurate if the underlying conditions change over time. For instance, economic forecasts based on past trends may be inaccurate if significant economic shifts occur.
-
Measurement Error: Errors in the measurement instruments or procedures can lead to inaccurate data and, consequently, inaccurate grouped estimates. Careful instrument calibration and rigorous testing are essential to minimize measurement error.
-
Bias in Data Collection: Systematic biases in the data collection process, such as selection bias or response bias, can significantly affect the accuracy of grouped estimates. Careful planning and implementation of the data collection process are crucial to minimize such biases.
Improving the Accuracy of Grouped Estimates
Improving the accuracy of grouped estimates requires a multifaceted approach that addresses all the factors discussed above. Here are some key strategies:
-
Invest in Data Quality: Prioritize data quality throughout the entire data lifecycle, from collection and cleaning to validation and storage.
-
Refine Grouping Strategies: Carefully consider the grouping variables, the number of groups, and the group boundaries to ensure meaningful and interpretable results.
-
Employ Robust Statistical Methods: Choose appropriate statistical methods based on the data characteristics and the research question. Consider using robust methods that are less sensitive to outliers and data violations.
-
Increase Sample Size: Whenever feasible, strive for larger sample sizes to reduce sampling variability and improve the precision of the estimates. Ensure sufficient sample sizes within each group.
-
Address External Factors: Be mindful of external factors that could affect the accuracy of the estimates. Consider adjusting the analysis or incorporating additional information to account for these external influences.
Conclusion: Accuracy is Paramount
The accuracy of grouped estimates is not just a technical detail; it's the cornerstone of reliable conclusions and effective decision-making. Understanding the factors that can influence accuracy is crucial for anyone working with grouped data. By paying meticulous attention to data quality, employing appropriate methodologies, and considering potential biases, we can significantly improve the reliability and validity of our grouped estimates, leading to more insightful analyses and informed actions. Remember that achieving accurate grouped estimates is an iterative process involving careful planning, rigorous execution, and critical evaluation.
Latest Posts
Latest Posts
-
Which Benefit Is Provided By Milieu Therapy
Apr 16, 2025
-
Let Us Now Praise Famous Men Summary
Apr 16, 2025
-
Which Of The Following Best Describes The Columbian Exchange
Apr 16, 2025
-
Prior To Starting Each Maneuver Pilots Should
Apr 16, 2025
-
Centered Space Is Best Described As
Apr 16, 2025
Related Post
Thank you for visiting our website which covers about Accuracy Of Grouped Estimates Depends On . We hope the information provided has been useful to you. Feel free to contact us if you have any questions or need further assistance. See you next time and don't miss to bookmark.