Identify The True And False Statements About Factorial Designs.
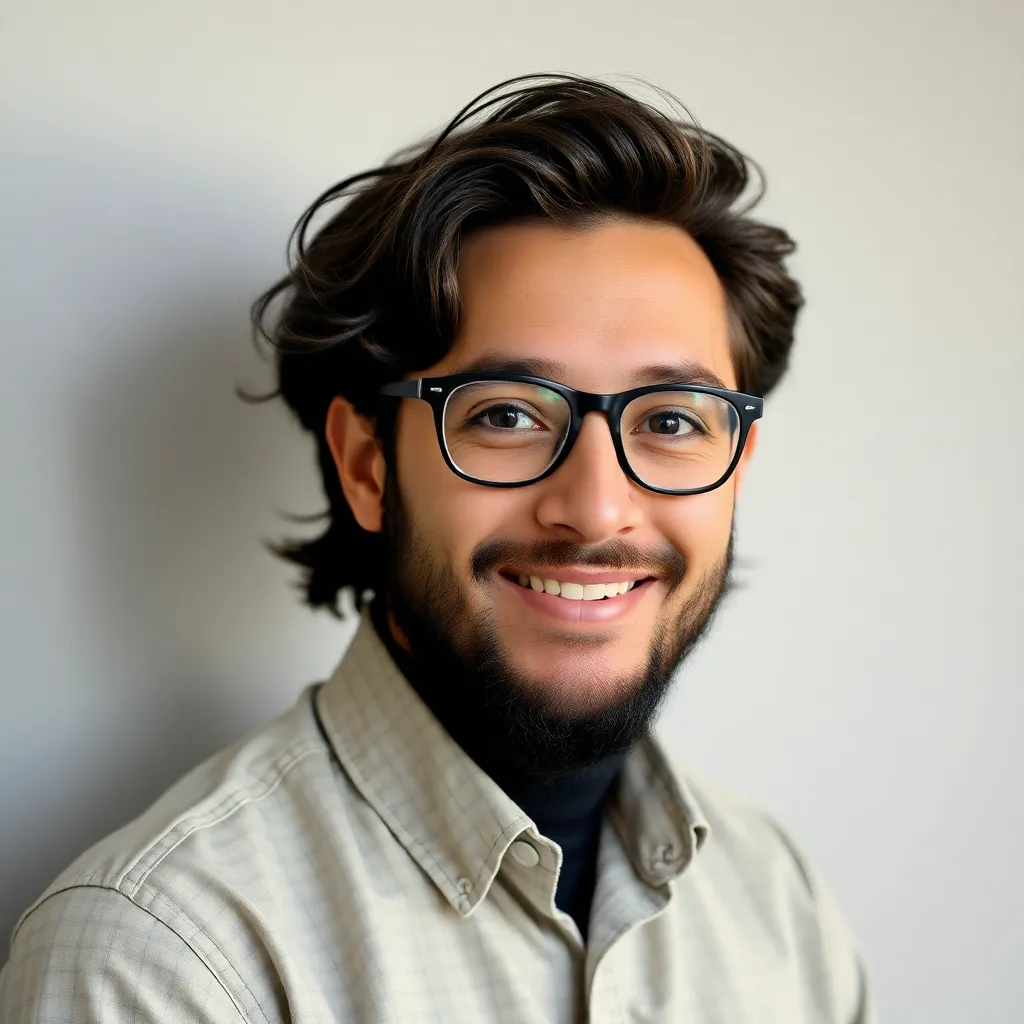
Onlines
May 09, 2025 · 6 min read
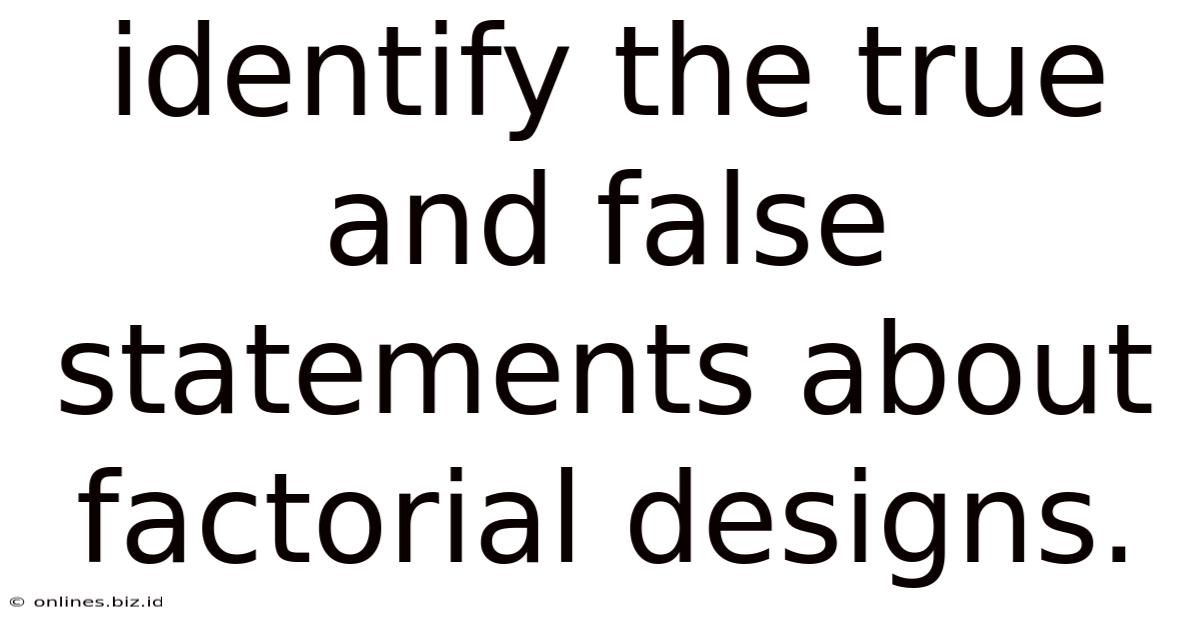
Table of Contents
Identifying True and False Statements About Factorial Designs
Factorial designs are a cornerstone of experimental research, offering a powerful approach to investigating the effects of multiple independent variables (factors) simultaneously. Understanding their nuances is crucial for researchers across various disciplines. This article delves into common misconceptions surrounding factorial designs, clarifying true and false statements to solidify your comprehension.
Understanding Factorial Designs: A Foundation
Before tackling the true/false statements, let's establish a foundational understanding. A factorial design examines the influence of two or more independent variables (factors) on a dependent variable. Each independent variable has two or more levels, creating a combination of conditions. For example, a 2x2 factorial design involves two factors, each with two levels. A 3x2x2 factorial design involves three factors; the first with three levels, and the second and third with two levels each. The beauty of factorial designs lies in their ability to assess not only the main effects of each independent variable but also the interactions between them.
Main effects represent the overall effect of a single independent variable, averaging across the levels of other factors. Interactions occur when the effect of one independent variable differs depending on the level of another. This is where factorial designs truly shine, revealing complexities often missed by studying factors in isolation.
True or False: Debunking Common Myths
Now, let's address some common statements about factorial designs, identifying whether they are true or false and providing detailed explanations.
Statement 1: Factorial designs are only suitable for experiments with a small number of factors. (FALSE)
