Analyzing And Interpreting Scientific Data Pogil
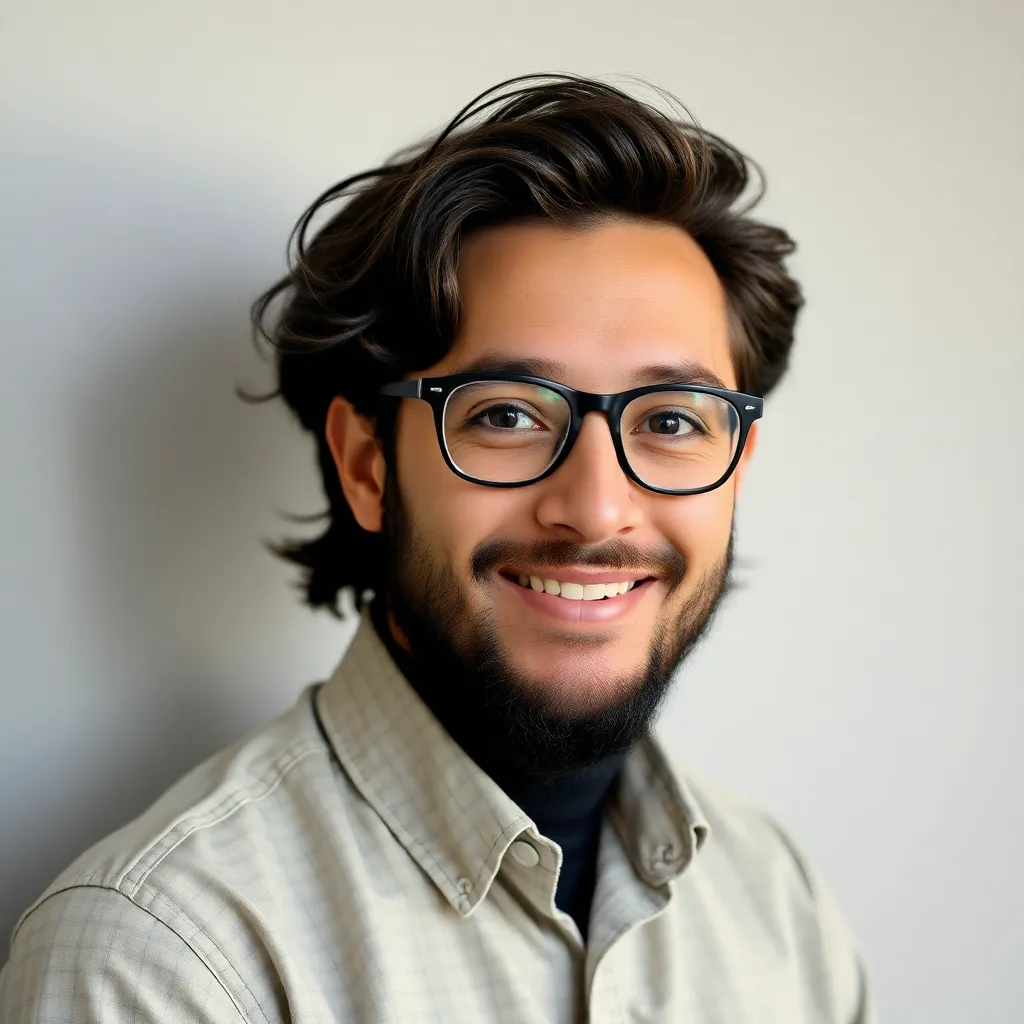
Onlines
May 07, 2025 · 6 min read
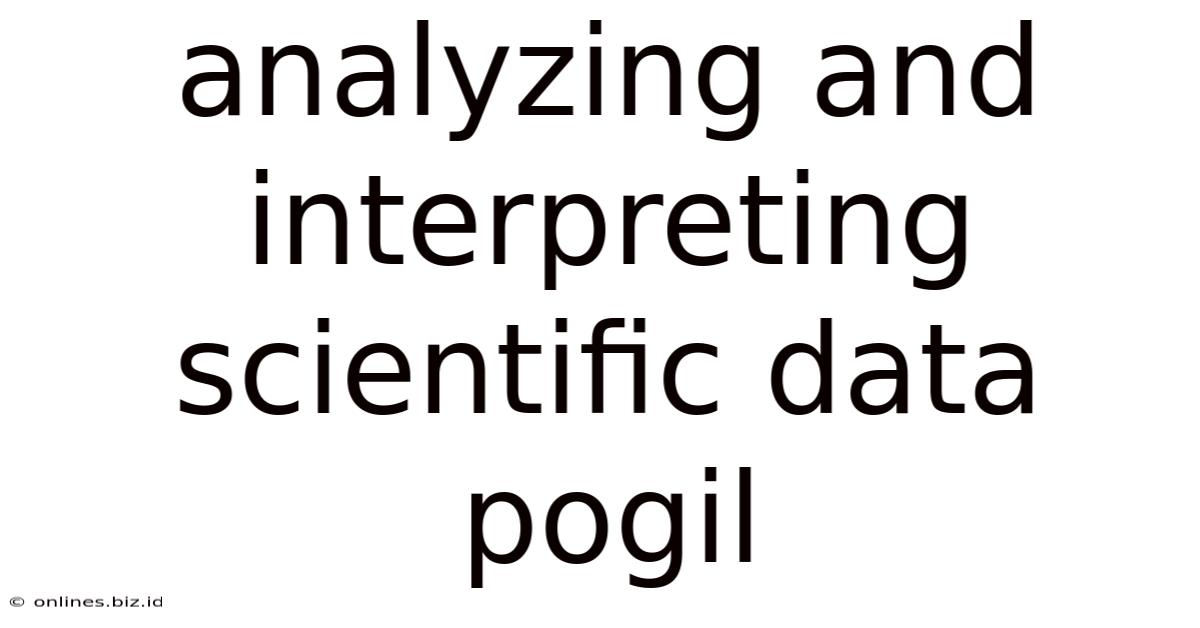
Table of Contents
Analyzing and Interpreting Scientific Data: A Deep Dive into POGIL Activities
The ability to analyze and interpret scientific data is a cornerstone of scientific literacy. It's not enough to simply collect data; understanding what that data means is crucial for drawing meaningful conclusions and advancing scientific knowledge. Process-Oriented Guided-Inquiry Learning (POGIL) activities provide a powerful framework for developing these crucial skills. This article delves into the nuances of analyzing and interpreting scientific data within the POGIL context, offering practical strategies and examples to enhance your understanding and application.
Understanding the POGIL Approach
POGIL is a student-centered, collaborative learning approach where students actively construct their understanding of scientific concepts through guided inquiry. Unlike traditional lecture-based learning, POGIL emphasizes critical thinking, problem-solving, and collaborative learning. In a POGIL activity focusing on data analysis, students aren't simply given the answers; instead, they are presented with raw data and guided through a structured process of analysis and interpretation. This active engagement fosters deeper understanding and retention than passive learning methods.
Key Features of POGIL Data Analysis Activities:
- Collaborative Learning: Students work in small groups to analyze data, fostering discussion and diverse perspectives.
- Structured Inquiry: Activities provide a clear path through the analysis process, guiding students towards meaningful interpretations.
- Critical Thinking: Students are challenged to evaluate data, identify patterns, and draw evidence-based conclusions.
- Self-Assessment: POGIL activities often include self-assessment components, enabling students to gauge their understanding and identify areas needing further attention.
- Real-World Relevance: Data used in POGIL activities is often drawn from real-world scientific studies, making the learning more engaging and relatable.
