Circuit Training Statistics Exploring 1 Variable Data Unit Review
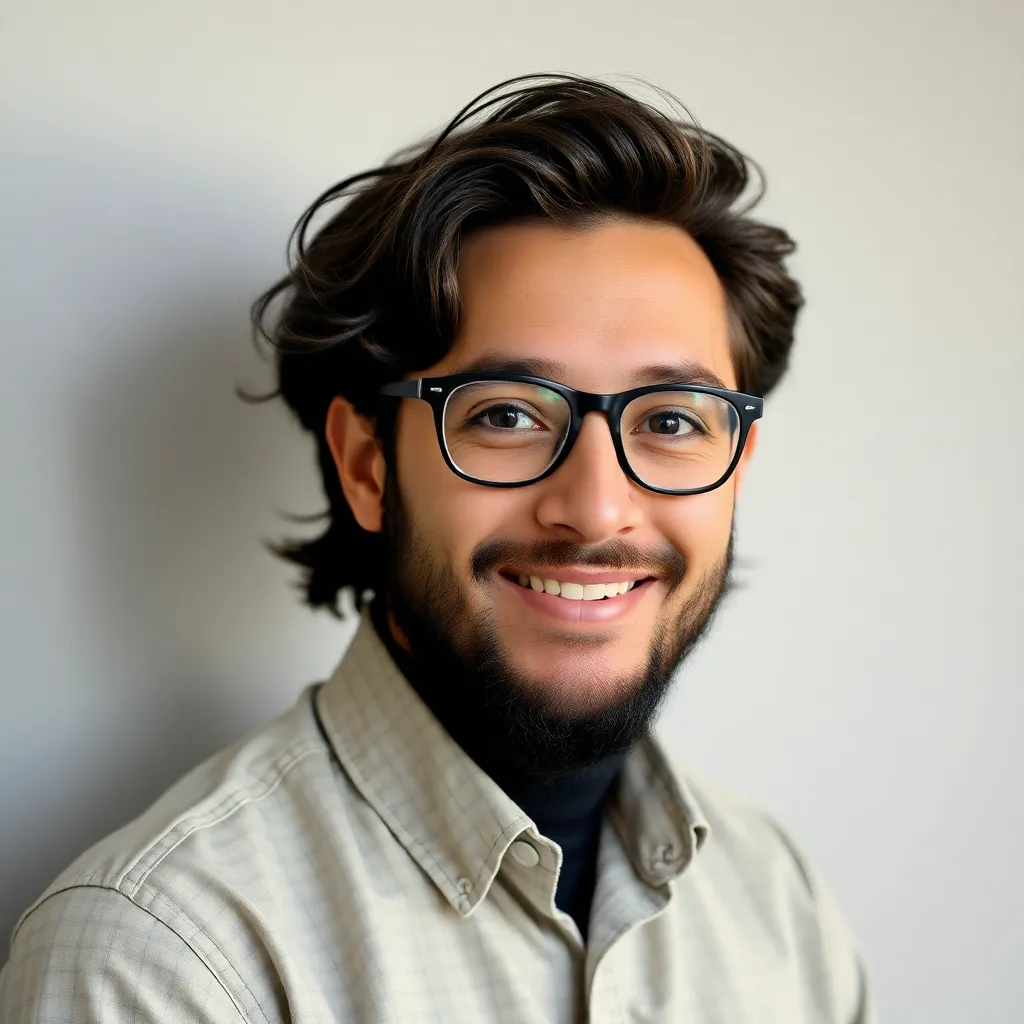
Onlines
Apr 14, 2025 · 6 min read

Table of Contents
Circuit Training Statistics: Exploring 1-Variable Data Unit Review
Circuit training, a popular fitness methodology, offers a dynamic blend of cardiovascular exercise and strength training. Analyzing its effectiveness requires a robust statistical approach, often beginning with the exploration of single-variable data units. This article delves into the statistical methods used to analyze data collected from circuit training programs, focusing on the review and interpretation of one-variable datasets. We will explore various statistical measures and their implications for understanding the impact of circuit training.
Understanding the Data: The Single Variable
Before diving into statistical analysis, it's crucial to define the variable of interest. In the context of circuit training, several single-variable data units could be analyzed. These might include:
-
Heart Rate (HR): A key indicator of cardiovascular intensity during and after circuit training sessions. Analyzing HR data can help determine the effectiveness of the training program in improving cardiovascular fitness.
-
Weight Loss (kg or lbs): A common goal of many circuit training programs. Analyzing weight loss data helps assess the program's efficacy in promoting fat loss and weight management.
-
Muscle Strength (e.g., 1RM): Measured through one-repetition maximum (1RM) tests for various exercises. This data reveals the program's success in building muscular strength.
-
Body Composition (e.g., % Body Fat): Determined through methods like DEXA scans or bioelectrical impedance analysis. Changes in body composition provide a more nuanced understanding of fitness improvements beyond just weight loss.
-
Exercise Performance (e.g., reps completed): Tracking the number of repetitions performed in each exercise during a circuit provides insight into training adaptations and fatigue levels.
-
Resting Heart Rate (RHR): A measure of cardiovascular fitness often showing improvement with regular training. Lower RHR indicates increased cardiovascular efficiency.
-
Blood Pressure: Analyzing changes in systolic and diastolic blood pressure helps assess the impact of the training on cardiovascular health.
