Explain How Redundant Data Often Leads To Poorer Decision Making
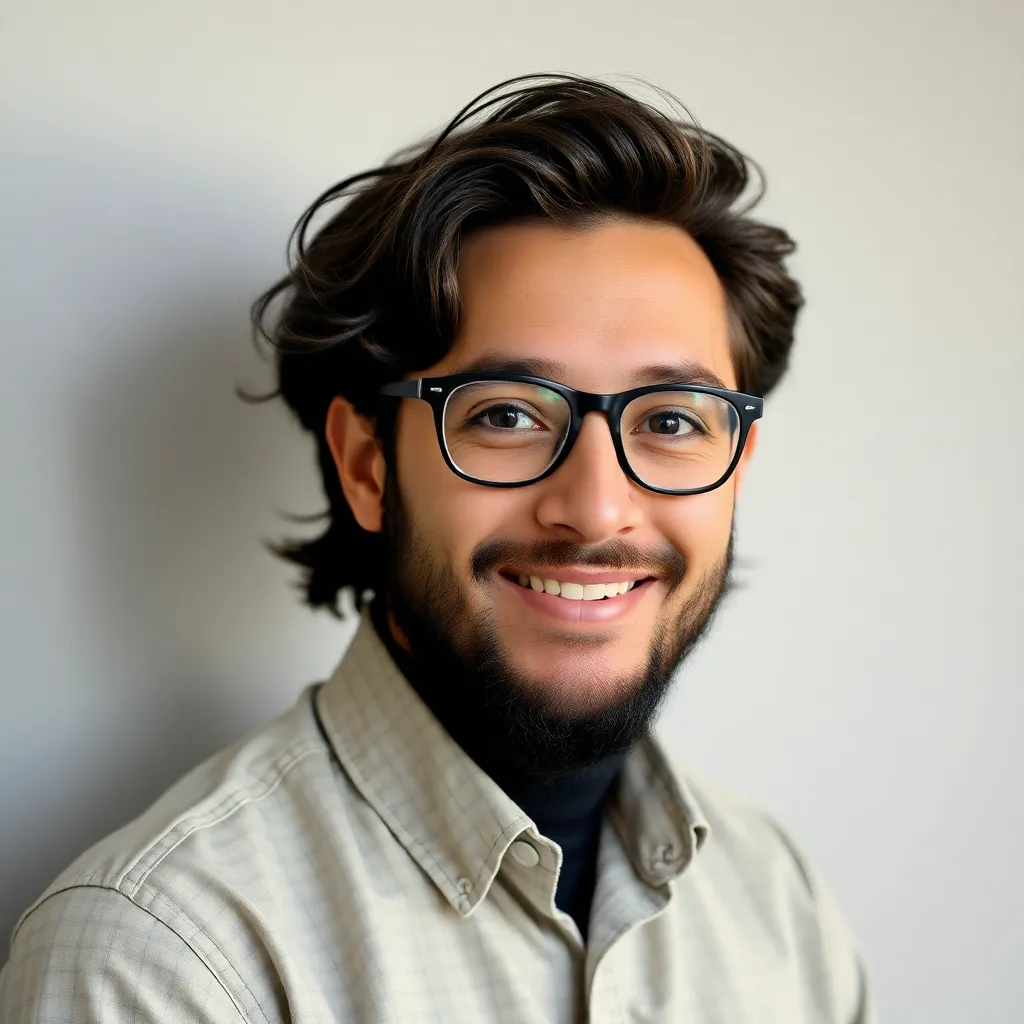
Onlines
May 12, 2025 · 6 min read
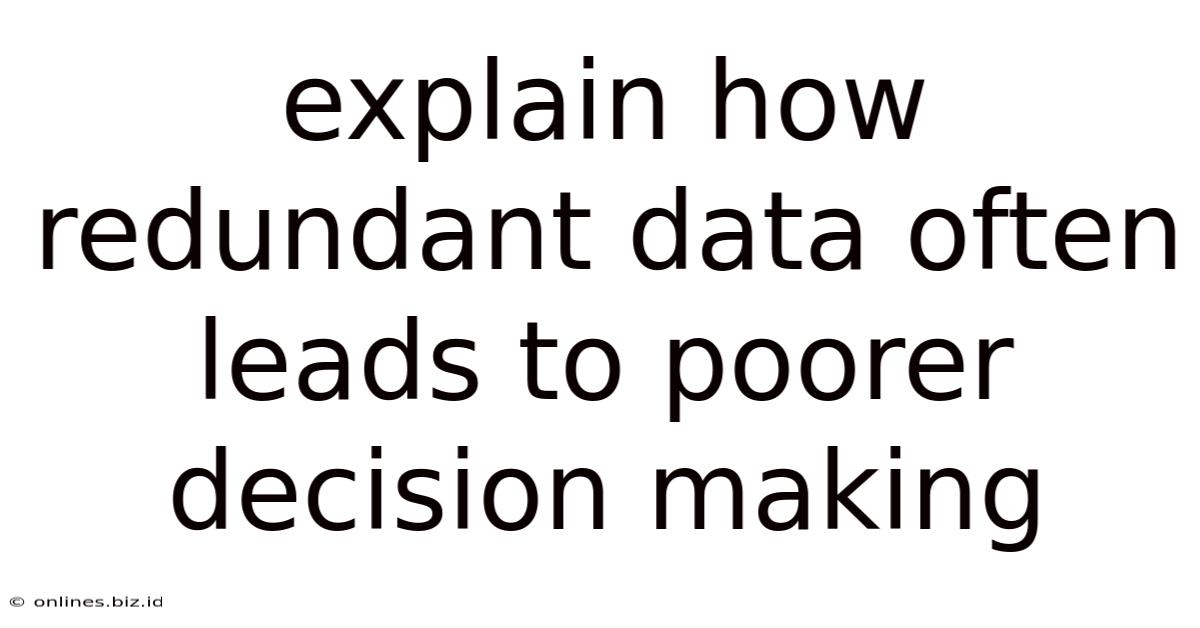
Table of Contents
How Redundant Data Often Leads to Poorer Decision-Making
In today's data-driven world, access to information is more prevalent than ever before. However, the sheer volume of data available doesn't automatically translate to better decision-making. In fact, redundant data, or data that is duplicated or unnecessarily repeated, can significantly hinder the decision-making process, leading to flawed conclusions and ultimately, poor outcomes. This article will delve into the various ways redundant data negatively impacts decision-making, exploring the underlying causes, consequences, and strategies for mitigation.
The Perils of Redundancy: How Duplicate Data Clouds Judgment
Redundant data presents a multi-faceted problem, impacting decision-making in several critical ways:
1. Data Inconsistency and Confusion: The Root of Poor Decisions
One of the most immediate consequences of redundant data is the creation of inconsistencies. Different versions of the same data may exist across various databases or spreadsheets, leading to confusion and uncertainty. Imagine trying to make a strategic business decision based on sales figures that vary wildly depending on the source. This discrepancy can lead to erroneous conclusions and ultimately, flawed strategies. The resulting inconsistencies erode trust in the data itself, making any decision based on it inherently suspect.
2. Increased Complexity and Reduced Efficiency: A Time-Wasting Nightmare
