Digital Information Processed Into A Useful Form Is Called
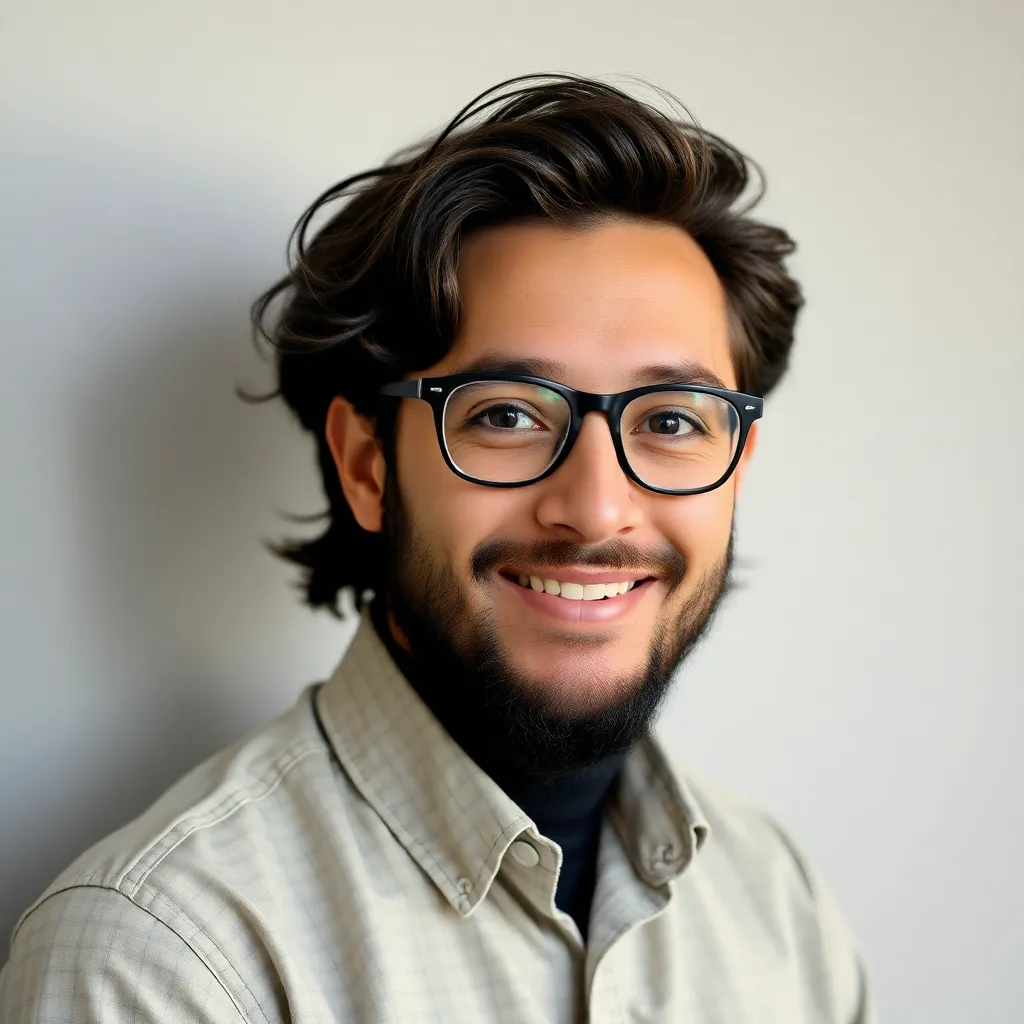
Onlines
May 10, 2025 · 6 min read
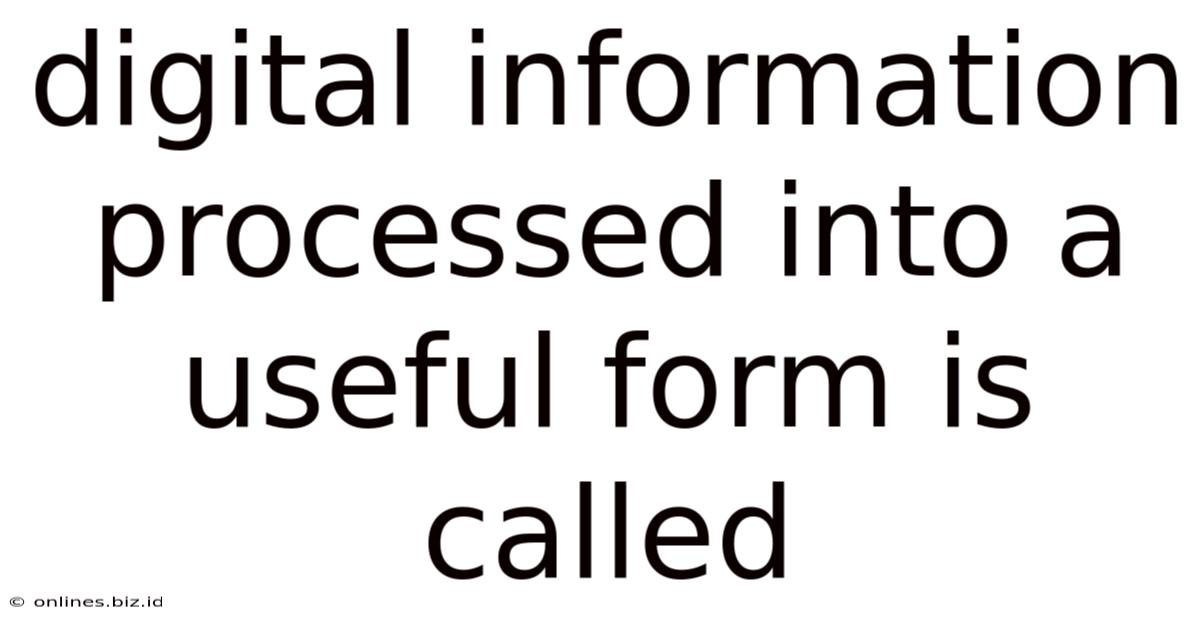
Table of Contents
Digital Information Processed into a Useful Form: Understanding Data Transformation
The phrase "digital information processed into a useful form" encompasses a vast and crucial area of modern computing and data science. While there isn't one single, universally accepted term to define this process, it can be described as data transformation, data processing, information extraction, knowledge discovery, or even data analysis, depending on the context and the ultimate goal. This article delves deep into the various aspects of this transformation, exploring its methods, applications, and the crucial role it plays in our increasingly data-driven world.
What is Digital Information Processing?
At its core, digital information processing involves taking raw digital data – a seemingly chaotic collection of bits and bytes – and converting it into a structured, meaningful, and usable format. This process is essential because raw data, in its unprocessed state, is often incomprehensible and incapable of providing valuable insights. Think of it like mining for gold: the raw ore contains gold, but it requires significant processing to extract and refine it into a valuable form.
Digital information processing leverages various techniques and tools to achieve this transformation. These techniques can be broadly categorized into:
1. Data Cleaning and Preprocessing: Laying the Foundation
Before any meaningful analysis can occur, the data must be cleaned and preprocessed. This vital step involves:
- Handling Missing Values: Identifying and addressing missing data points through techniques like imputation (filling in missing values based on existing data) or removal of incomplete records.
- Outlier Detection and Treatment: Identifying and managing outliers – data points significantly different from the rest – which can skew results. Methods include removal, transformation, or capping.
- Data Transformation: Converting data into a suitable format for analysis. This might involve scaling numerical data, converting categorical data into numerical representations (e.g., one-hot encoding), or handling date and time formats.
