Data Analysis And Graphing Lab Answers
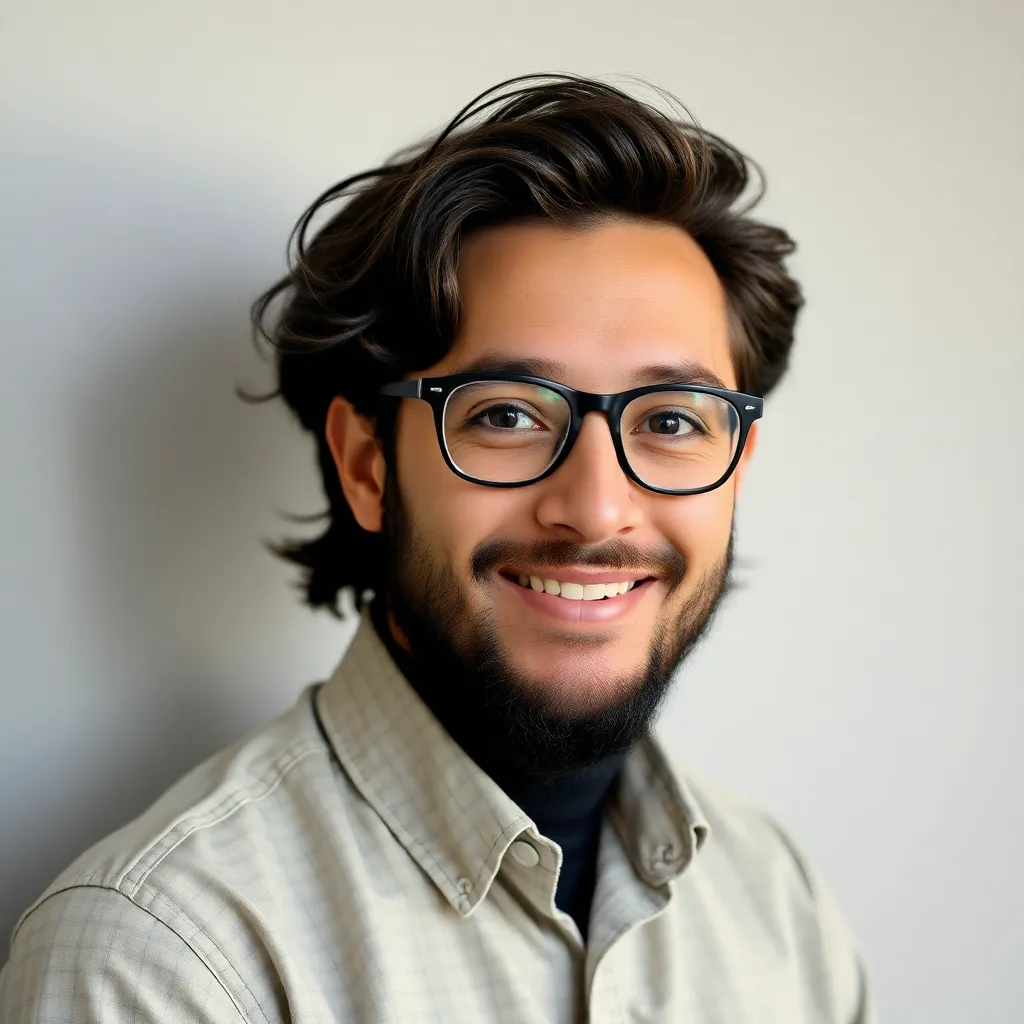
Onlines
Apr 16, 2025 · 6 min read

Table of Contents
Data Analysis and Graphing Lab Answers: A Comprehensive Guide
Data analysis and graphing are fundamental skills in numerous scientific disciplines. This comprehensive guide provides detailed explanations and answers to common questions encountered in data analysis and graphing labs. We'll explore various statistical methods, graphing techniques, and interpretation strategies to help you master these crucial skills.
Understanding Your Data: The Foundation of Analysis
Before diving into complex calculations and visualizations, it's crucial to thoroughly understand your data. This involves several key steps:
1. Data Collection and Organization
The quality of your analysis hinges on the quality of your data. Ensure your data collection methods are reliable and consistent. Organize your data into tables, using clear and concise labels for each variable. Consider using spreadsheets (like Excel or Google Sheets) for efficient data management.
2. Identifying Variables
Clearly distinguish between independent and dependent variables. The independent variable is the one you manipulate or control (e.g., temperature, time), while the dependent variable is the one you measure (e.g., plant growth, reaction rate). Proper identification of variables is critical for accurate interpretation.
3. Data Cleaning
Before any analysis, clean your data. This includes:
- Identifying and removing outliers: Outliers are data points significantly different from the rest. They can skew results. Investigate outliers; they may be errors, requiring correction or removal.
- Handling missing data: Missing data can impact your analysis. Decide on appropriate strategies: removal of incomplete data sets, imputation (estimating missing values based on available data), or using statistical techniques designed for incomplete datasets.
- Checking for data entry errors: Carefully review your data for typos or inconsistencies. Double-checking is essential for accuracy.
Descriptive Statistics: Summarizing Your Data
Descriptive statistics provide a concise summary of your data. Common descriptive statistics include:
1. Measures of Central Tendency
- Mean: The average of all data points. Sensitive to outliers.
- Median: The middle value when data is ordered. Less sensitive to outliers than the mean.
- Mode: The most frequent value.
Example: Consider a dataset of test scores: {70, 80, 85, 90, 95}. The mean is 84, the median is 85, and the mode doesn't exist in this case (all values appear only once).
2. Measures of Dispersion
- Range: The difference between the highest and lowest values. Simple but sensitive to outliers.