While simpler designs (e.g., 2x2) are easier to manage, factorial designs can accommodate numerous factors. However, the number of participants and experimental conditions increase exponentially with each added factor and level. Larger designs demand careful consideration of resources, statistical power, and the potential for participant fatigue. Techniques like fractional factorial designs can mitigate this complexity by strategically selecting a subset of all possible condition combinations. These are still considered factorial designs, just more efficient versions.
Statement 2: Factorial designs always require a between-subjects design. (FALSE)
While between-subjects designs (different participants in each condition) are common with factorial designs, they are not mandatory. Within-subjects designs (same participants experiencing all conditions) can also be employed. Within-subjects designs offer increased statistical power by controlling for individual differences, but they are susceptible to order effects (the order of conditions influencing the results). Counterbalancing techniques help mitigate this concern. Mixed designs, combining between- and within-subjects elements, are also possible. The choice depends on the research question, the nature of the variables, and potential confounding factors.
Statement 3: Interaction effects are always more important than main effects. (FALSE)
The relative importance of main effects and interactions depends entirely on the research question and the results. In some studies, main effects may be the primary focus, while in others, the interactions are the most interesting and theoretically relevant finding. For example, a study examining the effects of caffeine and sleep deprivation on cognitive performance might find a strong interaction effect: the effect of caffeine differs significantly depending on the level of sleep deprivation. However, the main effect of sleep deprivation might be equally or even more important in terms of public health implications. The interpretation always depends on the specific context and the magnitude of the observed effects.