Dealing with redundant data dramatically increases the complexity of data analysis. Instead of focusing on extracting meaningful insights, analysts spend valuable time identifying and resolving inconsistencies, cleaning the data, and ensuring accuracy. This reduces efficiency and increases the overall cost and time associated with decision-making. The time wasted on data cleanup could have been better spent on strategic analysis and formulation of actionable plans.
3. Biased Analysis and Misinterpretation: A Recipe for Disaster
Redundant data can introduce bias into the analysis process. If certain data points are duplicated more frequently than others, they may disproportionately influence the results. This can lead to a skewed understanding of the situation, resulting in decisions based on incomplete or misleading information. For example, if a survey contains redundant responses from a particular demographic group, the analysis might overrepresent that group's preferences, leading to flawed conclusions about the overall population.
4. Increased Storage Costs and Resource Consumption: A Hidden Expense
Storing redundant data requires significant storage space and computational resources. This translates to increased IT costs, which can be a substantial burden for organizations, particularly those dealing with large datasets. The financial implications of managing redundant data often go unnoticed, yet they represent a significant hidden expense that could be avoided through better data management practices. These resources could have been allocated towards more productive tasks.
5. Delayed Decision-Making and Lost Opportunities: The Price of Inaction
The challenges posed by redundant data often lead to delays in decision-making. The process of identifying, resolving, and cleaning the data can take considerable time, delaying crucial actions and potentially missing valuable opportunities. In fast-paced environments, where timely decisions are crucial for success, the cost of delayed decision-making can be substantial, impacting profitability and competitiveness.
