An Is A Scheme Of Calssifying Informaiton An Dknoweldge
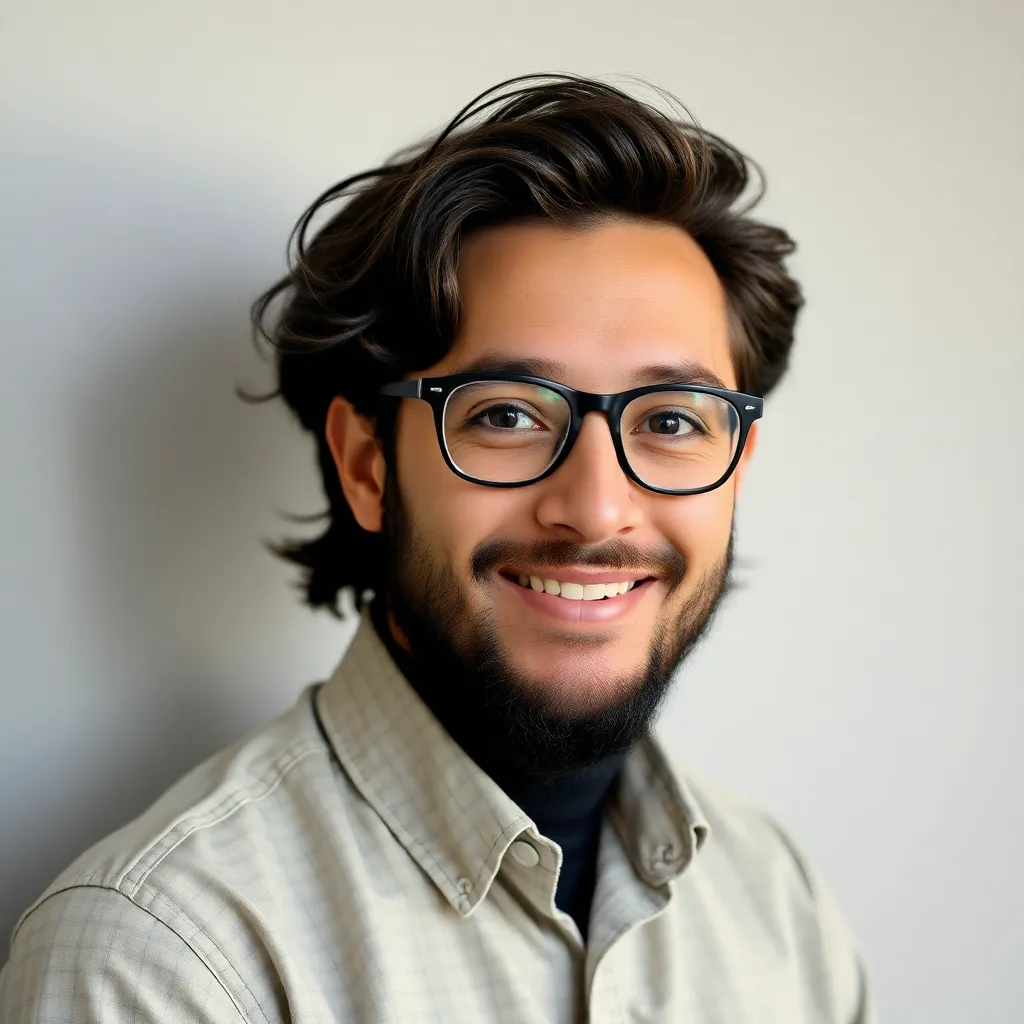
Onlines
Apr 24, 2025 · 6 min read

Table of Contents
An Ontology Is a Scheme of Classifying Information and Knowledge
Ontologies are formal representations of knowledge within a specific domain. They provide a structured vocabulary and a set of relationships that define concepts and their interconnections. Understanding ontologies is crucial for anyone working with large datasets, knowledge representation, and artificial intelligence. This article dives deep into the intricacies of ontologies, explaining their purpose, structure, components, creation process, and applications across various fields.
What is an Ontology?
At its core, an ontology is a formal representation of knowledge. Think of it as a sophisticated, structured dictionary that not only defines terms but also specifies how those terms relate to each other. Unlike a simple dictionary, an ontology goes beyond providing definitions; it articulates the relationships between concepts, allowing for complex reasoning and inference. This structured nature makes ontologies invaluable for organizing, searching, and analyzing information.
Key Characteristics of an Ontology:
- Formalized: Ontologies employ formal languages, such as OWL (Web Ontology Language) or RDF (Resource Description Framework), to ensure precision and consistency. This formalization enables computers to process and reason with the knowledge represented.
- Domain-Specific: An ontology is typically focused on a particular domain or area of expertise. For example, you might have an ontology for medical terminology, another for e-commerce products, and yet another for geographical information. This focus ensures relevance and accuracy.
- Shared Understanding: Ontologies aim to establish a common understanding of concepts and their relationships within a community of users. This shared understanding facilitates interoperability and collaboration.
- Reusable: Well-designed ontologies can be reused across multiple applications and systems. This reusability saves time and resources.
Components of an Ontology:
An ontology typically consists of several key components:
1. Concepts:
Concepts are the fundamental building blocks of an ontology. They represent objects, events, situations, or abstract ideas within the domain. Examples include "patient," "disease," "treatment," "product," "customer," or "location." Each concept is usually given a unique identifier and a definition.
2. Relationships:
Relationships define how concepts are connected. These connections can represent various types of associations, such as:
- Is-a (subclass-superclass): Indicates a hierarchical relationship, where one concept is a subtype of another. For example, "Dog is-a Mammal."
