Elementary Statistics A Step By Step Approach 10th Edition Pdf
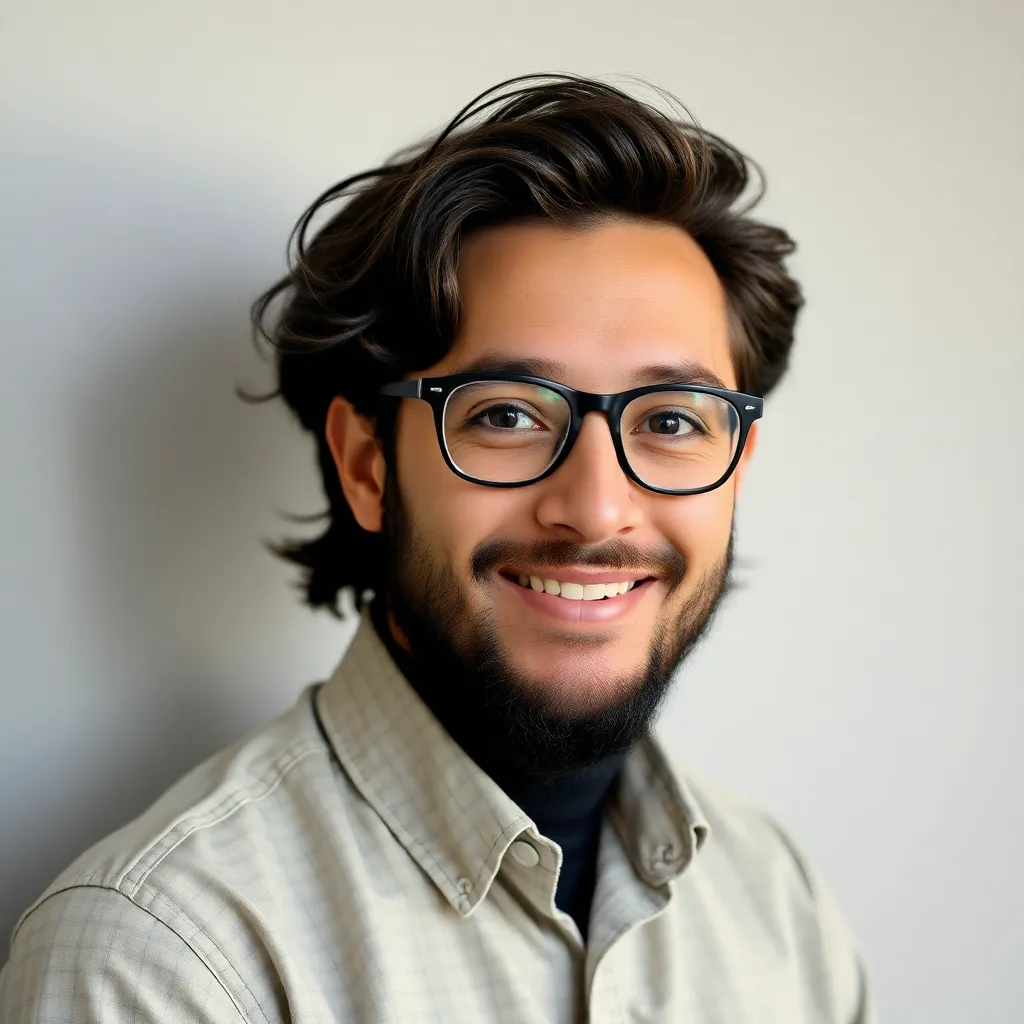
Onlines
May 08, 2025 · 7 min read
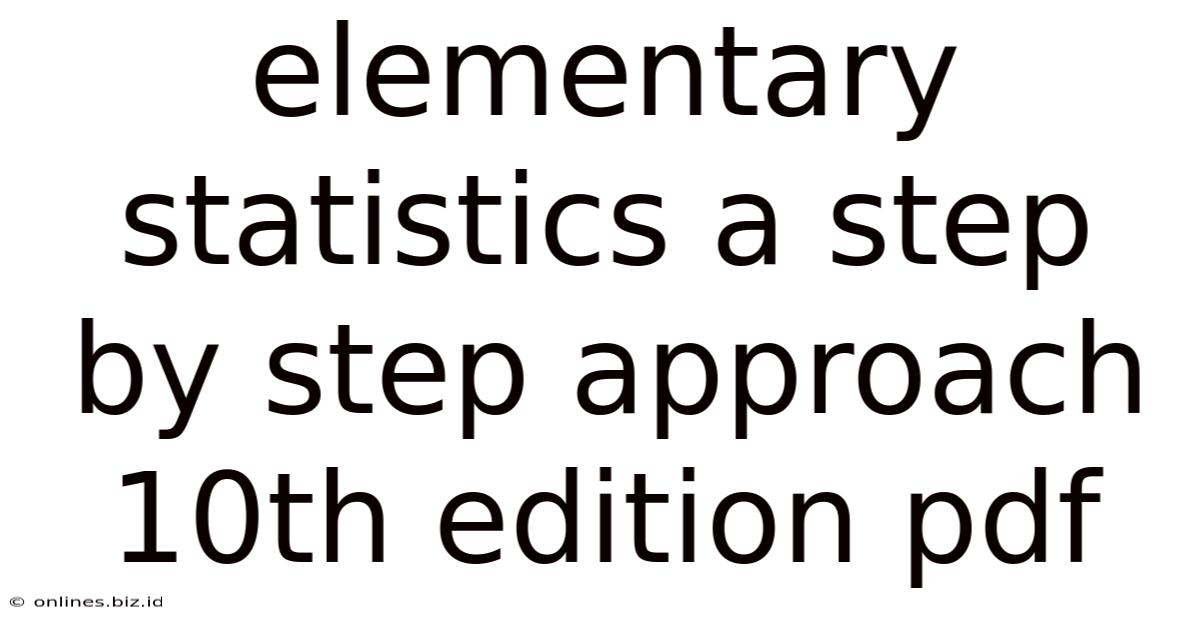
Table of Contents
Elementary Statistics: A Step-by-Step Approach, 10th Edition - A Comprehensive Guide
Finding the right resources for learning elementary statistics can be challenging. Many students struggle with the abstract concepts and complex calculations involved. This guide aims to provide a detailed overview of the content typically covered in an elementary statistics course, using Elementary Statistics: A Step-by-Step Approach, 10th Edition as a reference point. While we won't provide a PDF of the textbook, we'll delve into the key concepts, offering explanations and examples to enhance your understanding.
This article will cover the core topics typically found in such a textbook, broken down into manageable sections for easier comprehension. Remember, consistent practice and problem-solving are crucial for mastering statistics.
I. Descriptive Statistics: Summarizing and Presenting Data
This foundational section deals with methods to organize, summarize, and present data in a meaningful way. Key concepts include:
1. Organizing and Graphing Data
-
Frequency Distributions: These tables summarize the number of times each value (or range of values) occurs in a dataset. Histograms, frequency polygons, and ogives visually represent these distributions. Understanding the shape of the distribution (symmetrical, skewed, etc.) provides valuable insights.
-
Stem-and-Leaf Plots: A less common but effective way to display data, especially for smaller datasets, showing both the frequency and the actual data values.
-
Pie Charts and Bar Graphs: Excellent for categorical data, showing proportions or frequencies of different categories. These are visually appealing and easily interpretable for non-statistical audiences.
2. Measures of Central Tendency
These statistics describe the "center" of a dataset. The three most common are:
-
Mean: The average of all values. Easily calculated but sensitive to outliers (extreme values).
-
Median: The middle value when the data is ordered. Less sensitive to outliers than the mean.
-
