An Artificial Neural Network Does All Of The Following Except
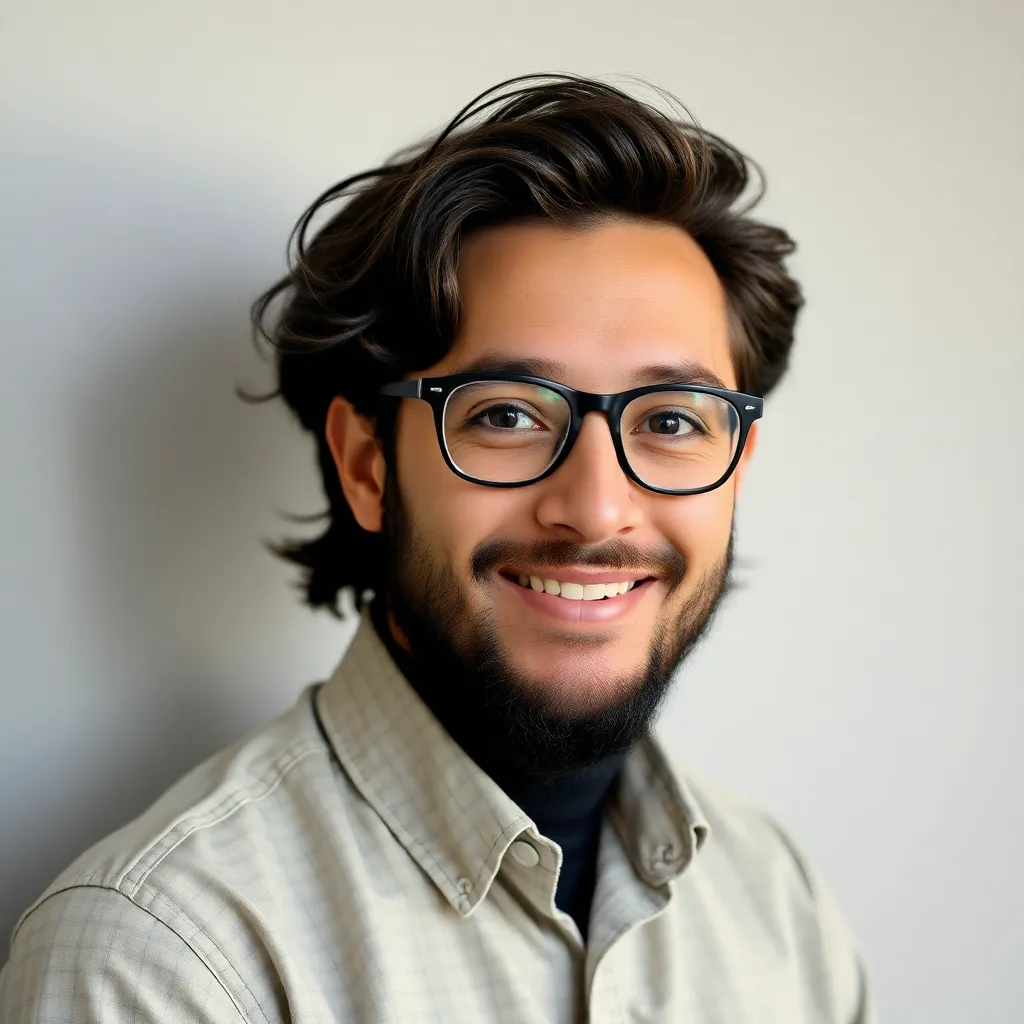
Onlines
May 11, 2025 · 5 min read
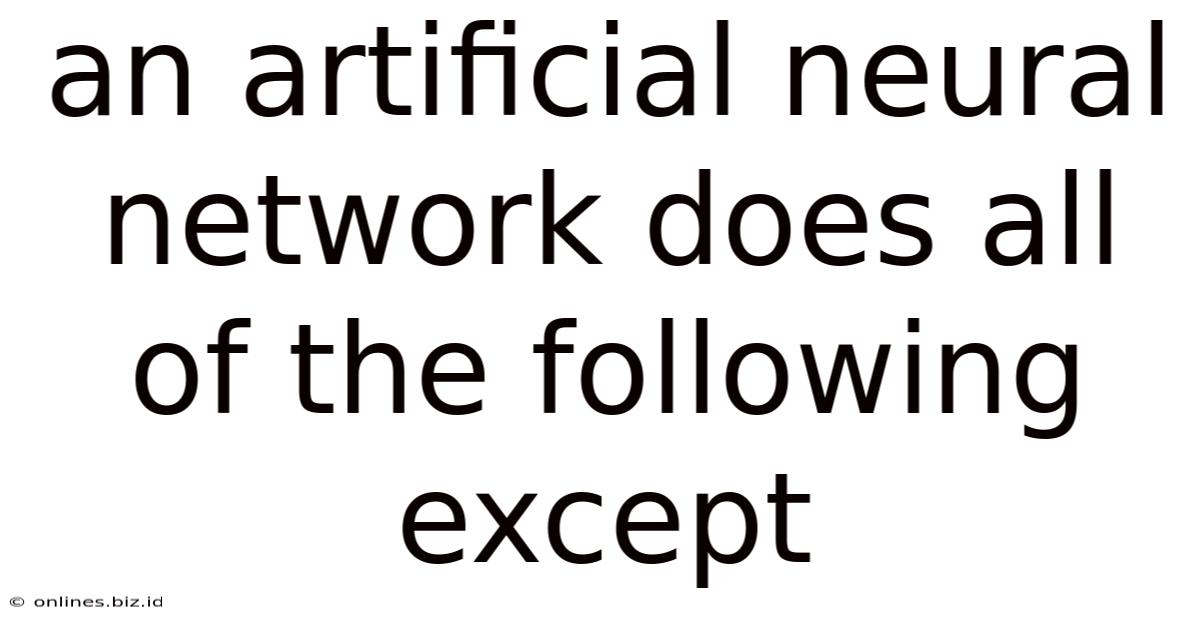
Table of Contents
An Artificial Neural Network Does All of the Following Except…
Artificial Neural Networks (ANNs), inspired by the biological neural networks in the human brain, are revolutionizing various fields. Their ability to learn complex patterns and make predictions from data makes them incredibly powerful tools. But what are their limitations? What tasks are beyond the capabilities of even the most sophisticated ANNs? Let's explore what ANNs can do exceptionally well, and then delve into what they cannot do, at least not without significant augmentation or alternative approaches.
What ANNs Excel At: A Comprehensive Overview
Before we address the limitations, it's crucial to understand the breadth of ANN capabilities. Their applications span a vast landscape:
1. Pattern Recognition and Classification:
- Image Recognition: ANNs are the backbone of facial recognition systems, object detection in self-driving cars, and medical image analysis for disease diagnosis. They can identify intricate patterns in images, even with noise or variations.
- Speech Recognition: Virtual assistants like Siri and Alexa rely heavily on ANNs to convert spoken language into text. These networks can differentiate between different accents, background noise, and varying speaking styles.
