Data Cannot Be Used To Disaggregate Problems.
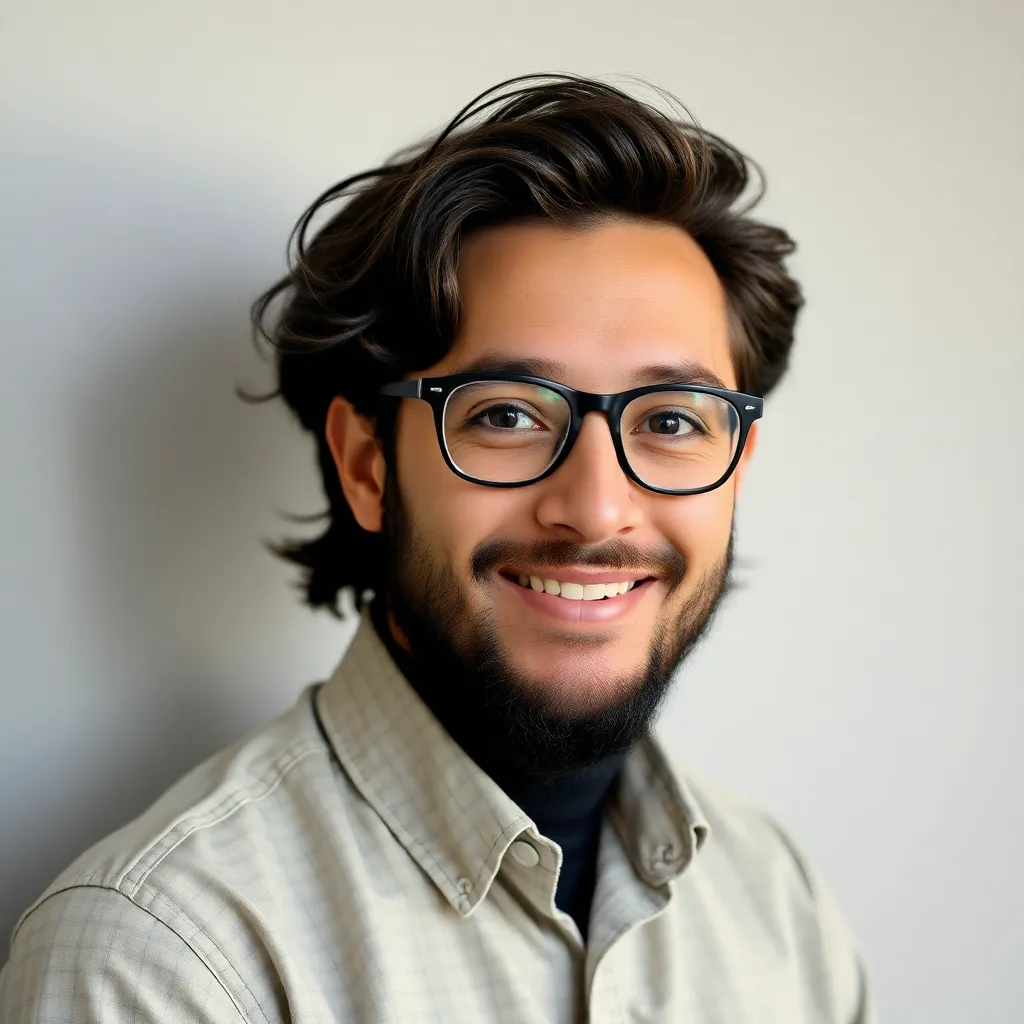
Onlines
May 05, 2025 · 5 min read

Table of Contents
Data Cannot Be Used to Disaggregate Problems: A Critical Examination
The prevalent belief that data can solve all our problems, particularly through disaggregation, is a seductive yet ultimately flawed assumption. While data is undeniably a powerful tool for understanding complex issues, its inherent limitations prevent it from serving as the sole solution for disaggregating and subsequently addressing multifaceted societal challenges. This article delves into the critical reasons why relying solely on data disaggregation for problem-solving is insufficient and even potentially misleading. We'll explore the conceptual limitations of data, the ethical considerations involved, and the crucial role of human interpretation and context in tackling complex societal problems.
The Illusion of Data-Driven Disaggregation: A Closer Look
The idea behind data disaggregation is straightforward: by breaking down large datasets into smaller, more specific subgroups, we can identify unique patterns and disparities within a population. This allows for a more nuanced understanding of the problem, leading to targeted interventions and potentially more effective solutions. For example, disaggregating crime data by race, gender, and socioeconomic status might reveal disparities in policing practices or uncover underlying social factors contributing to crime rates within specific communities.
However, this seemingly straightforward process is riddled with complexities. The effectiveness of data disaggregation hinges on several crucial factors that are often overlooked:
1. Data Quality and Representativeness: The Foundation of Flawed Analysis
Garbage in, garbage out remains a fundamental principle in data analysis. If the initial data is biased, incomplete, or inaccurate, any subsequent disaggregation will inherit and amplify these flaws. This can lead to misleading conclusions and potentially harmful interventions. For instance, if a survey used to collect data on healthcare access excludes a significant portion of the population due to language barriers or lack of internet access, the resulting disaggregation will inevitably present a skewed picture of healthcare disparities.
