Factorial Designs Are Often Employed Because
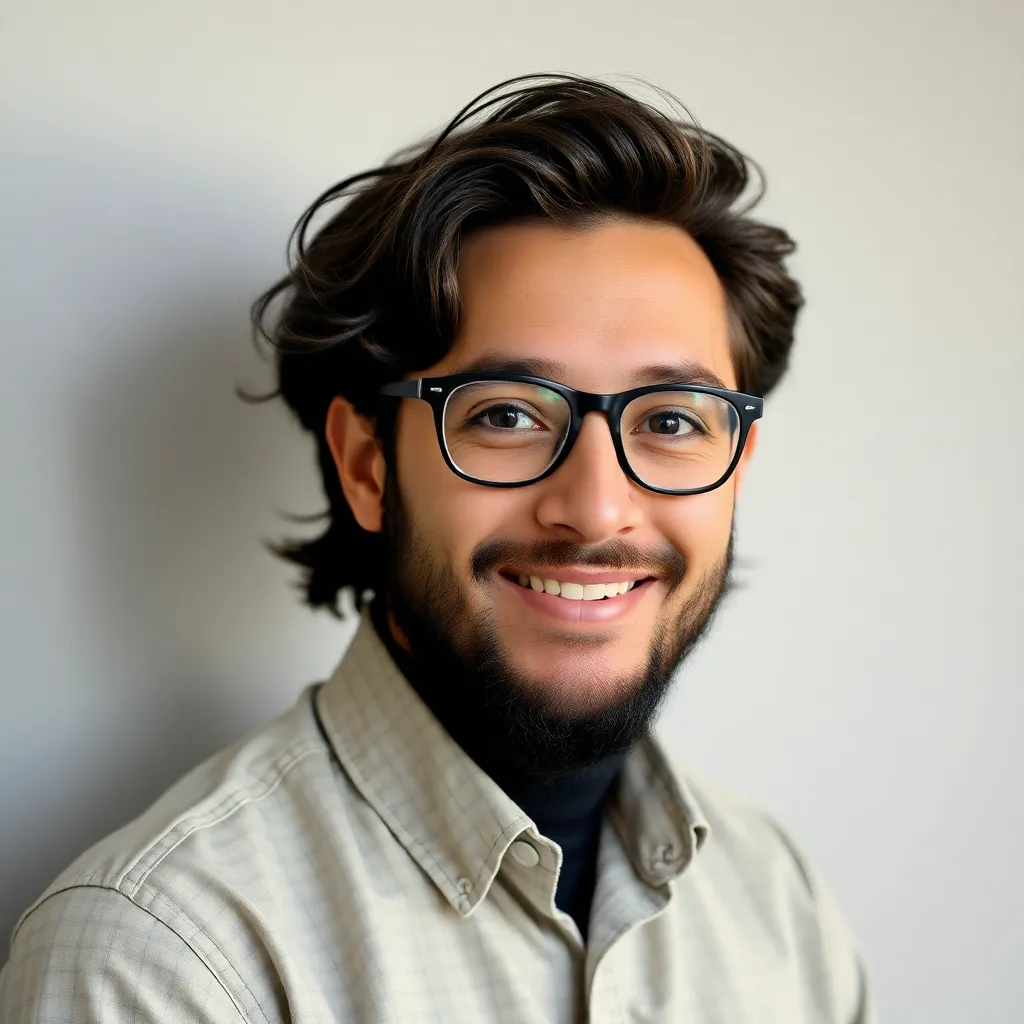
Onlines
May 08, 2025 · 5 min read
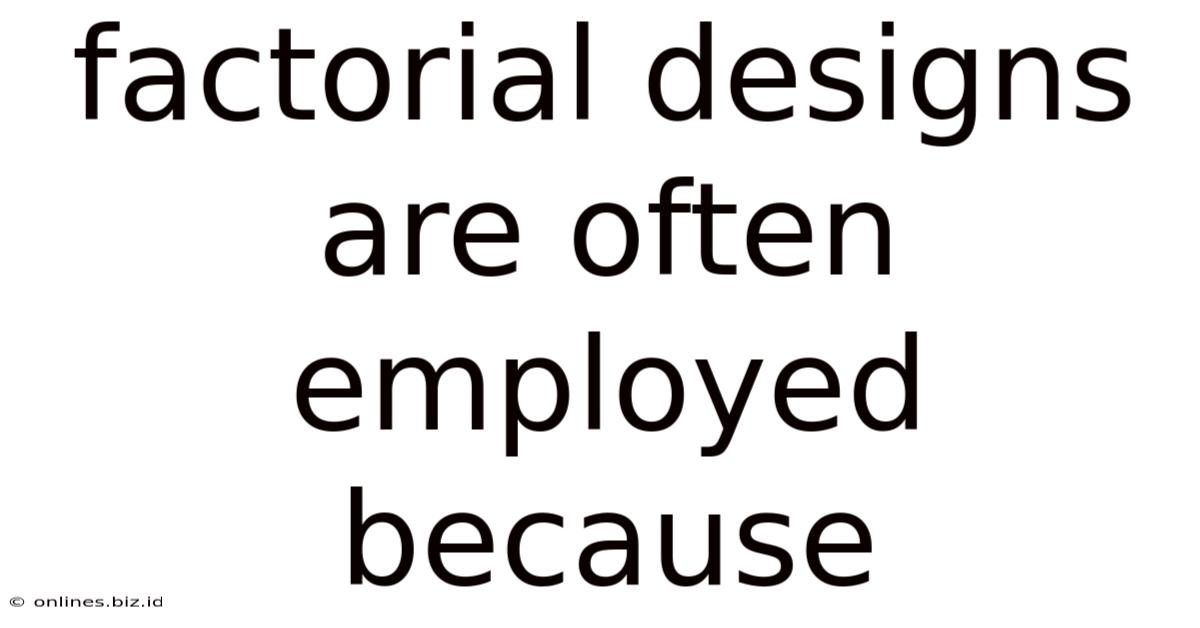
Table of Contents
Factorial Designs: Why They Reign Supreme in Experimental Design
Factorial designs are a cornerstone of experimental design, prized for their efficiency and the wealth of information they yield. But why are they so often employed? The answer lies in their ability to simultaneously investigate the effects of multiple factors (independent variables) and, crucially, the interactions between those factors. This article delves deep into the reasons behind the widespread adoption of factorial designs, exploring their benefits and applications across various fields.
The Power of Simultaneous Investigation
One primary reason for the popularity of factorial designs is their efficiency. Unlike one-factor-at-a-time (OFAT) approaches, which examine each independent variable in isolation, factorial designs evaluate all factors and their combinations in a single experiment. This drastically reduces the time, resources, and cost associated with experimentation. Imagine testing three different fertilizers (Factor A) and three different watering techniques (Factor B) on crop yield. An OFAT approach would require nine separate experiments. A factorial design, however, can achieve the same results in a more streamlined manner, potentially using fewer experimental units overall.
Reduced Experimental Error and Improved Precision
By simultaneously testing all factors, factorial designs inherently minimize experimental error. Variations due to uncontrolled factors (environmental changes, instrument drift, etc.) are more evenly distributed across different treatment combinations, leading to more precise estimations of the effects. This improved precision is crucial for drawing reliable conclusions and making informed decisions based on the experimental findings.
Unveiling the Secrets of Interactions
Perhaps the most significant advantage of factorial designs lies in their ability to detect interactions between factors. An interaction occurs when the effect of one factor depends on the level of another factor. Consider our fertilizer and watering example: the optimal fertilizer might differ depending on the watering technique. A factorial design allows researchers to identify this interaction, which would be completely missed by an OFAT approach. This understanding of interactions is critical in many applications. For example, in drug development, understanding the interaction between different drugs or between a drug and an individual’s genetic makeup can be vital for determining efficacy and safety.
Understanding Complex Systems Through Interactions
Many real-world phenomena are governed by complex relationships between multiple factors. Factorial designs excel at disentangling these relationships. They don't just provide information on the main effects of each factor but also reveal how these factors combine to influence the outcome. This is particularly valuable in fields like:
- Engineering: Optimizing the performance of a product by understanding the interaction between different design parameters.
- Manufacturing: Improving process efficiency by examining the interaction between different process variables.
