Explain How The New Data Supports Your Hypothesis
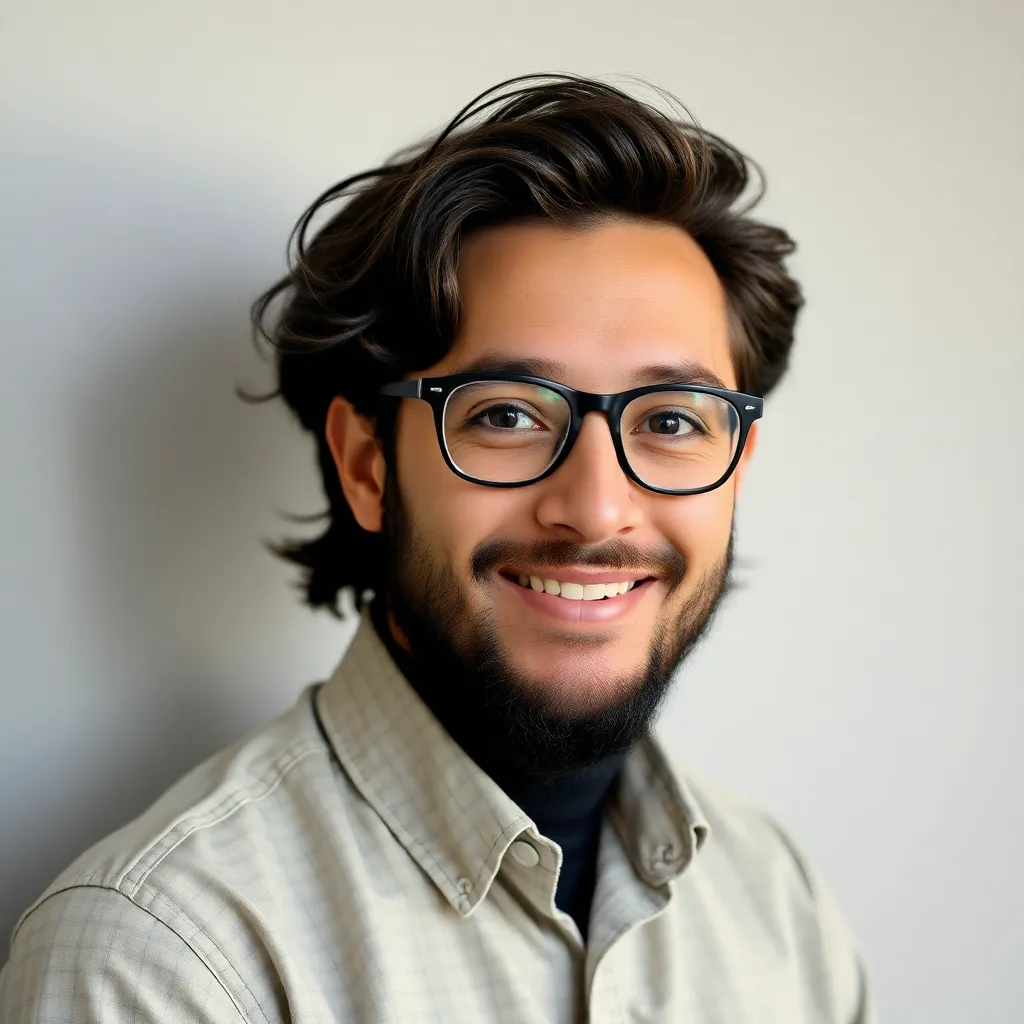
Onlines
May 11, 2025 · 6 min read
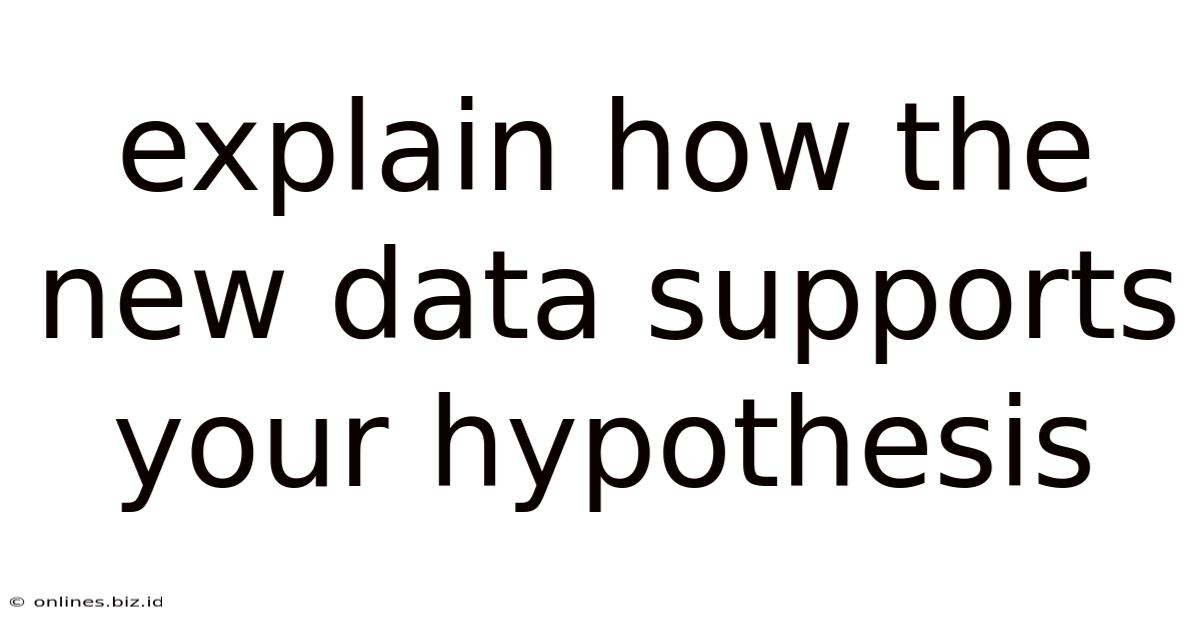
Table of Contents
Explaining How New Data Supports Your Hypothesis: A Comprehensive Guide
Successfully proving a hypothesis requires more than just a hunch; it necessitates rigorous research, data collection, and a compelling analysis demonstrating a clear link between your predictions and observed results. This article provides a comprehensive guide on how to effectively explain how new data supports your hypothesis, focusing on the key elements of scientific rigor, effective communication, and persuasive argumentation.
Understanding the Foundation: Your Hypothesis and Research Design
Before diving into the analysis of new data, it's crucial to revisit the foundational elements of your research: your hypothesis and research design.
1. Clearly Stated Hypothesis:
Your hypothesis should be a testable statement that predicts a relationship between variables. It should be clearly articulated, leaving no room for ambiguity. For example, instead of stating "Exercise is good for you," a better hypothesis would be: "Individuals who engage in regular moderate-intensity aerobic exercise for at least 30 minutes, three times per week, will show a statistically significant reduction in body mass index (BMI) after three months compared to a control group." Note the specificity: the type of exercise, duration, frequency, and measurable outcome are all clearly defined.
2. Robust Research Design:
The research design is the blueprint of your study. A strong design minimizes bias and maximizes the validity and reliability of your results. Key aspects include:
- Sample Size: Sufficiently large sample size ensures statistical power to detect meaningful effects.
