Common Data Quality Problems Include All Of The Following Except
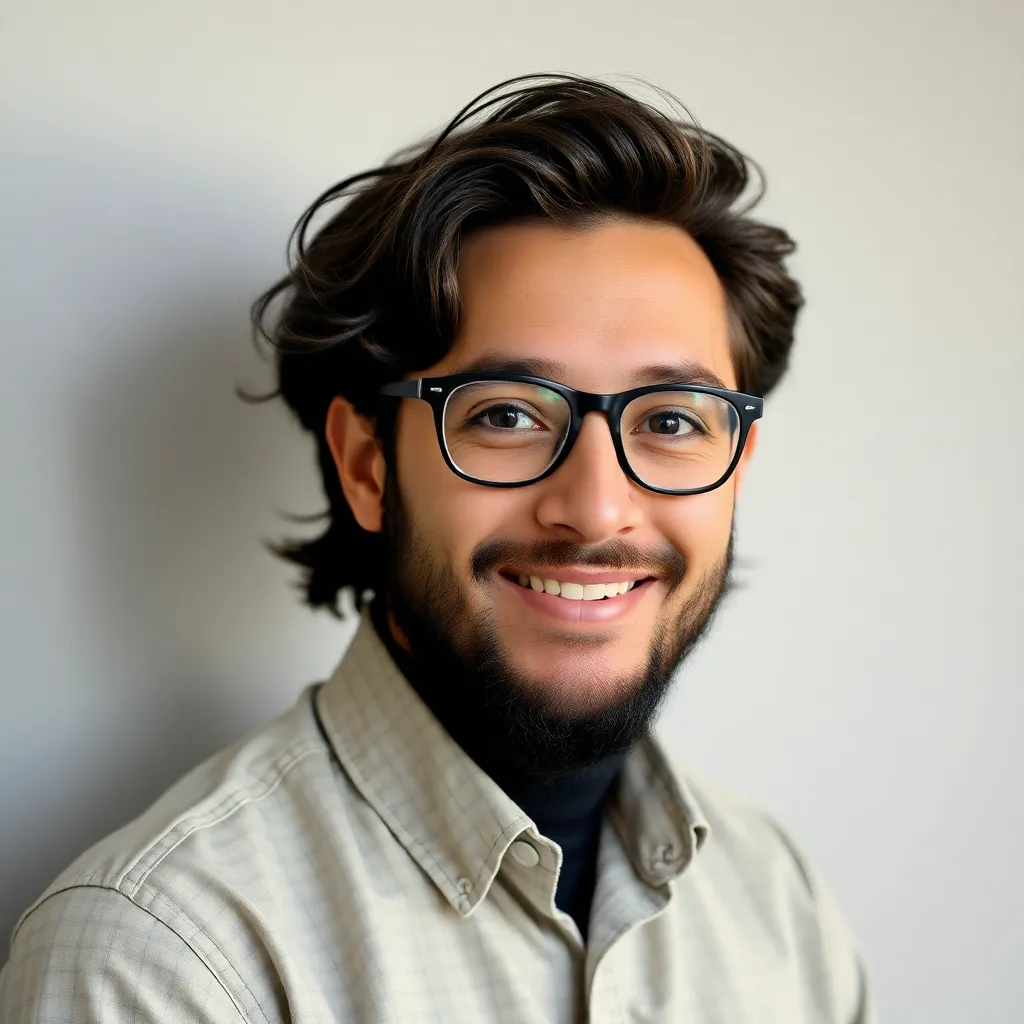
Onlines
May 09, 2025 · 6 min read
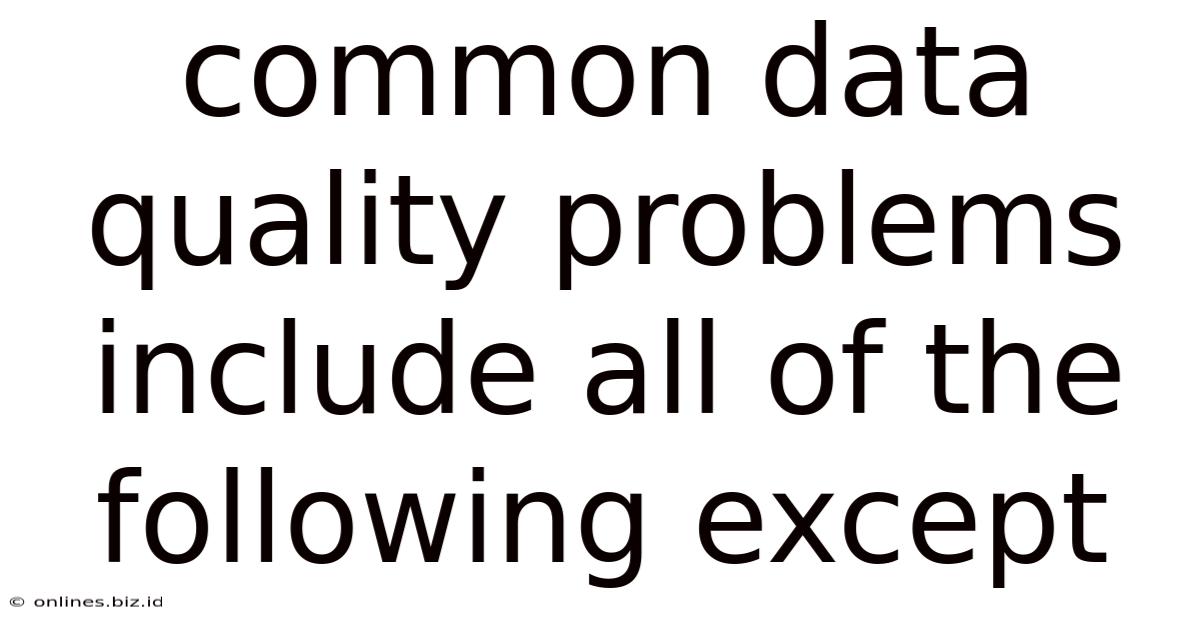
Table of Contents
Common Data Quality Problems: All Except...
Data quality is the cornerstone of any successful business. Making informed decisions, developing accurate predictions, and maintaining a competitive edge all hinge on having reliable, consistent, and accurate data. However, achieving pristine data is often a significant challenge. Many organizations grapple with various data quality issues that can negatively impact their operations. This article explores the common data quality problems businesses face, focusing on what is not typically considered a primary issue, within the broader context of ensuring data integrity.
Common Data Quality Problems: A Comprehensive Overview
Before we identify the outlier, let's establish a solid foundation by outlining the common pitfalls that organizations encounter when managing their data:
1. Inconsistent Data
This is perhaps the most prevalent problem. Inconsistent data arises when the same information is recorded differently across various sources or systems. For example, a customer's name might be written as "John Smith" in one database, "John A. Smith" in another, and "J. Smith" in a third. This inconsistency creates significant challenges for data analysis and reporting.
Impact: Inconsistency hinders accurate reporting and analysis, leading to flawed business decisions. It makes data integration complex and time-consuming, increasing the risk of errors.
2. Incomplete Data
Incomplete data refers to missing information in a dataset. This can occur due to various reasons, such as data entry errors, incomplete forms, or data loss during transfer. For instance, a customer record might be missing a crucial piece of information like their email address or phone number.
