An Example Of Multivariate Analysis Would Be
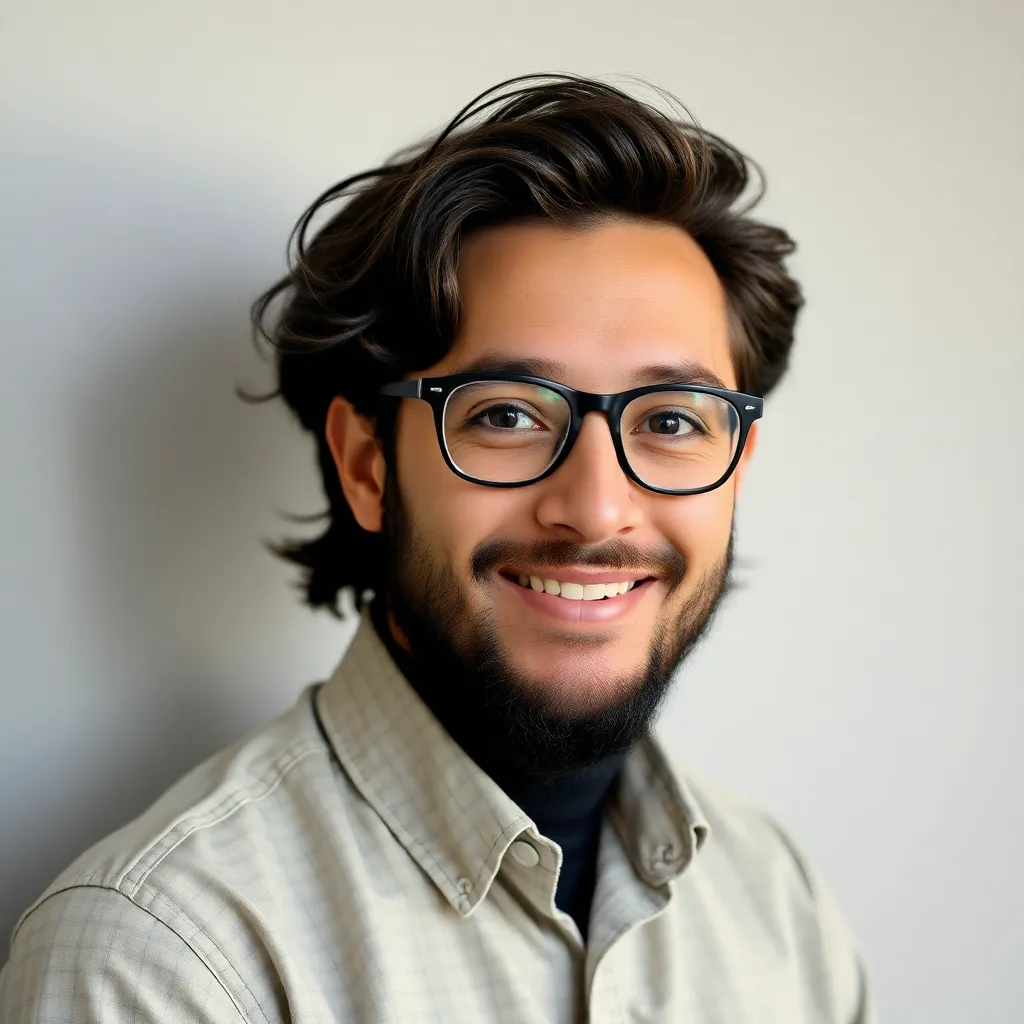
Onlines
May 09, 2025 · 6 min read
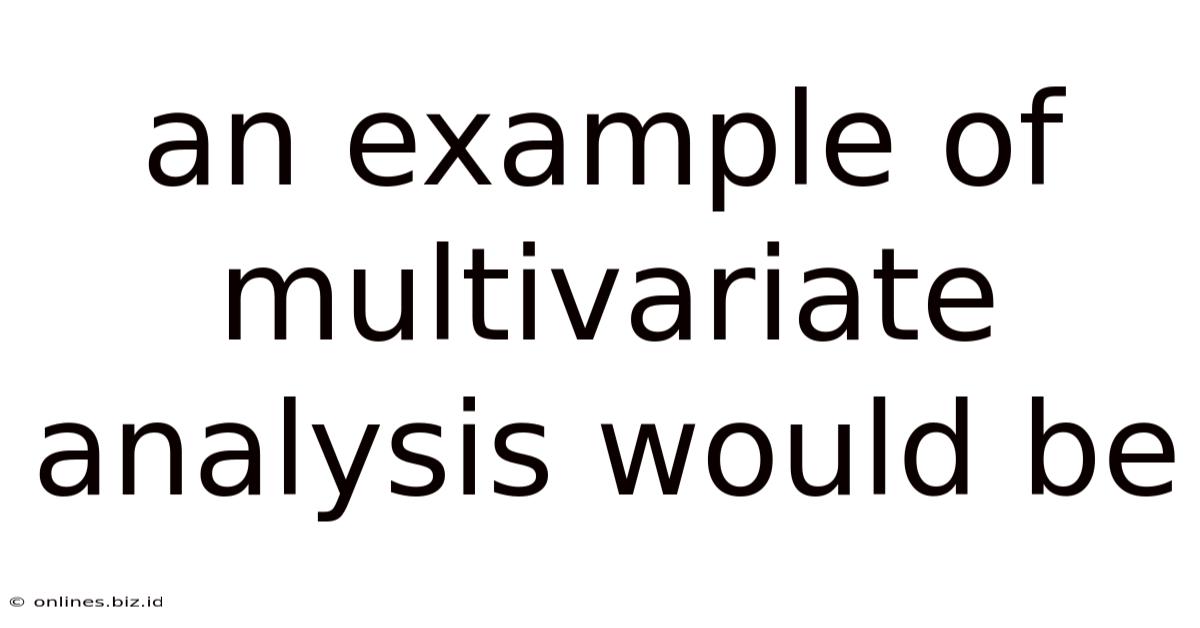
Table of Contents
An Example of Multivariate Analysis: Understanding Customer Segmentation with Market Research Data
Multivariate analysis is a powerful statistical technique used to analyze data sets containing more than one variable. Unlike univariate analysis (which examines a single variable) or bivariate analysis (which examines the relationship between two variables), multivariate analysis delves into the relationships among multiple variables simultaneously. This allows for a richer, more nuanced understanding of complex phenomena. This article will delve into a practical example of multivariate analysis using a hypothetical market research data set focusing on customer segmentation.
The Business Problem: Understanding Customer Preferences for a New Product Line
Let's imagine a company, "Innovate Gadgets," is preparing to launch a new line of smart home devices. Before launching, they want to understand their potential customer base better, specifically identifying distinct customer segments with different preferences and needs. This will inform their marketing strategies, product development, and overall launch plan. They've conducted extensive market research collecting data on potential customers.
The Data Set: Variables and Measurement
The market research yielded a dataset containing the following variables for each respondent:
- Age: (Continuous variable, measured in years)
- Gender: (Categorical variable: Male, Female, Other)
- Income: (Continuous variable, measured in annual dollars)
- TechSavviness: (Ordinal variable, measured on a scale of 1 to 5, with 1 being "Not at all tech-savvy" and 5 being "Extremely tech-savvy")
