Graphing And Data Analysis Worksheet Answer Key
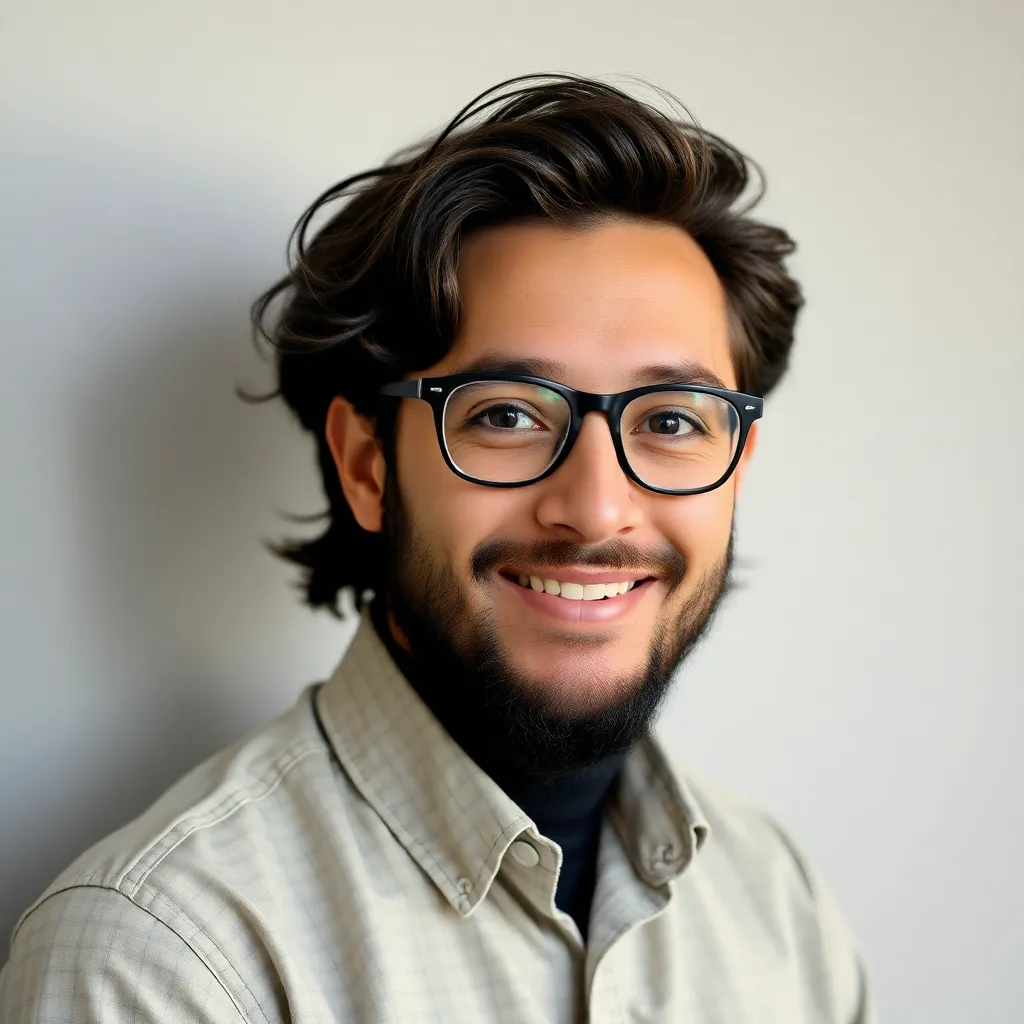
Onlines
Apr 10, 2025 · 7 min read

Table of Contents
Graphing and Data Analysis Worksheet Answer Key: A Comprehensive Guide
Data analysis is a cornerstone of modern decision-making across numerous fields, from scientific research to business strategy. Understanding how to effectively represent and interpret data is paramount. This comprehensive guide serves as a virtual answer key and walkthrough for common graphing and data analysis worksheets, encompassing various chart types, statistical measures, and analytical techniques. While we won't provide specific answers to your worksheet (as that would defeat the learning process!), we'll equip you with the knowledge to solve any problem you encounter.
Understanding Different Chart Types
Before diving into analysis, selecting the appropriate chart type is crucial for clear data visualization. Mismatched charts can obscure insights and mislead interpretations. Let's explore some common chart types and their best applications:
1. Bar Charts: Comparing Categories
Bar charts are ideal for comparing discrete categories. They use rectangular bars with lengths proportional to the values they represent. Consider these aspects:
- Vertical vs. Horizontal: Vertical bar charts are generally preferred for easier readability, especially with numerous categories. Horizontal bars might be better when category labels are long.
- Clustered Bar Charts: These are perfect for comparing multiple data series within the same categories. For example, you might show sales figures for different products over several quarters.
- Stacked Bar Charts: Useful when showing the composition of a whole. Each segment of a bar represents a portion of the total value.
Example: A bar chart could effectively illustrate the number of students enrolled in different subjects (Math, Science, English, etc.).
2. Line Charts: Showing Trends Over Time
Line charts excel at visualizing trends and changes over continuous data, often time-based. They connect data points with lines, revealing patterns and fluctuations.
- Multiple Lines: Useful for comparing trends across different categories or variables.
- Interpolation: Lines connecting points imply a continuous relationship. Don't overinterpret interpolated values between actual data points.
- Extrapolation: Extending the line beyond the data range to predict future trends should be done cautiously, only with a clear understanding of the underlying patterns and limitations.
Example: A line chart is best suited to showing the temperature fluctuations over a 24-hour period or the growth of a company's revenue over several years.