Impact: Incomplete data limits the effectiveness of analysis and reporting. It reduces the accuracy of predictions and models, potentially leading to missed opportunities or incorrect conclusions. Missing data can significantly skew results, making them unreliable.
3. Inaccurate Data
Inaccurate data is simply incorrect information. This could stem from typos, human error during data entry, outdated information, or faulty data collection methods. For instance, a customer's birthdate might be entered incorrectly, or their address might be outdated.
Impact: Inaccurate data leads to unreliable reports, flawed analysis, and poor decision-making. It can damage a company's reputation and lead to legal or financial repercussions. Decisions based on incorrect information can have significant, and potentially costly, consequences.
4. Duplicate Data
Duplicate data refers to the same data appearing multiple times within a database or across different systems. This redundancy can significantly inflate the dataset's size, slowing down processing speeds and consuming valuable storage space.
Impact: Duplicate data makes it challenging to gain a clear and accurate understanding of the data. It inflates the apparent size of the customer base, leading to inaccurate market analysis and potentially inefficient marketing campaigns. Data cleansing becomes more complex and time-consuming.
5. Invalid Data
Invalid data refers to information that does not conform to predefined data types or constraints. This might involve incorrect data formats, out-of-range values, or data that violates business rules. For example, entering text into a numerical field or entering a date in an incorrect format.
Impact: Invalid data can cause errors in data processing and analysis, leading to inaccurate reports and potentially system crashes. It necessitates robust validation mechanisms and error handling processes to ensure data integrity.