Unpacking the Sources of Redundancy: Identifying the Culprits
Understanding the sources of redundant data is crucial for implementing effective prevention strategies. Several factors contribute to the accumulation of redundant data within an organization:
1. Data Silos and Lack of Integration: The Fragmentation Problem
Data silos, or isolated data repositories that aren't integrated with each other, are a major contributor to data redundancy. Different departments or systems may maintain their own independent copies of the same data, resulting in inconsistencies and duplication. A lack of data integration leads to a fragmented view of information, making it difficult to obtain a holistic understanding of the situation.
2. Poor Data Governance and Lack of Standards: The Governance Gap
The absence of robust data governance policies and standards contributes to the proliferation of redundant data. Without clear guidelines for data management, individuals may create redundant copies of data without realizing the implications. This lack of standardization leads to inconsistencies in data format, naming conventions, and storage practices.
3. Manual Data Entry and Human Error: The Human Factor
Manual data entry is a significant source of redundancy, as human errors are inevitable. Individuals may accidentally create duplicate entries or fail to update data consistently across different systems. This highlights the importance of automating data entry processes as much as possible to reduce the likelihood of human error.
4. Inefficient Data Migration and Integration Processes: The Migration Mess
During data migration or integration projects, data redundancy can easily occur if proper precautions aren't taken. If data is not properly cleaned and de-duplicated before merging different datasets, redundancy issues can arise. Careful planning and execution are crucial to avoid this problem.
5. Lack of Data Quality Monitoring and Cleansing Processes: The Oversight Issue
A lack of data quality monitoring and cleansing processes allows redundant data to accumulate over time. Without regular checks and cleaning routines, inconsistencies and duplicates can go undetected, ultimately hindering the decision-making process. Implementing regular data quality checks is essential to identify and address redundancy issues before they escalate.
Mitigating the Impact: Strategies for Better Data Management
To effectively combat the negative effects of redundant data, organizations must implement strategies for better data management. Several key approaches can be employed:
1. Implementing Data Governance Frameworks: Establishing Clear Guidelines
Establishing a robust data governance framework is crucial. This framework should define clear guidelines for data management, including data quality standards, data ownership, and data access control. It should also establish processes for data cleansing and de-duplication.
2. Data Integration and Consolidation: Breaking Down Silos
Integrating and consolidating data from different sources is essential to eliminate redundancy. This involves establishing a central data repository that houses all relevant information, ensuring consistency and minimizing duplication. This requires investment in data integration tools and expertise.
3. Data Quality Monitoring and Cleansing: Proactive Management
Implementing data quality monitoring and cleansing processes is critical. This involves regularly checking for data inconsistencies and duplicates, and implementing automated processes for cleaning and correcting the data. This proactive approach prevents redundancy from accumulating over time.
4. Automating Data Entry and Data Processes: Minimizing Human Error
Automating data entry and other data processes as much as possible can significantly reduce human error and the likelihood of creating redundant data. This may involve implementing robotic process automation (RPA) or other automation technologies.
5. Data De-duplication Techniques: Efficiently Removing Duplicates
Employing data de-duplication techniques is vital for removing existing redundant data. These techniques can involve using specialized software tools or employing manual processes to identify and remove duplicates. Careful consideration must be given to the approach selected to ensure accuracy and completeness.
6. Training and Education: Empowering Data Stewards
Providing training and education to employees on data management best practices is crucial. This empowers individuals to understand the importance of data quality and to avoid creating redundant data. It also enhances their ability to identify and report data quality issues effectively.
Conclusion: The Path to Data-Driven Excellence
Redundant data is a significant obstacle to effective decision-making. It leads to inconsistencies, increased complexity, biased analysis, and ultimately, poor outcomes. By understanding the sources of redundancy and implementing effective data management strategies, organizations can overcome these challenges and leverage data to make better, more informed decisions. The investment in data quality and governance is an investment in the future of the organization, leading to improved decision-making, increased efficiency, and ultimately, a stronger competitive advantage. The path to data-driven excellence involves a commitment to robust data management practices, enabling organizations to harness the true power of their data assets.
Latest Posts
Latest Posts
-
Nurse Susan Is Explaining To Nurse Connor About Isolation Precautions
May 12, 2025
-
Which Expression Is Equivalent To The Following Complex Fraction 1 1 Y 1 1 Y
May 12, 2025
-
Which Of The Following Does Not Describe Treppe
May 12, 2025
-
Andy Is Writing An Article And Wants To Verify
May 12, 2025
-
A Blend Of Command Training Coaching Encouragement
May 12, 2025
Related Post
Thank you for visiting our website which covers about Explain How Redundant Data Often Leads To Poorer Decision Making . We hope the information provided has been useful to you. Feel free to contact us if you have any questions or need further assistance. See you next time and don't miss to bookmark.