- Interest in Smart Home Devices: (Ordinal variable, measured on a scale of 1 to 5, with 1 being "Not at all interested" and 5 being "Extremely interested")
- Preferred Purchasing Channel: (Categorical variable: Online, In-store, Both)
This data represents a classic multivariate scenario; we're dealing with multiple variables, both continuous and categorical, and we need to uncover the relationships between them to understand customer segments.
Choosing the Right Multivariate Analysis Technique: Cluster Analysis
For this scenario, cluster analysis is a suitable multivariate technique. Cluster analysis aims to group individuals (in this case, potential customers) into clusters based on their similarity across multiple variables. Individuals within the same cluster will be more similar to each other than to individuals in other clusters.
There are several types of cluster analysis, including:
- K-means clustering: This is a popular algorithm that partitions the data into k clusters, where k is a pre-defined number.
- Hierarchical clustering: This builds a hierarchy of clusters, allowing for different levels of granularity in the analysis.
For our example, let's assume we use k-means clustering with the goal of identifying three distinct customer segments (k=3). Before applying the algorithm, it's crucial to pre-process the data. This often includes:
- Standardization: Converting variables to a similar scale (e.g., z-scores) is important because variables measured on different scales (like age and income) might disproportionately influence the clustering results.
- Handling Missing Data: Missing values should be addressed through imputation or removal, depending on the extent of the missing data and the chosen method.