- Variance: The average of the squared differences from the mean. Measures the spread of data around the mean.
- Standard Deviation: The square root of the variance. A more interpretable measure of dispersion than variance, expressed in the same units as the data.
Example: For the test scores above, the range is 25 (95-70), the variance is approximately 100, and the standard deviation is approximately 10.
3. Frequency Distributions and Histograms
Frequency distributions show the number of times each value or range of values occurs. Histograms are visual representations of frequency distributions, providing a graphical summary of data distribution. Histograms help identify patterns such as skewness (asymmetry) and modality (number of peaks).
Inferential Statistics: Drawing Conclusions
Inferential statistics involve using sample data to make inferences about a larger population. This typically involves hypothesis testing.
1. Hypothesis Testing
A hypothesis is a testable statement about a population. Hypothesis testing involves:
- Stating a null hypothesis (H0): This is the statement you want to disprove.
- Stating an alternative hypothesis (H1 or Ha): This is the statement you want to support.
- Choosing a significance level (α): This is the probability of rejecting the null hypothesis when it's actually true (Type I error). Common values are 0.05 or 0.01.
- Performing a statistical test: The choice of test depends on the type of data and research question (e.g., t-test, ANOVA, chi-squared test).
- Interpreting the results: Based on the p-value (probability of obtaining the observed results if the null hypothesis is true), you either reject or fail to reject the null hypothesis. If the p-value is less than α, you reject the null hypothesis.
2. Common Statistical Tests
- t-test: Compares the means of two groups.
- ANOVA (Analysis of Variance): Compares the means of three or more groups.
- Chi-squared test: Tests for association between categorical variables.
- Correlation: Measures the strength and direction of the linear relationship between two variables. Correlation does not imply causation.
- Regression: Models the relationship between a dependent variable and one or more independent variables. Linear regression models a linear relationship, while other regression models can account for non-linear relationships.
Graphing Your Data: Visualizing Insights
Effective visualization is crucial for communicating your findings. Different graph types are suitable for different types of data and research questions.
1. Bar Charts
Suitable for comparing categorical data. Each bar represents a category, and the bar height represents the frequency or value for that category.
2. Histograms
Suitable for showing the distribution of numerical data. The x-axis represents data values or ranges, and the y-axis represents frequency.
3. Line Graphs
Suitable for showing trends over time or across continuous variables. Useful for visualizing relationships between two continuous variables.
4. Scatter Plots
Suitable for showing the relationship between two continuous variables. Each point represents a data point, with its x and y coordinates representing the values of the two variables. Useful for identifying correlations.
5. Pie Charts
Suitable for showing the proportion of different categories within a whole.
Interpreting Your Results: Drawing Meaningful Conclusions
Once you've analyzed your data and created your graphs, carefully interpret your results. Consider the following:
- Context: Interpret your findings within the context of your research question and experimental design.
- Limitations: Acknowledge any limitations of your study, such as sample size, data collection methods, or potential biases.
- Errors: Discuss potential sources of error, both systematic (consistent errors) and random (unpredictable errors).
- Implications: Discuss the implications of your findings, and suggest areas for future research.
Example Lab Scenario and Answers
Let's consider a hypothetical lab investigating the effect of different fertilizers on plant growth.
Scenario: Three different fertilizers (A, B, C) are applied to three groups of plants. Plant height is measured after four weeks. The data are as follows:
Fertilizer A: {15, 17, 16, 18, 19} cm Fertilizer B: {12, 10, 11, 13, 14} cm Fertilizer C: {20, 22, 21, 19, 23} cm
Analysis and Answers:
-
Descriptive Statistics: Calculate the mean, median, standard deviation for each fertilizer group. Create a table summarizing these statistics.
-
Graphing: Create a bar chart comparing the mean plant height for each fertilizer group. This visually represents the effect of different fertilizers on plant growth.
-
Inferential Statistics: Perform an ANOVA test to determine if there's a statistically significant difference in mean plant height among the three fertilizer groups. State your null and alternative hypotheses. Report your p-value and interpret the results. If the p-value is significant (e.g., less than 0.05), perform post-hoc tests (like Tukey's HSD) to determine which groups differ significantly from each other.
-
Interpretation: Based on your analysis, conclude whether the different fertilizers have a significant impact on plant growth. Discuss any limitations of the study, and suggest areas for further research (e.g., larger sample size, longer experimental period, consideration of other factors influencing plant growth).
This example demonstrates the process of data analysis and graphing. The specific calculations and statistical tests would be performed using statistical software or a calculator. Remember to always clearly present your data, methods, results, and interpretations in a concise and well-organized manner.
This guide provides a comprehensive overview of data analysis and graphing techniques. By understanding these concepts and applying appropriate methods, you can effectively analyze your data, draw meaningful conclusions, and communicate your findings clearly and effectively. Remember to consult relevant textbooks and resources for more in-depth explanations and specific applications. Consistent practice is key to mastering these valuable skills.
Latest Posts
Latest Posts
-
The Optimal Capital Structure Has Been Achieved When The
Apr 17, 2025
-
Double Stuff Oreo Lab Answer Key Pdf
Apr 17, 2025
-
What Type Of Reaction Steps Are Represented Below
Apr 17, 2025
-
Bohr Model Of Hydrogen Gizmo Answers
Apr 17, 2025
-
Me Aburren Las Matematicas Bore Please Bother Enchant
Apr 17, 2025
Related Post
Thank you for visiting our website which covers about Data Analysis And Graphing Lab Answers . We hope the information provided has been useful to you. Feel free to contact us if you have any questions or need further assistance. See you next time and don't miss to bookmark.