- Data Reduction: Reducing the size of the dataset while preserving important information. Techniques include dimensionality reduction (e.g., Principal Component Analysis) and feature selection.
- Data Integration: Combining data from multiple sources, ensuring consistency and accuracy. This is crucial in scenarios with data spread across different databases or systems.
2. Data Analysis and Interpretation: Unveiling the Insights
Once the data is cleaned and preprocessed, the actual analysis begins. Various techniques are used, depending on the type of data and the research questions:
- Descriptive Statistics: Summarizing data using measures like mean, median, mode, standard deviation, and percentiles. This provides a basic understanding of the data's distribution and central tendency.
- Inferential Statistics: Drawing conclusions about a population based on a sample of data. This includes hypothesis testing and confidence intervals.
- Regression Analysis: Modeling the relationship between a dependent variable and one or more independent variables. Used for prediction and understanding causal relationships.
- Classification: Categorizing data into predefined classes. Techniques include decision trees, support vector machines, and neural networks.
- Clustering: Grouping similar data points together based on their characteristics. Useful for identifying patterns and segmenting data.
- Data Visualization: Presenting data in a visually appealing and informative manner using charts, graphs, and dashboards. This makes complex data easier to understand and communicate.
3. Knowledge Representation and Reasoning: Building Understanding
The ultimate goal of digital information processing often involves extracting knowledge and building understanding from the data. This can involve:
- Knowledge Graphs: Representing information as interconnected nodes and edges, allowing for complex reasoning and querying.
- Semantic Web Technologies: Using ontologies and semantic annotations to provide richer meaning and context to data.
- Machine Learning (ML) and Artificial Intelligence (AI): Leveraging algorithms to automatically learn patterns and make predictions from data. This allows for automation of tasks and the discovery of complex relationships.