3. Pie Charts: Representing Proportions
Pie charts effectively display the proportion of different categories relative to a whole. Each slice represents a percentage of the total.
- Labeling: Clearly label each slice with its category and percentage for easy understanding.
- Limited Categories: Pie charts become less effective with many categories, as it becomes difficult to differentiate small slices.
- Avoid 3D Pie Charts: These can distort the perception of proportions.
Example: A pie chart could show the percentage of respondents in a survey who prefer different colors.
4. Scatter Plots: Examining Relationships
Scatter plots reveal the relationship between two variables. Each point represents a data pair, and the overall pattern reveals correlation (or lack thereof).
- Correlation: Points clustered along a line suggest a strong positive or negative correlation. Scattered points indicate a weak or no correlation.
- Outliers: Identify data points that deviate significantly from the overall pattern. These might warrant further investigation.
- Trend Lines: Adding a trend line (often a regression line) can help visualize the relationship more clearly.
Example: A scatter plot can illustrate the relationship between hours studied and exam scores.
5. Histograms: Showing Data Distribution
Histograms display the frequency distribution of a single continuous variable. Data is grouped into bins (intervals), and the height of each bar represents the frequency of data within that bin.
- Bin Size: The choice of bin size can significantly affect the appearance of the histogram. Experiment with different bin sizes to find the most informative representation.
- Skewness: Observe the symmetry (or lack thereof) of the histogram. A skewed distribution suggests that data is clustered more towards one end.
- Mode: The highest bar represents the mode (most frequent value) in the data.
Example: A histogram could show the distribution of heights in a group of people.
Key Statistical Measures for Data Analysis
Analyzing data often involves calculating several key statistical measures to summarize and understand its characteristics.
1. Mean, Median, and Mode
- Mean (Average): The sum of all values divided by the number of values. Sensitive to outliers.
- Median: The middle value when the data is ordered. Less sensitive to outliers than the mean.
- Mode: The most frequent value. Can have multiple modes or no mode at all.
Understanding the differences between these measures helps reveal the central tendency of the data and its potential skewness.
2. Range and Variance
- Range: The difference between the maximum and minimum values. A simple measure of spread but sensitive to outliers.
- Variance: The average of the squared differences from the mean. A more robust measure of spread than the range. The square root of the variance is the standard deviation.
3. Standard Deviation
The standard deviation measures the dispersion of data around the mean. A small standard deviation indicates data clustered closely around the mean, while a large standard deviation indicates more spread-out data.
4. Percentiles and Quartiles
- Percentiles: Values that divide the data into 100 equal parts. The 25th percentile is the first quartile (Q1), the 50th percentile is the median (Q2), and the 75th percentile is the third quartile (Q3).
- Interquartile Range (IQR): The difference between the third and first quartiles (Q3 - Q1). A measure of spread resistant to outliers.
Interpreting Data and Drawing Conclusions
Once you've created your graphs and calculated relevant statistical measures, it's time to interpret the results and draw meaningful conclusions. This involves:
- Identifying Trends: Look for patterns and trends in your graphs. What are the highs and lows? Are there any clear upward or downward trends?
- Comparing Data: Compare different categories or variables to identify similarities and differences.
- Considering Outliers: Investigate any data points that significantly deviate from the overall pattern. Are they errors or legitimate values with unique characteristics?
- Drawing Conclusions: Based on your analysis, what conclusions can you draw about the data? Are there any implications or actions that should be taken?
- Limitations: Always acknowledge the limitations of your analysis. Were there any biases in data collection? Are there factors that were not considered?
Advanced Data Analysis Techniques
For more complex datasets, you might need to utilize more advanced techniques. These include:
- Regression Analysis: Used to model the relationship between a dependent variable and one or more independent variables.
- Correlation Analysis: Measures the strength and direction of the linear relationship between two variables.
- Hypothesis Testing: Used to test whether a hypothesis about the data is supported by the evidence.
- ANOVA (Analysis of Variance): Used to compare the means of two or more groups.
- Chi-Square Test: Used to determine if there is a significant association between two categorical variables.
Tips for Success with Graphing and Data Analysis Worksheets
- Read the instructions carefully: Understand what is being asked before attempting to solve the problems.
- Choose the appropriate chart type: Select the chart that best suits the data and the questions being asked.
- Label your graphs clearly: Include titles, axis labels, and legends to ensure clarity.
- Show your work: Document your calculations and reasoning.
- Check your answers: Review your work to identify any errors.
- Practice regularly: The more you practice, the better you will become at graphing and data analysis.
This comprehensive guide provides a strong foundation for tackling graphing and data analysis worksheets. Remember, the key to success lies in understanding the different chart types, statistical measures, and analytical techniques, and then applying them appropriately to the data at hand. By following these steps and practicing regularly, you can develop the skills necessary to effectively analyze data and draw insightful conclusions. While we haven’t provided specific worksheet answers, this detailed explanation will allow you to confidently approach any problem you might encounter. Good luck!
Latest Posts
Latest Posts
-
Ap Chemistry Unit 1 Progress Check Mcq
Apr 18, 2025
-
Why Did The Orchestra Get An R Rating
Apr 18, 2025
-
The Concept Of Social Complexity Suggests That
Apr 18, 2025
-
Cer Analyzing Data And Tiger Sharks Answer Key
Apr 18, 2025
-
Work Equilibrium And Free Energy Pogil Answer Key
Apr 18, 2025
Related Post
Thank you for visiting our website which covers about Graphing And Data Analysis Worksheet Answer Key . We hope the information provided has been useful to you. Feel free to contact us if you have any questions or need further assistance. See you next time and don't miss to bookmark.