Implementing the Cluster Analysis and Interpreting the Results
After pre-processing, the k-means algorithm is applied to the standardized data. The algorithm iteratively assigns individuals to clusters, aiming to minimize the within-cluster variance (the variation within each cluster) and maximize the between-cluster variance (the variation between the clusters).
The output of the cluster analysis will include:
- Cluster assignments: Each individual will be assigned to one of the three clusters.
- Cluster centroids: These represent the average values of each variable for each cluster. These centroids are crucial for understanding the characteristics of each segment.
Let's imagine the following cluster centroids emerge from our analysis:
Cluster 1: "Tech-Savvy Affluents"
- Age: 35
- Gender: Relatively balanced
- Income: High
- TechSavviness: 4.5
- Interest in Smart Home Devices: 4.8
- Preferred Purchasing Channel: Online
Cluster 2: "Budget-Conscious Early Adopters"
- Age: 28
- Gender: Slightly more male
- Income: Medium
- TechSavviness: 4.0
- Interest in Smart Home Devices: 4.2
- Preferred Purchasing Channel: Both (Online and In-store)
Cluster 3: "Cautious Consumers"
- Age: 50
- Gender: Relatively balanced
- Income: Low to Medium
- TechSavviness: 2.0
- Interest in Smart Home Devices: 2.5
- Preferred Purchasing Channel: In-store
Marketing Implications and Strategic Recommendations
The cluster analysis reveals three distinct customer segments with unique characteristics:
-
Tech-Savvy Affluents: This group is older, wealthier, highly tech-savvy, and strongly interested in smart home devices. They prefer online purchasing. Marketing efforts should focus on highlighting the advanced features, seamless integration, and premium quality of the products.
-
Budget-Conscious Early Adopters: This younger segment is moderately tech-savvy and interested in smart home devices but is price-sensitive. They are open to both online and in-store purchasing. Marketing should emphasize value for money, innovative features, and easy-to-use interfaces. Promotional offers and flexible payment options could be attractive.
-
Cautious Consumers: This older group is less tech-savvy and less interested in smart home devices. They prefer in-store purchasing. Marketing needs to focus on simplicity, ease of use, and clear demonstrations of the benefits. Emphasis on reliability and customer service would also be beneficial.
Further Considerations and Extensions
This example showcases the power of multivariate analysis in market research. However, several other considerations and extensions are possible:
-
Validation: The chosen number of clusters (k=3) should be validated. Techniques like silhouette analysis or the elbow method can help determine the optimal number of clusters.
-
Other Multivariate Techniques: Depending on the research questions, other multivariate techniques such as discriminant analysis, factor analysis, or principal component analysis could be employed. For instance, factor analysis could be used to reduce the number of variables by identifying underlying latent factors that explain the observed correlations among the variables.
-
Predictive Modeling: The cluster assignments could be used as input variables in predictive models to forecast sales or customer lifetime value.
-
Incorporating Qualitative Data: Combining quantitative data with qualitative data (e.g., open-ended survey responses) can provide richer insights into customer motivations and preferences.
Conclusion: The Power of Multivariate Analysis in Unveiling Hidden Patterns
Multivariate analysis provides a robust framework for analyzing complex datasets and uncovering meaningful relationships between multiple variables. This example demonstrates how cluster analysis can help segment customers based on their characteristics and preferences, providing valuable insights for marketing and product development. By understanding customer segments, Innovate Gadgets can tailor its marketing messages, product features, and distribution channels to effectively reach each target group, maximizing the success of its new product launch. The application of multivariate techniques is not limited to market research; they find applications across various fields, including finance, healthcare, and social sciences, providing powerful tools for data-driven decision-making. Remember to always carefully consider the chosen technique, data preprocessing, and interpretation of results to ensure reliable and meaningful conclusions.
Latest Posts
Latest Posts
-
Ultimate Source Of Energy That Drives The Food Chain
May 09, 2025
-
Which Statement About The Field Of Abnormal Psychology Is Accurate
May 09, 2025
-
Consider The Reaction Of An Alkyl Bromide And Hydroxide Ion
May 09, 2025
-
Algebra 1 Unit 4 Test Answer Key
May 09, 2025
-
Which Statement Describes Both Predators And Parasites
May 09, 2025
Related Post
Thank you for visiting our website which covers about An Example Of Multivariate Analysis Would Be . We hope the information provided has been useful to you. Feel free to contact us if you have any questions or need further assistance. See you next time and don't miss to bookmark.