- Randomization: Random assignment of participants to different groups (e.g., control and experimental) minimizes selection bias.
- Control Group: A control group provides a baseline for comparison, allowing you to isolate the effects of your independent variable.
- Blinding: When feasible, blinding participants and researchers to group assignments reduces bias in data collection and interpretation.
- Data Collection Methods: Reliable and valid methods are crucial. This could involve standardized questionnaires, objective measurements (e.g., blood pressure, weight), or observations.
Analyzing and Interpreting the New Data
Once you have collected your data, the next step is rigorous analysis and interpretation. This involves several key stages:
1. Data Cleaning and Preparation:
Raw data is rarely perfect. This stage involves identifying and addressing issues such as missing values, outliers, and inconsistencies. Techniques like imputation (replacing missing values) or outlier removal may be necessary, but always justify these decisions and acknowledge potential limitations they may introduce.
2. Descriptive Statistics:
Descriptive statistics summarize your data. This includes measures of central tendency (mean, median, mode), variability (standard deviation, range), and frequency distributions. These provide an initial overview of your data and highlight potential patterns.
3. Inferential Statistics:
Inferential statistics allow you to draw conclusions about a population based on your sample data. The choice of statistical test depends on your research question, data type, and research design. Common tests include t-tests, ANOVA, chi-square tests, and regression analysis. Your choice should be justified based on the statistical assumptions of the test.
