After Researchers Have Gathered Data What Do They Do Next
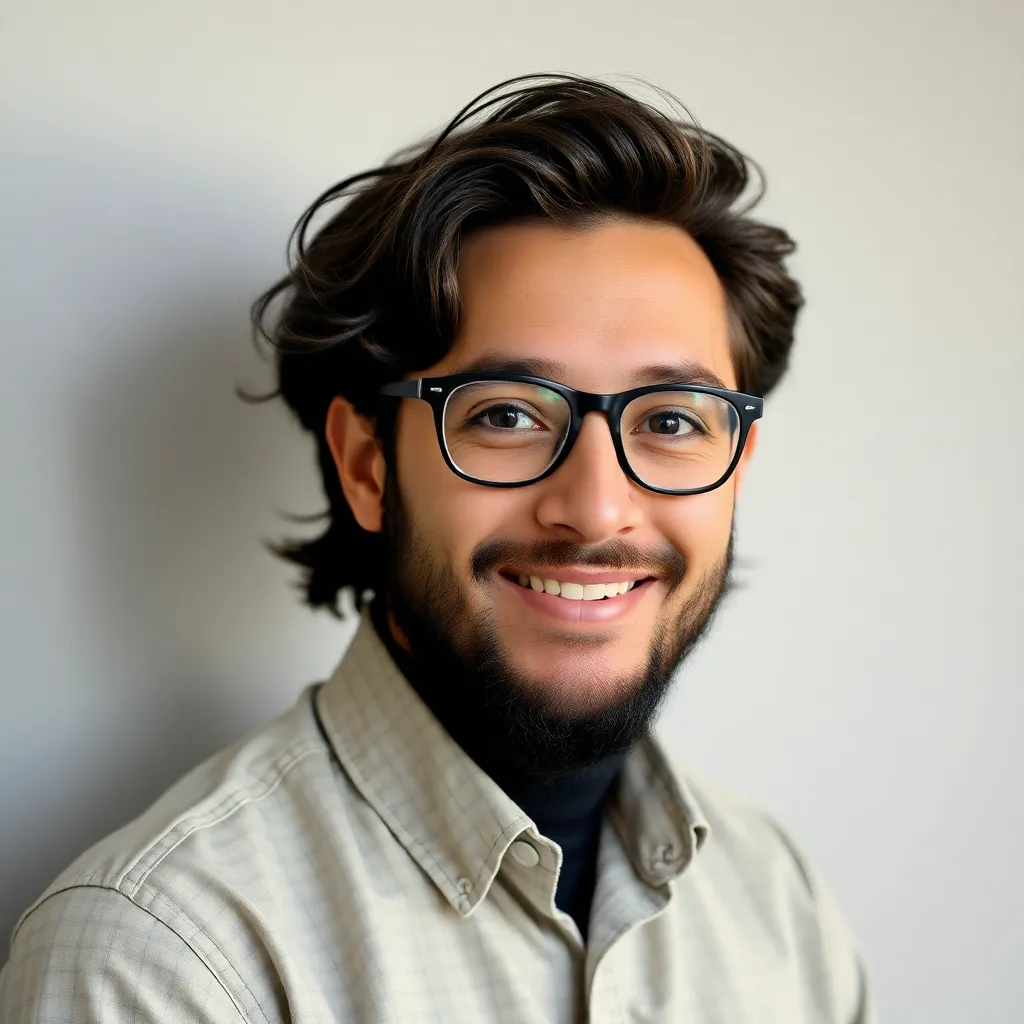
Onlines
May 11, 2025 · 6 min read
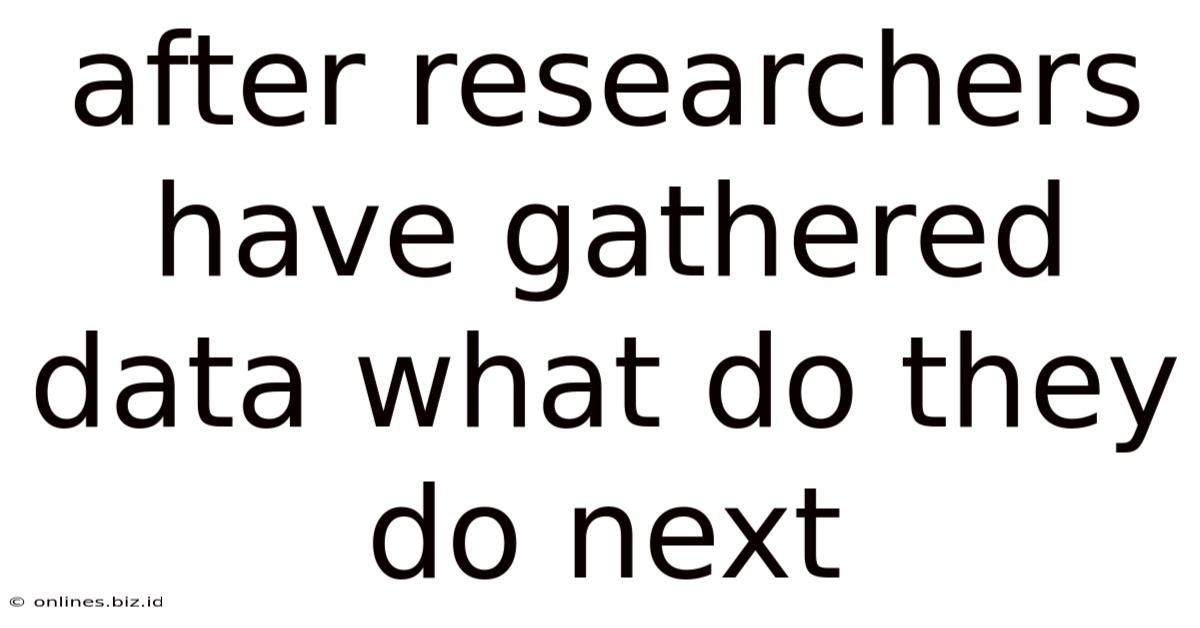
Table of Contents
After Researchers Have Gathered Data: What Happens Next?
So, your research team has diligently collected a mountain of data. Congratulations! But the journey is far from over. Gathering data is only the first step in the research process. What happens next is a critical phase that determines the validity and impact of your findings. This process involves several crucial stages, each demanding careful consideration and meticulous execution. This comprehensive guide outlines the key steps researchers take after data collection, offering insights into the intricate world of data analysis and interpretation.
1. Data Cleaning and Preparation: Laying the Foundation for Accurate Analysis
Before diving into analysis, the data undergoes a crucial process: cleaning and preparation. This often overlooked step is vital for ensuring the accuracy and reliability of your results. Think of it as preparing the ingredients before you start cooking – you wouldn't start making a cake with spoiled eggs!
1.1 Identifying and Handling Missing Data:
Missing data is a common problem in research. Researchers must decide how to deal with it. Common techniques include:
- Deletion: Removing data points with missing values (listwise deletion). This is simple but can lead to a loss of valuable information, especially with large amounts of missing data.
- Imputation: Replacing missing values with estimated values. Methods include mean imputation, regression imputation, and more sophisticated techniques like multiple imputation. Careful consideration is crucial as improper imputation can introduce bias.
- Analysis of Missing Data Patterns: Understanding why data is missing can be just as important as dealing with the missing data itself. Missing data patterns can reveal underlying issues with the study design or data collection methods.