-
Rating of Perceived Exertion (RPE): A subjective measure of how hard someone feels they are working during the circuit. This data offers valuable insights into the participant's experience and training intensity.
This article will use the example of heart rate (HR) as our single variable data unit to illustrate the statistical analysis. The principles discussed can be applied to any of the variables listed above.
Descriptive Statistics: Summarizing the Data
Once the data is collected, descriptive statistics are used to summarize and understand the main features of the dataset. Key descriptive statistics include:
1. Measures of Central Tendency:
-
Mean: The average heart rate across all observations. It provides a central point of the data.
-
Median: The middle value when the data is arranged in ascending order. It's less sensitive to outliers compared to the mean.
-
Mode: The most frequent heart rate value. It indicates the most common heart rate observed.
For example, if we collected heart rate data from 10 participants after a circuit training session, we can calculate the mean, median, and mode to understand the typical heart rate response. A high mean heart rate could indicate a high-intensity workout. Discrepancies between the mean and median might suggest the presence of outliers.
2. Measures of Dispersion:
-
Range: The difference between the highest and lowest heart rates. It provides a simple measure of the data's spread.
-
Variance: The average of the squared differences from the mean. It quantifies the overall variability in the data.
-
Standard Deviation: The square root of the variance. It represents the typical deviation of individual heart rates from the mean. A larger standard deviation indicates greater variability in heart rate responses.
For instance, a large range or standard deviation in heart rate suggests a wide variation in individual responses to the circuit training, requiring further investigation into potential influencing factors such as fitness levels, age, or adherence to the program.
3. Visualizations:
Histograms, box plots, and frequency distributions are valuable visual tools to understand the distribution of the heart rate data. These visualizations provide a clear picture of the data's shape, identifying potential outliers or skewness. A skewed distribution might indicate a need for further analysis using non-parametric methods.
Inferential Statistics: Drawing Conclusions
Descriptive statistics provide a summary of the data, but inferential statistics allow us to draw conclusions about a larger population based on the sample data. If, for instance, we want to determine if circuit training significantly increases heart rate compared to a control group, we need inferential statistics.
For a single variable like heart rate, we can use:
-
t-tests: If we compare the mean heart rate of a group before and after circuit training, we can use a paired samples t-test to determine if the difference is statistically significant. This test assesses if the observed changes are likely due to the circuit training or random chance.
-
One-sample t-test: This test can be used to compare the mean heart rate of a group after circuit training against a pre-defined value (e.g., a target heart rate zone).
-
Non-parametric tests: If the data doesn't meet the assumptions of parametric tests (like normality), non-parametric equivalents such as the Wilcoxon signed-rank test (for paired samples) or Mann-Whitney U test (for independent samples) should be used. These tests are less sensitive to outliers and violations of normality assumptions.
Considering Potential Biases and Limitations
When interpreting statistical results, it's crucial to acknowledge potential biases and limitations:
-
Sampling Bias: If the participants are not representative of the broader population, the results may not be generalizable.
-
Measurement Error: Inaccurate measurement of heart rate (or any other variable) can affect the results.
-
Confounding Variables: Other factors (diet, sleep, stress levels) can influence the outcome and should be considered. Control groups and careful experimental design are essential to minimize these effects.
-
Small Sample Size: A small sample size can reduce the statistical power, making it difficult to detect significant differences even if they exist.
-
Data Integrity: The accuracy of the data collection methods greatly influences the validity of any conclusions drawn.
Advanced Statistical Techniques
For more complex analyses, the following techniques can be employed:
-
Regression analysis: To investigate the relationship between heart rate and other variables (e.g., age, fitness level).
-
ANOVA (Analysis of Variance): To compare heart rate across multiple groups (e.g., different circuit training programs).
-
Time series analysis: To track changes in heart rate over time during and after the circuit training program.
-
Clustering techniques: Group participants based on their responses to different elements within the circuit training to investigate individual responses and determine appropriate training modifications.
-
Survival analysis: Explore the time taken to reach certain fitness goals or the time until dropout from the circuit training program.
Conclusion: The Importance of Statistical Analysis in Circuit Training
Statistical analysis plays a crucial role in understanding the effectiveness of circuit training programs. By employing both descriptive and inferential statistics, researchers can gain valuable insights into the program's impact on various physiological variables. Careful consideration of potential biases and limitations is necessary for a valid and reliable interpretation of the results. The use of advanced statistical techniques can provide a deeper understanding of the complex relationships between circuit training and its effects on fitness and health. Remember that statistical analysis is a tool; it’s the interpretation and application of these results that inform the development and improvement of effective and safe circuit training programs. Always prioritize the safety and well-being of participants and ensure ethical considerations are observed throughout the research process.
Latest Posts
Latest Posts
-
Symbolism In Never Let Me Go
Apr 15, 2025
-
En Muchos Paises Hispanos El Gobierno Ofrece Servicios Medicos
Apr 15, 2025
-
What Is Advertising Used For Check All That Apply
Apr 15, 2025
-
What Statement About Conflict Is True
Apr 15, 2025
-
How Have Individuals In Your Life Influenced Your Schema Development
Apr 15, 2025
Related Post
Thank you for visiting our website which covers about Circuit Training Statistics Exploring 1 Variable Data Unit Review . We hope the information provided has been useful to you. Feel free to contact us if you have any questions or need further assistance. See you next time and don't miss to bookmark.