4. Visualization:
Visual representations of your data, such as graphs and charts (histograms, scatter plots, box plots), are crucial for effective communication. Well-designed visuals can reveal patterns and relationships in your data that might be missed in numerical summaries alone.
5. Connecting the Data to Your Hypothesis:
The most crucial part is demonstrating the clear connection between your findings and your original hypothesis. Do your results support or refute your hypothesis? If your results are statistically significant, this strengthens your claim. However, even non-significant results can be valuable; they might suggest limitations in your study design or the need to refine your hypothesis.
Example: Let's revisit the exercise and BMI hypothesis. After three months, you analyze the data and find that the experimental group (regular exercise) showed a statistically significant decrease in BMI compared to the control group (no exercise). This statistically significant finding directly supports your initial hypothesis.
Effectively Communicating Your Findings
Clearly and persuasively communicating your findings is as crucial as the research itself.
1. Structure Your Report:
A well-structured report enhances clarity and understanding. This usually involves:
- Introduction: Introduce the background, your hypothesis, and your research design.
- Methods: Detail your data collection and analysis methods.
- Results: Present your findings using descriptive and inferential statistics, accompanied by appropriate visualizations.
- Discussion: Interpret your results, explain how they support or refute your hypothesis, and discuss the implications of your findings. Acknowledge any limitations of your study.
- Conclusion: Summarize your main findings and their significance.
2. Use Clear and Concise Language:
Avoid jargon and technical terms unless your audience is familiar with them. Use simple, direct language to convey your message effectively.
3. Visual Aids:
Graphs, charts, and tables make complex data easier to understand. Choose appropriate visual representations for your data and ensure they are clearly labeled and easy to interpret.
4. Emphasize Statistical Significance:
When presenting statistically significant results, clearly state the p-value (the probability of observing your results if there were no real effect). A p-value less than 0.05 is generally considered statistically significant.
5. Acknowledge Limitations:
No study is perfect. Acknowledge any limitations of your study, such as sample size limitations, potential biases, or methodological constraints. This demonstrates intellectual honesty and strengthens your credibility.
Addressing Potential Challenges and Counterarguments
Anticipating potential challenges and addressing counterarguments strengthens your argument.
1. Conflicting Evidence:
If your data doesn't fully support your hypothesis, discuss potential reasons. Is there conflicting evidence from other studies? Are there alternative explanations for your findings? Acknowledging limitations and exploring alternative explanations shows a nuanced understanding of your research.
2. Methodological Limitations:
Be transparent about any limitations in your methodology. Did you have a small sample size? Were there potential biases in your data collection? Addressing these head-on strengthens your credibility.
3. Statistical Significance vs. Practical Significance:
While statistical significance indicates a reliable effect, it doesn't always translate to practical significance. Consider the magnitude of the effect and its real-world implications. A small statistically significant effect might not be practically meaningful.
4. Alternative Hypotheses:
Explore alternative hypotheses that could explain your findings. This shows a comprehensive understanding of the research area and strengthens your argument by addressing potential counterarguments.
Strengthening Your Argument: Further Considerations
Beyond the core elements, several additional steps can strengthen your argument and bolster your hypothesis:
- Replication: Independent replication of your study by other researchers is a powerful validation of your findings.
- Meta-analysis: If multiple studies have examined similar questions, a meta-analysis can combine the results to provide a more comprehensive overview.
- Longitudinal Studies: Longitudinal studies, which follow participants over an extended period, can provide more robust evidence than cross-sectional studies.
- Triangulation: Using multiple methods (e.g., surveys, observations, physiological measurements) to gather data increases the validity and reliability of your findings.
By diligently following these steps, you can effectively explain how new data supports your hypothesis, building a strong and persuasive case for your research conclusions. Remember that rigorous research, careful analysis, and clear communication are the cornerstones of successful scientific inquiry. Your goal isn't just to present data, but to tell a compelling story that effectively conveys the significance of your findings.
Latest Posts
Related Post
Thank you for visiting our website which covers about Explain How The New Data Supports Your Hypothesis . We hope the information provided has been useful to you. Feel free to contact us if you have any questions or need further assistance. See you next time and don't miss to bookmark.