6. Outdated Data
Outdated data refers to information that is no longer current or relevant. In rapidly changing business environments, data can quickly become obsolete, impacting decision-making. For example, using last year's sales figures to predict this year's sales would likely be highly inaccurate.
Impact: Outdated data leads to poor forecasting, inefficient resource allocation, and a lack of responsiveness to market changes. Real-time data and regular data refreshes are essential to mitigate this problem.
7. Unstructured Data
Unstructured data refers to information that doesn't have a predefined format or organization. This includes text documents, images, audio files, and social media posts. While this type of data is often valuable, its unstructured nature makes it challenging to process and analyze effectively.
Impact: Unstructured data requires advanced techniques to extract meaningful insights. The lack of structure makes it difficult to integrate into existing systems and utilize for reporting or analysis without significant preprocessing and cleaning.
The Exception: Data Security Breaches
While all the problems above are crucial to address for maintaining robust data quality, data security breaches are different. They are not directly a problem of data quality, but rather a threat to data quality (and much more).
A data security breach, such as a hacking incident or unauthorized access, compromises the confidentiality, integrity, and availability of data. While the resulting data might be incomplete, inaccurate, or even deleted as a consequence of the breach, the core problem isn't inherent to the data's quality before the breach. The problem lies in the external threat and vulnerability of the system. Data quality issues often result from breaches, but are not synonymous with them.
A data security breach impacts data quality indirectly:
- Data Corruption: Hackers might intentionally corrupt or modify data, rendering it inaccurate and unreliable.
- Data Deletion: Data might be deleted or lost entirely during a breach.
- Data Exposure: Sensitive data might be exposed to unauthorized individuals, compromising privacy and potentially leading to legal issues.
However, the root cause of these issues isn't a data quality problem; it's a security vulnerability. Addressing data security breaches requires a different approach – focusing on security protocols, access controls, encryption, and disaster recovery planning – rather than data cleansing and validation techniques.
Proactive Measures for Data Quality Improvement
Maintaining high data quality requires a proactive approach. Here are some crucial steps organizations can take:
- Data Governance: Implement a robust data governance framework to define data standards, roles, responsibilities, and processes for data management.
- Data Profiling: Analyze data to identify patterns, inconsistencies, and anomalies. This helps understand the existing data quality issues and plan for remediation.
- Data Cleansing: Develop processes to clean and correct inaccurate, incomplete, or inconsistent data. This might involve manual review, automated rules, or machine learning algorithms.
- Data Validation: Implement data validation rules to prevent errors during data entry and updates. This ensures data conforms to defined standards and constraints.
- Data Integration: Integrate data from various sources to create a single, unified view of the information. This minimizes inconsistencies and redundancies.
- Data Monitoring: Continuously monitor data quality to identify and address issues proactively. Establish key performance indicators (KPIs) to track progress and measure improvements.
- Master Data Management (MDM): Establish a centralized repository for critical data elements (e.g., customer information, product details) to ensure consistency and accuracy across the organization.
- Data Quality Tools: Leverage specialized data quality tools to automate various tasks such as data profiling, cleansing, and validation.
- Employee Training: Educate employees on the importance of data quality and best practices for data entry and handling.
Conclusion
Data quality is critical for informed decision-making, efficient operations, and a competitive advantage. Many organizations struggle with several common data quality problems like inconsistency, incompleteness, inaccuracy, duplication, and invalid or outdated data. While data security breaches can significantly impact data quality, the root cause is a security vulnerability rather than an inherent data quality flaw. By implementing proactive strategies for data governance, profiling, cleansing, validation, integration, and monitoring, organizations can significantly enhance data quality, minimize risks, and unlock the full potential of their data. Remember, investing in data quality is investing in the future of your business.
Latest Posts
Latest Posts
-
Complete The Following Statement A Systems Approach To Decision Making
May 09, 2025
-
Moral Courage And Intelligent Disobedience Ted Thomas
May 09, 2025
-
Draw All Products Including Stereoisomers In The Following Reaction
May 09, 2025
-
What Is Thought To Lie At The Center Of Jupiter
May 09, 2025
-
Summary Of Chapter 9 Catcher In The Rye
May 09, 2025
Related Post
Thank you for visiting our website which covers about Common Data Quality Problems Include All Of The Following Except . We hope the information provided has been useful to you. Feel free to contact us if you have any questions or need further assistance. See you next time and don't miss to bookmark.