- Text Classification: From spam filtering to sentiment analysis, ANNs excel at categorizing textual data. They can discern the emotional tone of a piece of writing, identify topics, and even predict the author's style.
2. Prediction and Forecasting:
- Financial Modeling: ANNs are used to predict stock prices, assess investment risks, and detect fraudulent transactions. Their ability to learn from historical data allows for more accurate and timely financial predictions.
- Weather Forecasting: Incorporating vast amounts of meteorological data, ANNs can improve the accuracy and lead time of weather predictions, aiding in disaster preparedness.
- Demand Forecasting: Businesses utilize ANNs to predict customer demand, optimize inventory management, and streamline supply chains, resulting in significant cost savings.
3. Data Generation and Transformation:
- Image Generation: Generative Adversarial Networks (GANs), a type of ANN, can create realistic images, from portraits to landscapes. This has implications for art, design, and even medical imaging.
- Text Generation: ANNs can generate human-quality text, aiding in tasks like writing summaries, translating languages, and creating creative content.
- Data Augmentation: ANNs can be employed to artificially increase the size of datasets by generating synthetic data, particularly valuable when real-world data is scarce.
4. Control and Optimization:
- Robotics: ANNs are at the heart of advanced robotics, enabling robots to learn complex movements, adapt to unpredictable environments, and interact more naturally with humans.