- Natural Language Processing (NLP): Analyzing and understanding text data to extract meaning and sentiment. This is crucial for processing unstructured textual information.
Applications of Digital Information Processing: Transforming Industries
The impact of digital information processing is far-reaching, transforming numerous industries and aspects of our daily lives. Here are some key examples:
1. Business and Finance: Data-Driven Decision Making
Businesses utilize digital information processing for:
- Customer Relationship Management (CRM): Analyzing customer data to understand preferences, predict behavior, and personalize marketing efforts.
- Supply Chain Optimization: Analyzing supply chain data to improve efficiency, reduce costs, and enhance responsiveness.
- Fraud Detection: Identifying fraudulent transactions by analyzing patterns and anomalies in financial data.
- Risk Management: Assessing and managing various types of risks through data analysis and predictive modeling.
2. Healthcare: Improving Patient Care and Research
In healthcare, digital information processing is crucial for:
- Disease Diagnosis and Treatment: Analyzing medical images and patient data to aid in diagnosis and personalized treatment plans.
- Drug Discovery and Development: Using data analysis to accelerate drug discovery and development processes.
- Public Health Surveillance: Monitoring disease outbreaks and trends to inform public health interventions.
- Personalized Medicine: Tailoring treatment plans to individual patients based on their genetic and clinical data.
3. Science and Engineering: Advancing Knowledge and Innovation
Scientific research relies heavily on digital information processing for:
- Genomics and Proteomics: Analyzing large datasets of genomic and proteomic data to understand biological processes and develop new therapies.
- Climate Modeling: Analyzing climate data to predict future climate change scenarios and develop mitigation strategies.
- Material Science: Analyzing material properties to develop new materials with improved characteristics.
- Astronomy: Analyzing astronomical data to understand the universe and discover new celestial objects.
4. Social Sciences: Understanding Human Behavior and Societies
Social scientists utilize digital information processing to:
- Social Network Analysis: Analyzing social networks to understand social interactions and influence.
- Opinion Mining and Sentiment Analysis: Analyzing text data to understand public opinion and sentiment.
- Urban Planning: Analyzing city data to optimize urban planning and resource allocation.
- Political Science: Analyzing political data to understand voting patterns and political trends.
Challenges and Ethical Considerations
While digital information processing offers tremendous benefits, it also presents several challenges:
- Data Security and Privacy: Protecting sensitive data from unauthorized access and misuse is paramount. Robust security measures and ethical data handling practices are crucial.
- Data Bias and Fairness: Data can reflect existing societal biases, leading to unfair or discriminatory outcomes. Careful attention must be paid to mitigate bias in data and algorithms.
- Data Quality and Reliability: The accuracy and reliability of the results depend heavily on the quality of the input data. Data cleaning and validation are essential steps.
- Computational Complexity: Processing large datasets can be computationally expensive and time-consuming. Efficient algorithms and computational resources are needed.
- Explainability and Interpretability: Understanding how complex algorithms arrive at their conclusions is crucial for building trust and ensuring accountability.
The Future of Digital Information Processing
The field of digital information processing is constantly evolving, with new techniques and technologies emerging regularly. Key trends include:
- Big Data Analytics: Handling and analyzing massive datasets using distributed computing and advanced analytics techniques.
- Artificial Intelligence and Machine Learning: Increasing automation and intelligence in data processing tasks.
- Edge Computing: Processing data closer to the source, reducing latency and bandwidth requirements.
- Quantum Computing: Potentially revolutionizing data processing with its ability to solve complex problems that are intractable for classical computers.
In conclusion, digital information processed into a useful form – whether called data transformation, data analysis, or knowledge discovery – is the foundation of a data-driven world. Its applications are vast and continue to expand, impacting every aspect of our lives. However, addressing the ethical and practical challenges associated with this process is crucial to ensuring its responsible and beneficial use. By understanding the intricacies of digital information processing and employing best practices, we can unlock its immense potential for progress and innovation.
Latest Posts
Latest Posts
-
Which Option Uses Dialogue Most Effectively
May 10, 2025
-
How Is The Word Pl Ay Divided In This Example
May 10, 2025
-
Identity By Julio Noboa Polanco Answer Key
May 10, 2025
-
Suppose A Pigeon That Is Homozygous For The Grouse Allele
May 10, 2025
-
Espresso Express Operates A Number Of
May 10, 2025
Related Post
Thank you for visiting our website which covers about Digital Information Processed Into A Useful Form Is Called . We hope the information provided has been useful to you. Feel free to contact us if you have any questions or need further assistance. See you next time and don't miss to bookmark.