Identify The True And False Statements About Multiple Regression.
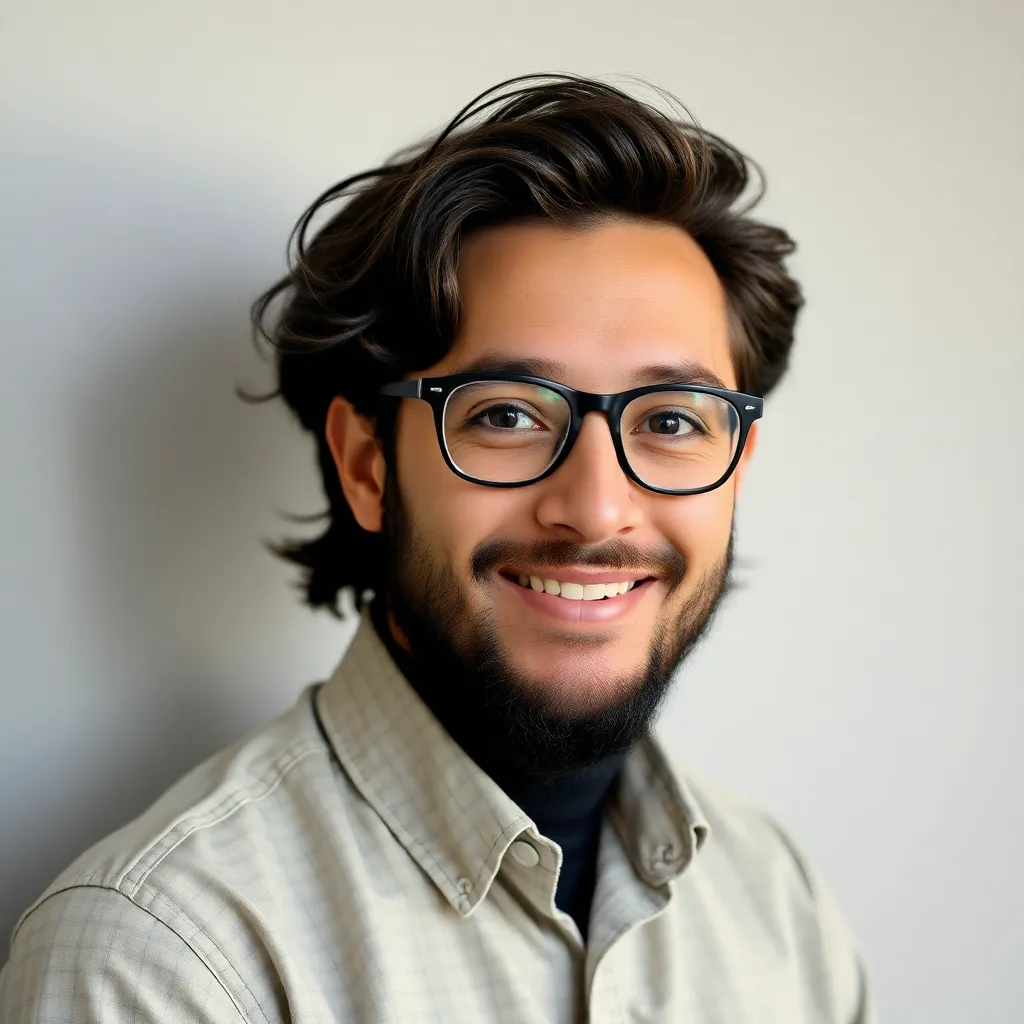
Onlines
Apr 19, 2025 · 7 min read

Table of Contents
Identifying True and False Statements About Multiple Regression: A Comprehensive Guide
Multiple regression analysis is a powerful statistical technique used to model the relationship between a single dependent variable and two or more independent variables. Understanding its nuances is crucial for accurate interpretation and effective application. This comprehensive guide will delve into common statements about multiple regression, identifying those that are true and those that are false, explaining the reasoning behind each. We will explore various aspects, from assumptions to interpretations, aiming to provide a clear and insightful understanding.
Understanding the Fundamentals of Multiple Regression
Before diving into true and false statements, let's solidify our understanding of the basics. Multiple regression aims to find the best-fitting linear equation that predicts the dependent variable (Y) based on the independent variables (X1, X2, X3… Xn). This equation takes the form:
Y = β0 + β1X1 + β2X2 + β3X3 + ... + βnXn + ε
Where:
- Y is the dependent variable.
- X1, X2, X3... Xn are the independent variables.
- β0 is the y-intercept (the value of Y when all X's are 0).
- β1, β2, β3... βn are the regression coefficients representing the change in Y for a one-unit change in the corresponding X, holding all other X's constant.
- ε is the error term, representing the unexplained variation in Y.
True and False Statements About Multiple Regression
Now, let's examine some common statements about multiple regression, categorizing them as true or false and providing detailed explanations.
Statement 1: Multiple regression assumes a linear relationship between the dependent variable and each independent variable.
TRUE. This is a fundamental assumption. The model assumes that the change in the dependent variable is directly proportional to the change in each independent variable. Non-linear relationships require transformations of the variables or the use of non-linear regression techniques. Violations of this assumption can lead to biased and inefficient estimates.
Statement 2: Multicollinearity, where independent variables are highly correlated, significantly impacts the reliability of regression coefficients.
TRUE. High multicollinearity makes it difficult to isolate the individual effects of each independent variable on the dependent variable. The regression coefficients become unstable and their standard errors inflate, making it hard to determine statistical significance. Techniques like Variance Inflation Factor (VIF) are used to detect and address multicollinearity.
Statement 3: Multiple regression requires the independent variables to be normally distributed.