Statement 4: Interpreting interactions requires examining simple main effects. (TRUE)
When a significant interaction is found, understanding its nature requires examining simple main effects. These effects isolate the impact of one independent variable at a specific level of another. For instance, in the caffeine/sleep deprivation example, we would analyze the effect of caffeine at high sleep deprivation, and separately at low sleep deprivation levels. This allows for a precise understanding of how the variables interact to affect the dependent variable. Simple effects analysis provides a nuanced interpretation beyond the overall interaction effect.
Statement 5: Factorial designs are only appropriate for experimental research. (FALSE)
Although frequently used in experiments, factorial designs are applicable in observational studies as well. While researchers can't manipulate independent variables directly in observational studies, they can examine the relationships between pre-existing groups or naturally occurring levels of factors. For instance, researchers might study the relationship between gender (a factor with two levels) and educational attainment (a factor with multiple levels), analyzing their effects on income. The analysis remains similar to experimental factorial designs, but the conclusions about causality are more limited.
Statement 6: Larger factorial designs always yield more significant results. (FALSE)
The size of a factorial design does not guarantee statistically significant results. Statistical significance depends on factors such as effect size, sample size, and the chosen alpha level. A larger design might require a larger sample size to maintain the desired statistical power. While increasing the number of factors and levels can increase the potential for discovering effects, it also increases the risk of Type I error (false positives). Careful consideration of power analysis is essential to ensure the design is adequately powered to detect meaningful effects.
Statement 7: All possible interactions must be examined in a factorial ANOVA. (TRUE)
Factorial ANOVA (Analysis of Variance) examines all possible main effects and interactions between the factors included in the design. The ANOVA model systematically partitions the variance in the dependent variable, assessing the contribution of each factor and their combinations. Failure to consider all interactions could lead to a misleading interpretation of the results, as significant interactions could mask or modify the effects of main factors.
Statement 8: Factorial designs are difficult to analyze statistically. (FALSE)
While the analysis can be complex for very large designs, standard statistical software packages readily handle the analysis of factorial designs using ANOVA or other appropriate techniques. The interpretation of the results might require some statistical expertise, especially when dealing with complex interactions, but the computational burden is easily handled by widely available tools.
Statement 9: A significant interaction implies that the main effects are unimportant. (FALSE)
Significant interactions and significant main effects can coexist. A significant interaction indicates that the effect of one independent variable depends on the level of another, but this doesn’t negate the importance of the main effects. The main effects still represent the average effects of each independent variable across all levels of the other factors, providing valuable information about the overall influence of each factor. Understanding both main effects and interactions offers a comprehensive understanding of the phenomena under investigation.
Statement 10: Confounding variables are easily controlled in factorial designs. (FALSE)
While factorial designs allow for the simultaneous examination of multiple independent variables, they don't automatically control for confounding variables. Confounding variables are extraneous factors that correlate with both the independent and dependent variables, potentially distorting the results. Careful experimental design, including random assignment of participants and appropriate controls, are essential to minimize the influence of confounding variables in any study, including those using factorial designs.
Conclusion: Mastering Factorial Designs for Robust Research
Factorial designs are incredibly versatile tools for exploring complex relationships between variables. This article has clarified several common misconceptions, emphasizing that while some aspects can be computationally or conceptually challenging, the advantages of examining multiple factors simultaneously, including their interactions, often outweigh the increased complexity. By understanding the true and false statements outlined here, researchers can more effectively design, implement, and interpret factorial designs, leading to robust and meaningful research conclusions. Remember, sound experimental design, proper statistical analysis, and a thorough understanding of the underlying concepts are key to maximizing the benefits of factorial designs.
Latest Posts
Latest Posts
-
A Network Administrator Enters The Service Password Encryption
May 09, 2025
-
The Five Step Marketing Research Approach Begins With
May 09, 2025
-
A Websites Analytics Report Shows That The Average
May 09, 2025
-
Provide A Systematic Name Of The Following Compound Below
May 09, 2025
-
Jko Dha Employee Safety Course Answers
May 09, 2025
Related Post
Thank you for visiting our website which covers about Identify The True And False Statements About Factorial Designs. . We hope the information provided has been useful to you. Feel free to contact us if you have any questions or need further assistance. See you next time and don't miss to bookmark.