1.2 Identifying and Handling Outliers:
Outliers are data points that significantly deviate from the rest of the data. They can skew results and distort interpretations. Researchers must carefully assess outliers:
- Identifying Outliers: Using statistical methods like box plots or Z-scores can help detect outliers.
- Addressing Outliers: Options include removing outliers, transforming the data (e.g., using logarithmic transformations), or using robust statistical methods less sensitive to outliers. The choice depends on the context and potential reasons for the outliers.
1.3 Data Transformation:
Raw data may not be in a suitable format for analysis. Transformations are often necessary to improve the data's suitability for specific statistical techniques:
- Scaling: Adjusting the range of data values (e.g., standardizing or normalizing). This is common when comparing variables with different scales.
- Coding: Converting categorical variables into numerical representations suitable for statistical analysis (e.g., dummy coding).
- Data aggregation: Combining data from multiple sources or at different levels of analysis (e.g., aggregating individual-level data to the group level).
2. Data Exploration and Descriptive Statistics: Unveiling Patterns and Insights
Once the data is cleaned and prepared, the next step involves exploring it. This phase focuses on understanding the basic characteristics of the data and identifying potential patterns.
2.1 Summary Statistics:
Researchers calculate descriptive statistics to summarize the data:
- Measures of central tendency: Mean, median, and mode provide insights into the typical values in the dataset.
- Measures of dispersion: Standard deviation, variance, and range describe the spread of the data.
- Frequencies and distributions: Histograms, bar charts, and frequency tables illustrate the distribution of variables.
