Is The Data Set Approximately Periodic
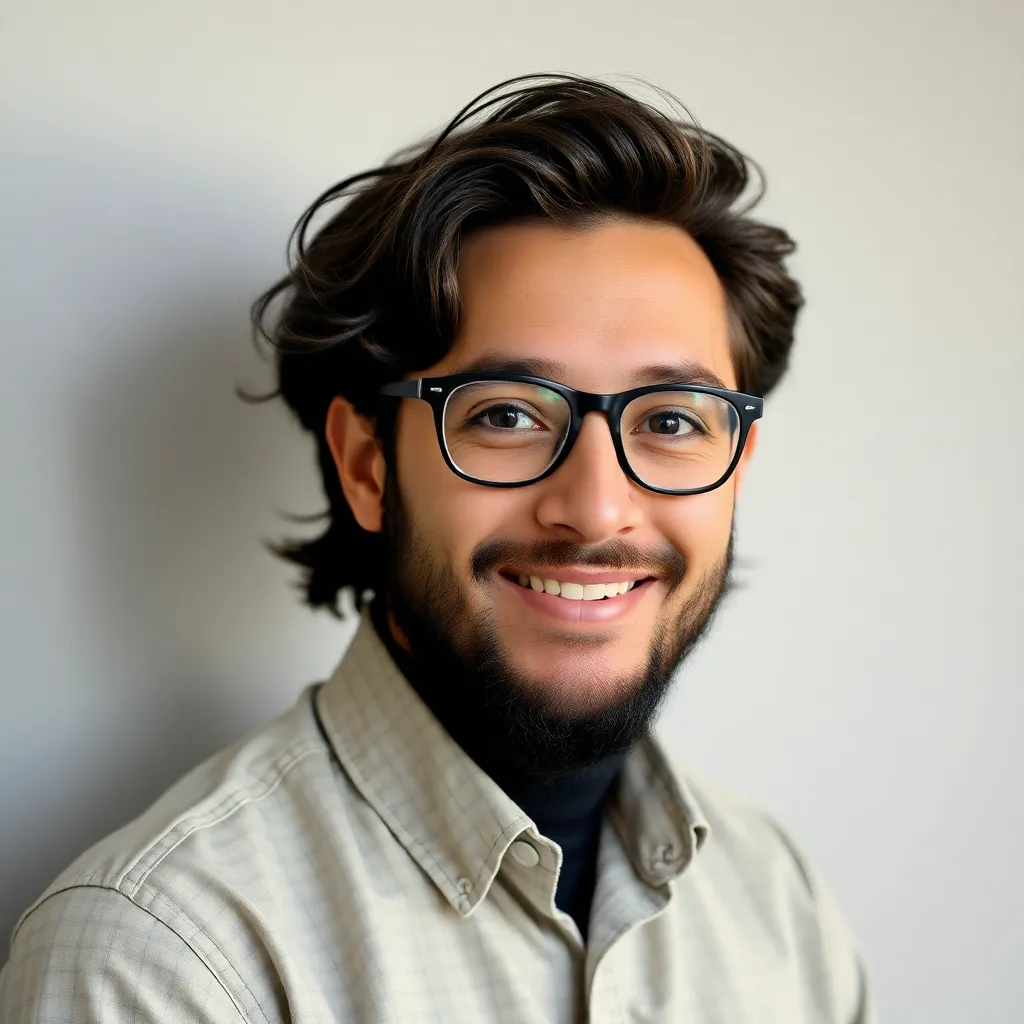
Onlines
Apr 25, 2025 · 6 min read

Table of Contents
Is the Dataset Approximately Periodic? A Comprehensive Guide to Periodicity Detection
Determining whether a dataset exhibits approximate periodicity is a fundamental task in many fields, from time series analysis in finance and climatology to signal processing in engineering and astronomy. Perfect periodicity, where a pattern repeats exactly at fixed intervals, is rare in real-world data. Instead, we often encounter approximate periodicity, where a pattern repeats with some variation or noise. This article provides a comprehensive guide to detecting approximate periodicity, covering both theoretical underpinnings and practical techniques.
Understanding Periodicity and its Challenges
Periodicity refers to the tendency of a phenomenon to repeat itself at regular intervals. A perfectly periodic signal can be represented by a simple sinusoidal function, but real-world data is rarely so clean. Noise, trends, and other confounding factors introduce deviations from perfect periodicity, making detection challenging. The challenge lies in distinguishing true approximate periodicity from random fluctuations.
Types of Periodicity
We can categorize periodicity into several types:
- Exact Periodicity: The signal repeats identically after a fixed time interval (period). This is ideal but rarely observed in real-world data.
- Approximate Periodicity: The signal repeats with variations in amplitude, phase, or period length. This is the most common type encountered in practice.
- Quasi-Periodicity: The signal exhibits a pattern that resembles periodicity but doesn't repeat exactly, often with variations in the interval between repetitions.
- Pseudo-Periodicity: The signal displays cyclical behavior, but the cycles are not strictly periodic, possibly due to complex underlying processes.
Challenges in Detecting Approximate Periodicity
Several factors complicate the detection of approximate periodicity:
- Noise: Random fluctuations obscure the underlying periodic pattern.
- Trends: Long-term changes in the data can mask the periodic component.
- Non-stationarity: The statistical properties of the data change over time, making it difficult to identify consistent patterns.
- Multiple Frequencies: The data may contain multiple periodic components with different periods, making it challenging to isolate individual patterns.