- Part-of (meronymic): Represents a part-whole relationship. For instance, "Wheel part-of Car."
- Has-property: Associates a concept with its attributes or characteristics. For example, "Patient has-property Age."
- Causes: Indicates a causal relationship between concepts. For example, "Smoking causes Lung Cancer."
3. Axioms:
Axioms are logical statements that define constraints and relationships between concepts. They provide a formal means of specifying the rules and inferences allowed within the ontology. For example, an axiom might state that "all mammals are vertebrates."
4. Instances:
Instances are specific examples of concepts. For instance, "Fido" would be an instance of the concept "Dog."
The Ontology Creation Process:
Building a robust and effective ontology is an iterative process that typically involves the following steps:
1. Requirements Gathering and Domain Analysis:
This crucial initial phase involves identifying the scope of the ontology, defining the specific domain it will cover, and determining the key concepts and relationships to be included. Collaboration with domain experts is essential during this stage.
2. Conceptual Modeling:
This stage involves creating a high-level representation of the ontology, often using diagrams and conceptual models. Popular tools include UML (Unified Modeling Language) diagrams and ontology editors.
3. Formalization:
In this phase, the conceptual model is translated into a formal language like OWL or RDF. This step requires careful attention to detail and adherence to the chosen language's syntax and semantics.
4. Implementation and Population:
Once formalized, the ontology needs to be implemented in a knowledge representation system and populated with data. This often involves integrating the ontology with databases or other information sources.
5. Evaluation and Refinement:
After implementation, the ontology needs to be evaluated for completeness, consistency, and accuracy. This may involve testing and refinement based on feedback from users and domain experts. The process is iterative, requiring continuous improvement and adaptation.
Applications of Ontologies:
Ontologies have a wide range of applications across various fields:
1. Semantic Web:
Ontologies are fundamental to the Semantic Web, enabling machines to understand and process information on the web more effectively. They facilitate interoperability between different systems and enable more intelligent search and data integration.
2. Knowledge Management:
Ontologies provide a structured way to organize and manage knowledge within an organization. They can improve knowledge sharing, facilitate knowledge discovery, and support decision-making.
3. Information Retrieval:
Ontologies enhance information retrieval by providing a more precise and meaningful way to search and retrieve information. They can improve search accuracy and reduce the amount of irrelevant information returned.
4. Data Integration:
Ontologies can integrate data from multiple sources by providing a common framework for representing and interpreting information. This is particularly useful in situations where data is stored in different formats and schemas.
5. Artificial Intelligence:
Ontologies play a critical role in various AI applications, including knowledge-based systems, expert systems, and natural language processing. They provide a structured representation of knowledge that AI systems can reason with.
6. Bioinformatics:
In bioinformatics, ontologies are used to represent biological knowledge, including gene functions, protein interactions, and metabolic pathways. They facilitate data analysis, knowledge discovery, and interoperability between different biological databases.
7. E-commerce:
In e-commerce, ontologies can be used to represent product information, customer profiles, and other relevant data. This can improve search, recommendation systems, and personalized shopping experiences.
8. Healthcare:
Ontologies are used extensively in healthcare to represent medical terminology, patient data, and clinical guidelines. They facilitate interoperability between different healthcare systems, improve data analysis, and support clinical decision-making.
Choosing the Right Ontology Language:
Several languages are used for creating ontologies, each with its strengths and weaknesses:
- OWL (Web Ontology Language): A widely used standard for representing ontologies on the Semantic Web. It offers a rich set of features for expressing complex relationships and constraints.
- RDF (Resource Description Framework): A more basic language than OWL, but widely used for representing metadata and linked data.
- RDF Schema (RDFS): An extension of RDF that provides a simple way to define classes and properties.
The choice of ontology language depends on the specific requirements of the application and the complexity of the knowledge being represented.
Challenges in Ontology Development:
Creating and maintaining ontologies can be challenging:
- Complexity: Developing a comprehensive and accurate ontology can be a complex and time-consuming task.
- Scalability: As the size and complexity of an ontology grow, managing and maintaining it becomes increasingly difficult.
- Evolution: Ontologies need to evolve over time to reflect changes in the domain and new knowledge.
- Interoperability: Ensuring that ontologies from different sources are compatible and interoperable can be a significant challenge.
Conclusion:
Ontologies are powerful tools for representing and reasoning with knowledge. Their structured and formal nature makes them invaluable for a wide range of applications. While the development and maintenance of ontologies can be challenging, their benefits in terms of improved information management, data integration, and AI applications far outweigh the costs. As the volume and complexity of information continue to grow, the importance of ontologies will only continue to increase. Understanding their structure, components, and applications is essential for anyone working with large datasets, knowledge representation, and artificial intelligence in the modern digital landscape.
Latest Posts
Latest Posts
-
Devil In The White City Characters
Apr 25, 2025
-
16 4 Evidence Of Evolution Answer Key
Apr 25, 2025
-
Exercise 6 Review Sheet Art Labeling Activity 4
Apr 25, 2025
-
Which Factor Distinguishes True Labor From False Labor
Apr 25, 2025
-
The Place Where Motion Begins Is Known As
Apr 25, 2025
Related Post
Thank you for visiting our website which covers about An Is A Scheme Of Calssifying Informaiton An Dknoweldge . We hope the information provided has been useful to you. Feel free to contact us if you have any questions or need further assistance. See you next time and don't miss to bookmark.