Mode: The value that occurs most frequently. Can be used for both numerical and categorical data. A dataset can have multiple modes or no mode at all.
Choosing the appropriate measure depends on the data's distribution and the research question.
3. Measures of Dispersion (Variability)
These statistics describe the spread or variability of the data. Understanding dispersion is crucial because it provides context for measures of central tendency.
-
Range: The difference between the largest and smallest values. Simple to calculate but heavily influenced by outliers.
-
Variance: The average of the squared deviations from the mean. It measures the average squared distance of each data point from the mean.
-
Standard Deviation: The square root of the variance. Expressed in the same units as the original data, making it easier to interpret than the variance. It's a widely used measure of variability.
-
Interquartile Range (IQR): The difference between the third quartile (75th percentile) and the first quartile (25th percentile). A robust measure of spread, less sensitive to outliers than the range or standard deviation.
II. Probability and Probability Distributions
This section introduces the fundamental concepts of probability, essential for making inferences from data.
1. Basic Probability Concepts
-
Sample Space: The set of all possible outcomes of an experiment.
-
Event: A subset of the sample space.
-
Probability: The likelihood of an event occurring, ranging from 0 (impossible) to 1 (certain).
-
Types of Probability: Classical (equally likely outcomes), empirical (based on observed frequencies), subjective (based on personal belief).
-
Rules of Probability: Addition rule (for mutually exclusive and non-mutually exclusive events), multiplication rule (for independent and dependent events), conditional probability.
2. Discrete Probability Distributions
-
Binomial Distribution: Models the probability of a certain number of successes in a fixed number of independent trials, each with the same probability of success.
-
Poisson Distribution: Models the probability of a certain number of events occurring in a fixed interval of time or space, when the events are independent and occur at a constant average rate.