- Agriculture: Enhancing crop yield by studying the interaction between fertilizer type, watering, and soil conditions.
- Medicine: Developing effective treatments by investigating the interaction between drugs and patient characteristics.
- Marketing: Optimizing marketing campaigns by exploring the interaction between advertising channels and target audiences.
Types of Factorial Designs: A Spectrum of Options
Factorial designs are not one-size-fits-all. They come in various forms, each offering advantages tailored to specific needs:
Completely Randomized Designs (CRD):
The simplest type, CRD involves randomly assigning experimental units to different treatment combinations. This approach is suitable when experimental units are homogeneous and there are no confounding factors.
Randomized Complete Block Designs (RCBD):
RCBD is employed when experimental units are not homogeneous. Experimental units are grouped into blocks (e.g., batches of materials, different geographical locations) where units within a block are more similar. Treatment combinations are then randomly assigned within each block. This design accounts for the variability between blocks, improving the precision of the experiment.
Split-Plot Designs:
These designs are used when factors are applied at different levels or when it's impractical to randomly assign treatments to all units. For instance, one factor (e.g., fertilizer type) might be applied to large plots of land, while another factor (e.g., watering technique) is applied to smaller subplots within those larger plots.
Latin Square Designs:
Latin square designs are particularly useful when the experimental units are arranged in a grid (e.g., rows and columns). Each treatment appears only once in each row and each column, thereby minimizing potential bias from row and column effects.
Analyzing Factorial Designs: Unveiling the Data's Story
Analyzing the data from a factorial design involves statistical methods like Analysis of Variance (ANOVA). ANOVA helps determine the significance of the main effects of each factor and the interactions between factors. The results are usually presented in tables and graphs, visualizing the effects and interactions.
Interpreting ANOVA Results:
Understanding the p-values and F-statistics from ANOVA is crucial for interpreting the results. A statistically significant p-value (typically less than 0.05) indicates that a factor or interaction has a statistically significant effect on the response variable.
Beyond the Basics: Fractional Factorial Designs
When dealing with a large number of factors, conducting a full factorial design can become impractical. Fractional factorial designs offer a solution. They involve running only a fraction of the possible treatment combinations while still being able to estimate the main effects and some interactions. This approach is particularly useful in exploratory studies where the goal is to identify the most important factors before conducting a more comprehensive experiment.
Taguchi Methods: Robust Design
Taguchi methods are a specialized form of fractional factorial design that emphasizes robustness. The goal is to design products and processes that are insensitive to variations in environmental conditions or manufacturing tolerances.
Choosing the Right Factorial Design: A Strategic Approach
Selecting the appropriate factorial design depends on several factors, including:
- The number of factors: More factors lead to more complex designs.
- The number of levels for each factor: Each factor can have multiple levels (e.g., high, medium, low).
- The resources available: Time, cost, and the number of experimental units constrain the choice of design.
- The expected interactions: If interactions are suspected, a design capable of detecting interactions should be chosen.
- The desired level of precision: More precise designs require more experimental units.
Conclusion: The Enduring Relevance of Factorial Designs
Factorial designs remain a dominant force in experimental design due to their efficiency, power to detect interactions, and adaptability to various experimental situations. Their capacity to provide a comprehensive understanding of complex relationships between multiple factors makes them indispensable across numerous disciplines. Whether optimizing a manufacturing process, designing a new drug, or improving crop yield, the principles and techniques of factorial design offer a powerful and robust methodology for generating valuable insights and making informed decisions. As our understanding of complex systems deepens, the role of factorial designs in unraveling their intricacies will only continue to grow.
Latest Posts
Latest Posts
-
In Fayetteville The Library Is 3 Miles
May 08, 2025
-
Refer To The Diagram Flow 3 Represents
May 08, 2025
-
A Double Blind Study Of A Vaccine Is One In Which
May 08, 2025
-
The Cost Of Successfully Defending A Patent Suit Should Be
May 08, 2025
-
The Human Body Should Be Functioning At Its Optimal Level
May 08, 2025
Related Post
Thank you for visiting our website which covers about Factorial Designs Are Often Employed Because . We hope the information provided has been useful to you. Feel free to contact us if you have any questions or need further assistance. See you next time and don't miss to bookmark.