Stages of Analyzing and Interpreting Scientific Data in POGIL
A typical POGIL activity focusing on data analysis might involve several key stages:
1. Data Exploration and Organization:
This initial stage focuses on familiarizing students with the data set. This might involve:
- Identifying Variables: Determining the independent and dependent variables, along with any control variables.
- Data Cleaning: Identifying and handling missing data or outliers. This may involve removing data points, replacing them with averages, or using more sophisticated statistical methods.
- Data Representation: Choosing appropriate methods to represent the data visually, such as tables, graphs (bar charts, histograms, scatter plots, line graphs), or other visual aids. The choice of representation will depend on the nature of the data and the questions being asked. For example, a scatter plot is ideal for showing the relationship between two continuous variables. A bar chart is best for comparing discrete categories.
Example: Imagine a POGIL activity investigating the relationship between plant growth and fertilizer concentration. The first stage would involve students organizing the data into a table, identifying the independent variable (fertilizer concentration), the dependent variable (plant growth), and any control variables (e.g., sunlight, water). They might then create a scatter plot to visualize the relationship between fertilizer concentration and plant growth.
2. Pattern Recognition and Descriptive Statistics:
Once the data is organized, students move on to identifying patterns and trends. This might involve calculating descriptive statistics such as:
- Measures of Central Tendency: Mean, median, and mode provide information about the central value of the data.
- Measures of Dispersion: Range, variance, and standard deviation describe the spread or variability of the data.
- Frequency Distributions: Histograms and frequency tables show the distribution of data values.
By analyzing these statistics, students can start to understand the characteristics of the data and identify any potential relationships between variables.