FALSE. While normality of the residuals (the differences between observed and predicted values of Y) is a crucial assumption for inference (e.g., hypothesis testing), the independent variables themselves do not need to be normally distributed. However, significant departures from normality in the independent variables might still affect the efficiency of the estimates.
Statement 4: A high R-squared value always indicates a good model.
FALSE. R-squared measures the proportion of variance in the dependent variable explained by the independent variables. While a high R-squared is generally desirable, it doesn't guarantee a good model. Overfitting, where the model fits the sample data too well but generalizes poorly to new data, can lead to a high R-squared despite poor predictive power. Adjusted R-squared, which penalizes the inclusion of irrelevant variables, is a better indicator of model fit.
Statement 5: The significance of individual regression coefficients can be assessed using t-tests.
TRUE. t-tests are used to test the null hypothesis that a specific regression coefficient is equal to zero. A significant t-statistic (typically with a p-value below a chosen significance level, like 0.05) indicates that the corresponding independent variable has a statistically significant effect on the dependent variable, holding other variables constant.
Statement 6: Outliers can severely influence the results of multiple regression analysis.
TRUE. Outliers are data points that are significantly different from other data points. They can exert undue influence on the regression line, distorting the estimates of the regression coefficients and affecting the overall model fit. Identifying and addressing outliers (through transformation, removal, or robust regression techniques) is crucial for obtaining reliable results.
Statement 7: Multiple regression can only be used with continuous dependent and independent variables.
FALSE. While commonly used with continuous variables, multiple regression can also be applied with categorical variables after appropriate coding (e.g., dummy coding for binary variables). However, the interpretation of the coefficients might require careful consideration depending on the coding scheme. Generalized linear models (GLMs) extend regression to handle various types of dependent variables, including binary and count data.
Statement 8: Heteroscedasticity, where the variance of the residuals is not constant, violates a key assumption of multiple regression.
TRUE. Homoscedasticity, or constant variance of residuals, is an important assumption. Heteroscedasticity leads to inefficient and potentially biased standard errors of the regression coefficients, affecting the reliability of hypothesis tests and confidence intervals. Transformations of the variables or weighted least squares regression can mitigate the effects of heteroscedasticity.
Statement 9: Multiple regression automatically selects the most important independent variables.
FALSE. Multiple regression does not automatically select variables. Feature selection techniques, such as stepwise regression, forward selection, backward elimination, or best subset selection, are often employed to identify the most relevant subset of independent variables. These methods have their own limitations and require careful consideration. The best approach is often guided by theoretical understanding and subject matter expertise.
Statement 10: The interpretation of regression coefficients depends on the scaling of the independent variables.
TRUE. The magnitude of the regression coefficients depends on the units of measurement of the independent variables. Standardizing the independent variables (e.g., by converting them to z-scores) allows for a direct comparison of the relative importance of different independent variables based on the standardized regression coefficients (often called beta coefficients).
Statement 11: Multiple regression can handle non-linear relationships between independent and dependent variables directly.
FALSE. Standard multiple regression assumes linear relationships. To model non-linear relationships, you need to incorporate non-linear terms (e.g., squared terms, interaction terms) into the model or use non-linear regression techniques. Polynomial regression, spline regression, and other non-linear methods are appropriate in such cases.
Statement 12: A perfectly fitting model (R-squared = 1) implies a causal relationship between the independent and dependent variables.
FALSE. A high R-squared only indicates a strong statistical association, not necessarily a causal relationship. Correlation does not equal causation. Other factors could be influencing the relationship, or the relationship could be spurious. Establishing causality requires careful consideration of other potential confounding variables and well-designed experiments.
Statement 13: The residuals from a multiple regression model should be independent of each other.
TRUE. This is an important assumption, often referred to as the independence of errors. If the residuals are correlated (e.g., autocorrelation in time series data), the standard errors of the regression coefficients will be underestimated, leading to inflated Type I error rates. Techniques like Durbin-Watson test can be used to assess autocorrelation.
Statement 14: Multiple regression can be used to predict future values of the dependent variable.
TRUE. One of the primary uses of multiple regression is prediction. Once a model is built and validated, it can be used to predict the value of the dependent variable for new observations based on their independent variable values. However, the accuracy of predictions depends heavily on the quality of the model and the similarity between the new data and the data used to build the model.
Statement 15: Understanding the limitations and assumptions of multiple regression is crucial for appropriate interpretation and application.
TRUE. This statement is paramount. Misinterpreting results or ignoring assumptions can lead to inaccurate conclusions and flawed decision-making. A thorough understanding of the assumptions, limitations, and appropriate diagnostic tests is essential for responsible use of multiple regression.
This comprehensive exploration of true and false statements regarding multiple regression should significantly enhance your understanding of this vital statistical tool. Remember that proper application requires careful consideration of assumptions, diagnostic checks, and the limitations inherent in any statistical model. By understanding these nuances, you can utilize multiple regression effectively and draw reliable inferences from your data.
Latest Posts
Latest Posts
-
2 3 Exponential Functions Practice Answer Key
Apr 19, 2025
-
Making Qualitative Estimates Of Ph Change
Apr 19, 2025
-
Which Scenario Describes The Use Of A Public Api
Apr 19, 2025
-
Final Draft 4 Answer Key Pdf
Apr 19, 2025
-
Mr Bickford Did Not Quite Qualify
Apr 19, 2025
Related Post
Thank you for visiting our website which covers about Identify The True And False Statements About Multiple Regression. . We hope the information provided has been useful to you. Feel free to contact us if you have any questions or need further assistance. See you next time and don't miss to bookmark.