- Short Data Lengths: Insufficient data points can hinder the accurate estimation of the period.
Methods for Detecting Approximate Periodicity
Numerous techniques can be employed to detect approximate periodicity in datasets. The choice of method depends on the characteristics of the data and the desired level of accuracy.
1. Visual Inspection: Plotting the Data
The simplest approach is to plot the data against time. Visual inspection can reveal obvious periodic patterns, although it's subjective and unreliable for subtle periodicities or noisy data. Plotting autocorrelation or spectral density plots can complement visual inspection by highlighting potential periodicities.
2. Autocorrelation Function (ACF)
The ACF measures the correlation between a time series and a lagged version of itself. Significant peaks in the ACF at specific lags indicate potential periodicities. However, the ACF can be affected by trends and noise, and interpreting it requires careful consideration.
Advantages: Simple to implement and interpret. Disadvantages: Sensitive to noise and trends; may not accurately detect multiple periodicities.
3. Spectral Analysis (Fourier Transform)
The Fourier Transform decomposes a time series into its constituent frequencies. Prominent peaks in the power spectrum (the squared magnitude of the Fourier Transform) correspond to dominant frequencies, which can be related to periodicities. The Fast Fourier Transform (FFT) is a computationally efficient algorithm for calculating the Fourier Transform.
Advantages: Robust to noise; can detect multiple periodicities. Disadvantages: Assumes stationarity; may be sensitive to abrupt changes in the data.
4. Wavelet Transform
The Wavelet Transform is a powerful technique for analyzing non-stationary time series. It decomposes the data into different frequency bands and time scales, allowing for the detection of periodicities that vary over time. Wavelet transforms, such as the Continuous Wavelet Transform (CWT) and Discrete Wavelet Transform (DWT), can reveal hidden periodicities that are masked by noise and non-stationarity.
Advantages: Handles non-stationarity effectively; can detect localized periodicities. Disadvantages: More complex to interpret than Fourier Transform.
5. Lomb-Scargle Periodogram
The Lomb-Scargle periodogram is a powerful method for detecting periodicities in unevenly sampled time series, where data points are not acquired at regular intervals. It is particularly useful for astronomical data or other situations where regular sampling is difficult or impossible.
Advantages: Works well with unevenly sampled data; robust to noise. Disadvantages: Can be computationally intensive.
6. Autoregressive (AR) Models
AR models represent a time series as a linear combination of its past values. By fitting an AR model to the data, it's possible to identify periodicities reflected in the model's parameters.
7. Hidden Markov Models (HMMs)
HMMs are probabilistic models that can be used to model data with hidden states and observed emissions. They are useful when the periodicity is affected by unobserved factors influencing the underlying process. By modeling these hidden states, one can infer the periodicity.
Evaluating Periodicity Significance
Once potential periodicities are identified, it's crucial to assess their statistical significance. Techniques include:
- Significance Tests: Statistical tests, such as hypothesis tests on the periodogram peaks, can help determine if the detected periodicities are significantly different from random noise. The significance level is typically set at 0.05, meaning there's a 5% chance of a false positive.
- Confidence Intervals: Constructing confidence intervals around the estimated periods provides a measure of uncertainty. A narrower confidence interval indicates a more precise estimation of the period.
- False Discovery Rate (FDR): When multiple periodicities are detected, the FDR controls the proportion of false positives among the detected periods.
Practical Considerations and Advanced Techniques
- Data Preprocessing: Before applying periodicity detection methods, preprocessing steps like detrending (removing trends), denoising (reducing noise), and outlier removal are crucial to improve accuracy.
- Choosing the Right Method: The optimal method depends on the data's characteristics. For example, the Lomb-Scargle periodogram is suited for unevenly sampled data, while the FFT is more efficient for evenly sampled data.
- Combining Methods: Often, combining multiple methods provides a more robust and reliable detection of approximate periodicity. For instance, using ACF for initial detection and then confirming with FFT or Wavelet Transform.
- Non-linear Periodicity: Some datasets exhibit non-linear periodicity, where the period or amplitude varies non-linearly over time. Advanced techniques, such as recurrence quantification analysis (RQA) and nonlinear time series analysis, are needed to detect such patterns.
Conclusion
Detecting approximate periodicity in datasets is a multifaceted problem requiring careful consideration of the data's characteristics and the appropriate analytical techniques. Visual inspection provides a starting point, but more sophisticated methods such as the Fourier Transform, Wavelet Transform, Lomb-Scargle Periodogram, and advanced time series models are essential for robust and reliable detection. Always remember to assess the statistical significance of detected periodicities and consider the limitations of each method. By employing a combination of techniques and careful interpretation, you can effectively uncover hidden periodic patterns and gain valuable insights from your data. Remember that context is crucial; understanding the underlying process generating the data is essential for correctly interpreting the results of any periodicity analysis.
Latest Posts
Latest Posts
-
Summary Of Chapter 20 Of The Giver
Apr 26, 2025
-
Beyond Good And Evil Part 4
Apr 26, 2025
-
All Summer In A Day Characters
Apr 26, 2025
-
There Will Come Soft Rains Themes
Apr 26, 2025
-
Experiment 25 Ph Measurements Buffers And Their Properties
Apr 26, 2025
Related Post
Thank you for visiting our website which covers about Is The Data Set Approximately Periodic . We hope the information provided has been useful to you. Feel free to contact us if you have any questions or need further assistance. See you next time and don't miss to bookmark.