Example: In the plant growth experiment, students would calculate the mean plant height for each fertilizer concentration. They might also calculate the standard deviation to determine the variability in plant height within each group. This information could reveal whether higher fertilizer concentrations led to greater plant growth, and whether the effect was consistent across all replicates.
3. Inferential Statistics and Hypothesis Testing:
For more advanced POGIL activities, students might delve into inferential statistics, which allow them to make inferences about a larger population based on a sample of data. This commonly involves:
- Hypothesis Testing: Formulating a null hypothesis (e.g., there is no relationship between fertilizer concentration and plant growth) and an alternative hypothesis (e.g., higher fertilizer concentrations lead to greater plant growth).
- Statistical Tests: Applying appropriate statistical tests (e.g., t-tests, ANOVA, correlation analysis) to determine whether the data supports or refutes the null hypothesis.
- P-values and Significance Levels: Interpreting p-values to assess the statistical significance of the results. A low p-value (typically below 0.05) indicates that the results are unlikely to have occurred by chance.
Example: Students might use a t-test to compare the mean plant height between plants grown with high and low fertilizer concentrations. A low p-value would indicate a statistically significant difference in plant height between the two groups, supporting the alternative hypothesis.
4. Interpretation and Conclusion:
The final stage involves interpreting the results in the context of the research question and drawing conclusions. This requires careful consideration of:
- Statistical Significance: Are the observed patterns statistically significant, or could they be due to chance?
- Effect Size: How large is the effect of the independent variable on the dependent variable?
- Limitations: What are the limitations of the study, and how might these limitations affect the interpretation of the results?
- Further Research: What further research is needed to address unanswered questions or explore related issues?
Example: Students would summarize their findings, stating whether their data supported or refuted their hypothesis. They would also discuss the limitations of their study, such as the sample size or the specific type of fertilizer used. Finally, they might suggest avenues for further research, such as investigating different types of fertilizers or conducting the experiment over a longer period.
Common Challenges and Strategies for Effective POGIL Data Analysis
While POGIL is a powerful approach, certain challenges may arise:
1. Overwhelm with Data:
Large or complex datasets can be overwhelming for students. Strategies to mitigate this include:
- Chunking the Data: Break down the analysis into smaller, manageable steps.
- Guided Practice: Provide ample opportunities for guided practice with smaller datasets before tackling more complex ones.
- Visual Aids: Emphasize the use of visual aids to help students understand the data.
2. Misinterpretation of Statistical Results:
Students may struggle to interpret statistical results correctly. Strategies include:
- Clear Explanations: Provide clear explanations of statistical concepts and their implications.
- Real-World Examples: Use real-world examples to illustrate the meaning of statistical results.
- Practice Problems: Provide ample opportunities for practice interpreting statistical results.
3. Lack of Collaborative Skills:
Effective collaboration is essential for POGIL activities. Strategies include:
- Teamwork Training: Provide explicit training in teamwork skills.
- Structured Group Activities: Use structured group activities to facilitate collaboration.
- Peer Feedback: Encourage peer feedback to enhance learning and collaboration.
Integrating Technology into POGIL Data Analysis
Technology can significantly enhance POGIL data analysis activities. Tools such as:
- Spreadsheet Software: Excel or Google Sheets can facilitate data organization, calculation, and visualization.
- Statistical Software: R or SPSS can be used for more advanced statistical analyses.
- Data Visualization Tools: Tools like Tableau or Power BI can create compelling visualizations of the data.
These tools can empower students to perform more sophisticated analyses and better understand their data.
Conclusion
Analyzing and interpreting scientific data is a crucial skill for scientific literacy. POGIL activities offer a valuable framework for developing this skill through active engagement, collaborative learning, and structured inquiry. By following the stages outlined in this article and addressing potential challenges proactively, educators can effectively implement POGIL data analysis activities that foster deep understanding and prepare students for future scientific endeavors. Remember that the key to successful POGIL implementation lies in careful planning, clear instructions, and a supportive learning environment that encourages active participation and critical thinking. The rewarding outcome will be students who are not only proficient in data analysis but also confident and capable scientific thinkers.
Latest Posts
Latest Posts
-
Summary Of Things Fall Apart Chapter 5
May 11, 2025
-
Summary Of Book 7 Of The Odyssey
May 11, 2025
-
Visionary Ceos See Global Citizenship As An Opportunity To
May 11, 2025
-
Table 2 Speed Of Diffusion Of Different Molecular Weight Dyes
May 11, 2025
-
Bullying In Early Adolescence Commonlit Answers
May 11, 2025
Related Post
Thank you for visiting our website which covers about Analyzing And Interpreting Scientific Data Pogil . We hope the information provided has been useful to you. Feel free to contact us if you have any questions or need further assistance. See you next time and don't miss to bookmark.