2. The Problem of Causation vs. Correlation: Unveiling the Underlying Dynamics
Disaggregated data might reveal correlations between variables, but it rarely provides definitive evidence of causation. Discovering a correlation between poverty and crime rates within a specific demographic group doesn't automatically imply that poverty causes crime. Other factors, such as lack of educational opportunities, systemic discrimination, or historical trauma, might play a more significant role. Confounding variables, often hidden within complex datasets, can easily distort the true relationship between variables. This necessitates a multi-faceted approach extending beyond simple data analysis.
3. Ignoring the Contextual Nuances: Beyond Numbers and Statistics
Data, by its nature, is quantitative. It represents numerical values and statistical patterns, but it often fails to capture the rich tapestry of human experiences and contextual factors that shape social problems. Disaggregating data on unemployment, for instance, might reveal disparities between different age groups. However, this analysis alone cannot capture the unique challenges faced by young adults entering a competitive job market or the struggles of older workers facing age discrimination. Understanding the lived experiences requires qualitative research, narratives, and on-the-ground insights that data alone cannot provide.
The Ethical Implications of Data Disaggregation: Potential for Harm
The ethical considerations associated with data disaggregation are substantial. While the goal is often to improve equity and address social injustices, the process itself can inadvertently perpetuate harmful biases or lead to unintended consequences:
1. Reinforcing Existing Stereotypes and Biases: The Perils of Reductionism
Disaggregating data by race, ethnicity, or gender can inadvertently reinforce existing stereotypes and biases if not carefully interpreted and contextualized. Focusing solely on group averages can obscure the diversity within each group and mask the unique experiences of individuals. This can lead to the creation of overly simplistic and potentially harmful narratives that reinforce discriminatory practices.
2. Data Privacy and Surveillance Concerns: Balancing Utility and Rights
Collecting and disaggregating sensitive personal data raises significant privacy concerns. The potential for misuse or unauthorized access to this information demands robust data protection mechanisms and ethical considerations throughout the data lifecycle. The balance between using data to address social problems and protecting individual privacy requires careful consideration and thoughtful policy frameworks.
3. The Risk of Stigmatization and Marginalization: Unintended Consequences
The results of data disaggregation can be used to justify discriminatory practices or further marginalize already vulnerable populations. For instance, disaggregated data showing higher crime rates in certain neighborhoods might lead to increased police surveillance and disproportionate targeting of those communities, exacerbating existing inequalities. This highlights the critical need for responsible data usage and the ethical framing of results.
Beyond Data Disaggregation: A Holistic Approach to Problem-Solving
While data disaggregation can be a valuable tool for informing policy and interventions, it should never be the sole basis for addressing complex social problems. A truly effective approach requires a multi-faceted strategy that integrates:
1. Qualitative Research and Community Engagement: Gathering Diverse Perspectives
Qualitative research methods, such as interviews, focus groups, and ethnographic studies, provide rich contextual information that complements quantitative data. Engaging directly with affected communities is essential for understanding their lived experiences and ensuring that solutions are relevant and culturally sensitive.
2. Interdisciplinary Collaboration: Leveraging Expertise from Diverse Fields
Addressing complex social issues requires the expertise of individuals from various disciplines. Collaboration between data scientists, social scientists, policymakers, community leaders, and affected individuals is crucial for developing comprehensive and effective strategies.
3. Policy Design and Implementation: Translating Insights into Action
Data insights are only useful if they inform effective policies and interventions. The process of translating data-driven insights into actionable policies requires careful consideration of resource allocation, implementation strategies, and ongoing evaluation.
4. Continuous Monitoring and Evaluation: Ensuring Accountability and Adaptability
Any intervention should be subject to ongoing monitoring and evaluation to assess its effectiveness and identify areas for improvement. This iterative process allows for adjustments based on real-world outcomes, ensuring that solutions remain responsive to evolving needs.
Conclusion: Data as a Tool, Not a Solution
Data disaggregation can be a valuable tool for understanding and addressing societal problems, but it is not a panacea. Its limitations, ethical implications, and the crucial role of human interpretation and context demand a more holistic and nuanced approach. Over-reliance on data disaggregation risks perpetuating biases, overlooking important contextual factors, and ultimately failing to address the root causes of complex social issues. A truly effective strategy requires a multi-faceted approach that integrates quantitative data analysis with qualitative research, community engagement, and interdisciplinary collaboration. Only then can we hope to achieve meaningful and sustainable solutions to the challenges facing our world.
Latest Posts
Latest Posts
-
The Three Competitive Factors That Impact Only
May 06, 2025
-
Which Of The Following Statements Regarding Carbon Is False
May 06, 2025
-
Bumpy Structure Under The Stomach Makes Insulin
May 06, 2025
-
Advance Study Assignment Identification Of A Compound By Mass Relationships
May 06, 2025
-
The Cask Of Amontillado One Pager
May 06, 2025
Related Post
Thank you for visiting our website which covers about Data Cannot Be Used To Disaggregate Problems. . We hope the information provided has been useful to you. Feel free to contact us if you have any questions or need further assistance. See you next time and don't miss to bookmark.