2.2 Visualization:
Visualizing data is crucial for identifying patterns and potential relationships. Common methods include:
- Scatter plots: Show the relationship between two continuous variables.
- Histograms: Display the distribution of a single continuous variable.
- Bar charts: Show the frequencies or means of categorical variables.
- Box plots: Illustrate the distribution of a variable, highlighting median, quartiles, and outliers.
2.3 Correlation Analysis:
Correlation analysis assesses the strength and direction of linear relationships between variables. This helps identify potential associations before conducting more complex analyses.
3. Inferential Statistics: Testing Hypotheses and Drawing Conclusions
This stage involves using statistical methods to draw inferences about the population based on the sample data. This is where researchers test their hypotheses and attempt to answer their research questions.
3.1 Hypothesis Testing:
Researchers formulate hypotheses (null and alternative) and use statistical tests to determine whether there is sufficient evidence to reject the null hypothesis. The choice of test depends on the type of data and the research question. Common tests include:
- t-tests: Compare the means of two groups.
- ANOVA (Analysis of Variance): Compares the means of three or more groups.
- Chi-square tests: Assess the association between categorical variables.
- Regression analysis: Examines the relationship between a dependent variable and one or more independent variables.
3.2 Choosing the Appropriate Statistical Test:
Selecting the correct statistical test is crucial for obtaining valid results. Factors to consider include:
- Type of data: Continuous, categorical, ordinal.
- Number of groups: Two groups or more.
- Research question: Comparing means, assessing associations, predicting outcomes.
3.3 Interpreting p-values and Effect Sizes:
- p-values: Indicate the probability of observing the obtained results if the null hypothesis were true. A low p-value (typically less than 0.05) provides evidence against the null hypothesis.
- Effect sizes: Quantify the magnitude of the effect observed. They provide a measure of the practical significance of the findings, independent of sample size.
4. Reporting and Dissemination: Sharing Your Findings with the World
The final step involves communicating the research findings effectively to the scientific community and the wider public.
4.1 Writing a Research Report:
A well-structured research report is essential for disseminating findings clearly and concisely. Key sections include:
- Abstract: A concise summary of the study.
- Introduction: Background information, research question, and hypotheses.
- Methods: Detailed description of the study design, data collection, and analysis techniques.
- Results: Presentation of the findings, using tables, figures, and statistical summaries.
- Discussion: Interpretation of the results, limitations of the study, and implications for future research.
- Conclusion: Summary of the main findings and their significance.
4.2 Presenting Research Findings:
Researchers may present their work at conferences, workshops, or seminars. Effective presentation skills are crucial for engaging the audience and conveying the key findings.
4.3 Publishing Research:
Publication in peer-reviewed journals is a crucial step for disseminating research to a wider audience and contributing to the scientific literature. The process typically involves submitting a manuscript, undergoing peer review, and making revisions before publication.
5. Data Archiving and Management: Ensuring Long-Term Accessibility
Proper data management is critical for ensuring the long-term accessibility and reproducibility of research findings. This includes:
- Data storage: Securely storing data in a format that is easily accessible and retrievable.
- Data documentation: Creating comprehensive documentation of the data, including metadata, data dictionaries, and codebooks.
- Data sharing: Sharing data with other researchers (where appropriate) to promote transparency and collaboration.
Conclusion: A Journey of Discovery and Rigorous Analysis
The journey from data collection to dissemination is a complex and multifaceted process. It demands careful planning, rigorous execution, and a commitment to transparency and accuracy. By following these steps, researchers can ensure that their findings are valid, reliable, and contribute meaningfully to their field of study. Remember that this is an iterative process – findings might lead to new questions and further research, continuing the cycle of scientific inquiry. The careful analysis of data after its collection forms the heart of this process, ultimately leading to advancements in knowledge and understanding.
Latest Posts
Latest Posts
-
What Is The Connection Between National Sovereignty And Demilitarization
May 11, 2025
-
All Quiet On The Western Front Ch 1 Summary
May 11, 2025
-
B Explain The 2 Specific Options To Legally Drive By
May 11, 2025
-
Thinking Critically And Solving Problems Posttest
May 11, 2025
-
Act 4 Scene 1 Twelfth Night
May 11, 2025
Related Post
Thank you for visiting our website which covers about After Researchers Have Gathered Data What Do They Do Next . We hope the information provided has been useful to you. Feel free to contact us if you have any questions or need further assistance. See you next time and don't miss to bookmark.