3. Continuous Probability Distributions
-
Normal Distribution: A bell-shaped, symmetrical distribution characterized by its mean (µ) and standard deviation (σ). Extremely important in statistics due to its frequent occurrence in natural phenomena and its use in many statistical tests. Understanding the empirical rule (68-95-99.7 rule) is vital for interpreting normal distributions.
-
Central Limit Theorem: A cornerstone of inferential statistics. It states that the sampling distribution of the mean of a large number of independent, identically distributed random variables will be approximately normally distributed, regardless of the shape of the original distribution. This is incredibly important for making inferences about population parameters based on sample data.
III. Inferential Statistics: Making Inferences about Populations
This section deals with drawing conclusions about populations based on sample data.
1. Sampling Distributions
-
Sampling Distribution of the Mean: The distribution of all possible sample means from a population. Its mean is equal to the population mean, and its standard deviation (standard error) is equal to the population standard deviation divided by the square root of the sample size.
-
Sampling Distribution of the Proportion: The distribution of all possible sample proportions from a population.
2. Estimation
-
Point Estimation: Using a sample statistic (e.g., sample mean) to estimate a population parameter (e.g., population mean).
-
Interval Estimation (Confidence Intervals): Providing a range of values within which the population parameter is likely to fall, with a certain level of confidence (e.g., 95% confidence interval). The width of the interval is influenced by the sample size, variability, and desired confidence level.
3. Hypothesis Testing
This is a crucial aspect of inferential statistics, involving testing claims about population parameters. The process generally involves:
-
Formulating Hypotheses: Stating the null hypothesis (H₀) and the alternative hypothesis (H₁ or Hₐ).
-
Selecting a Test Statistic: Choosing an appropriate test based on the type of data, hypotheses, and sample size. Common tests include the z-test, t-test, chi-square test, and ANOVA.
-
Determining the p-value: The probability of observing the obtained results (or more extreme results) if the null hypothesis is true. A small p-value (typically below a significance level, such as 0.05) leads to rejecting the null hypothesis.
-
Making a Decision: Based on the p-value and significance level, we decide whether to reject or fail to reject the null hypothesis.
-
Interpreting the Results: Clearly stating the conclusions in the context of the research question. Understanding Type I and Type II errors is crucial for accurate interpretation.
IV. Regression and Correlation Analysis
This section explores the relationship between two or more variables.
1. Correlation
-
Correlation Coefficient (r): Measures the strength and direction of a linear relationship between two variables. Ranges from -1 (perfect negative correlation) to +1 (perfect positive correlation). A value of 0 indicates no linear correlation.
-
Scatter Plots: Visual representations of the relationship between two variables.
2. Linear Regression
-
Linear Regression Equation: A mathematical model describing the linear relationship between a dependent variable (Y) and one or more independent variables (X). The equation is of the form Y = β₀ + β₁X + ε, where β₀ is the y-intercept, β₁ is the slope, and ε is the error term.
-
Least Squares Method: A method used to estimate the parameters (β₀ and β₁) of the linear regression equation that minimizes the sum of squared errors.
-
Coefficient of Determination (R²): Indicates the proportion of the variance in the dependent variable that is explained by the independent variable(s).
V. Other Important Topics
Depending on the specific textbook and course, other topics may be included, such as:
-
Analysis of Variance (ANOVA): Used to compare the means of three or more groups.
-
Chi-Square Tests: Used to analyze categorical data and test for independence between variables.
-
Non-parametric Tests: Statistical tests that do not assume any specific distribution for the data. Useful when the data doesn't meet the assumptions of parametric tests.
-
Sampling Techniques: Understanding different methods of sampling (simple random sampling, stratified sampling, cluster sampling) is essential for obtaining representative samples.
Conclusion:
Mastering elementary statistics requires consistent effort and practice. This guide provides a framework for understanding the key concepts covered in Elementary Statistics: A Step-by-Step Approach, 10th Edition, but remember that active learning through problem-solving and working through examples is crucial. Use this article as a stepping stone to further explore the fascinating world of statistics. Remember to consult additional resources, seek help when needed, and practice, practice, practice! Good luck!
Latest Posts
Latest Posts
-
What Is The Biggest Advantage Of Working With Reusable Datasets
May 08, 2025
-
Lauren Is Preparing A Presentation For Her Class
May 08, 2025
-
Green Mountain Coffee Roasters Statement Of Cash Flows
May 08, 2025
-
Find The Slope Of The Line Graphed Below Y12345 1 2 3 4 5x12345 1 2 3 4 5
May 08, 2025
-
Dantes Divine Comedy Depicts The Poets Mythical Journey Through
May 08, 2025
Related Post
Thank you for visiting our website which covers about Elementary Statistics A Step By Step Approach 10th Edition Pdf . We hope the information provided has been useful to you. Feel free to contact us if you have any questions or need further assistance. See you next time and don't miss to bookmark.