- Game Playing: From mastering chess and Go to excelling at video games, ANNs demonstrate exceptional strategic thinking and decision-making capabilities.
- Process Optimization: ANNs can be used to optimize industrial processes, improving efficiency, reducing waste, and enhancing overall productivity.
What ANNs Cannot Do (Yet): The Limitations and Challenges
Despite their impressive capabilities, ANNs have inherent limitations. These limitations are actively being researched, but some fundamental challenges remain:
1. Lack of True Understanding and Reasoning:
This is perhaps the most significant limitation. While ANNs can identify patterns and make predictions with remarkable accuracy, they do not possess true understanding or common sense reasoning. They operate based on statistical correlations in the data, not on genuine comprehension of the underlying concepts. This means they can be easily fooled by adversarial examples – subtly altered inputs that cause the network to make incorrect predictions.
2. Data Dependency and Bias:
ANNs are heavily reliant on the quality and quantity of the data they are trained on. Biased or incomplete data will lead to biased or inaccurate results. This is a significant concern, as it can perpetuate and amplify existing societal biases. Furthermore, training large ANNs requires massive datasets, which can be expensive and time-consuming to acquire and process.
3. Explainability and Interpretability:
Many ANNs, particularly deep learning models, are "black boxes." It's often difficult, if not impossible, to understand exactly how they arrive at their predictions. This lack of transparency makes it challenging to debug errors, identify biases, and build trust in their outputs, especially in critical applications like healthcare and finance.
4. Generalization and Transfer Learning:
While ANNs can learn complex patterns, they often struggle to generalize their knowledge to new, unseen data or tasks. This necessitates training separate models for each specific application, which is inefficient and resource-intensive. Transfer learning, where knowledge from one task is transferred to another, is an active area of research to mitigate this problem, but it's not a perfect solution.
5. Computational Cost and Energy Consumption:
Training large ANNs requires significant computational resources and energy. This limits their accessibility to researchers and organizations with substantial computing power. The environmental impact of training these models is also a growing concern.
6. Inability to Handle Symbolic Reasoning and Abstract Concepts:
ANNs struggle with tasks requiring symbolic reasoning, logical deduction, and the manipulation of abstract concepts. They cannot easily understand and process information expressed in symbolic form, such as mathematical equations or logical statements. This limits their applicability to problems that require higher-level cognitive abilities.
7. Difficulty in Handling Uncertainty and Ambiguity:
Real-world data is often noisy, incomplete, and ambiguous. ANNs can struggle to deal with uncertainty and make robust predictions in such situations. They often require carefully pre-processed and cleaned data, which can be a significant hurdle.
8. The Need for Human Supervision and Intervention:
Despite advancements in automated machine learning, ANNs still require significant human intervention in terms of data preparation, model selection, training, and evaluation. Expert knowledge is crucial throughout the entire process, from problem definition to deployment and monitoring.
Conclusion: The Future of ANNs
Artificial Neural Networks are remarkably powerful tools, capable of tackling complex problems across diverse domains. However, it's crucial to acknowledge their limitations. They are not a panacea for every problem, and their application requires careful consideration of their strengths and weaknesses. The future of ANNs lies in addressing these limitations through continued research in areas such as explainable AI, robust generalization, efficient training algorithms, and the integration of ANNs with other AI techniques. By combining the power of ANNs with the strengths of symbolic reasoning systems and human expertise, we can unlock even greater potential and create truly intelligent systems capable of addressing the most challenging problems facing humanity. The journey toward creating truly general-purpose artificial intelligence remains a significant challenge, but the advancements made in ANNs represent a crucial step in that direction.
Latest Posts
Latest Posts
-
Which Of The Following Is True About Adjusting Entries
May 12, 2025
-
Comprehensive Problem 1 Part 2 And Part 3
May 12, 2025
-
An Operations Manager Is Not Likely To Be Involved In
May 12, 2025
-
Competitive Strategy Determines All Of The Following Except
May 12, 2025
-
Ions In Polar Solvents Quick Check
May 12, 2025
Related Post
Thank you for visiting our website which covers about An Artificial Neural Network Does All Of The Following Except . We hope the information provided has been useful to you. Feel free to contact us if you have any questions or need further assistance. See you next time and don't miss to bookmark.