Isye 6501 Midterm 1 Cheat Sheet
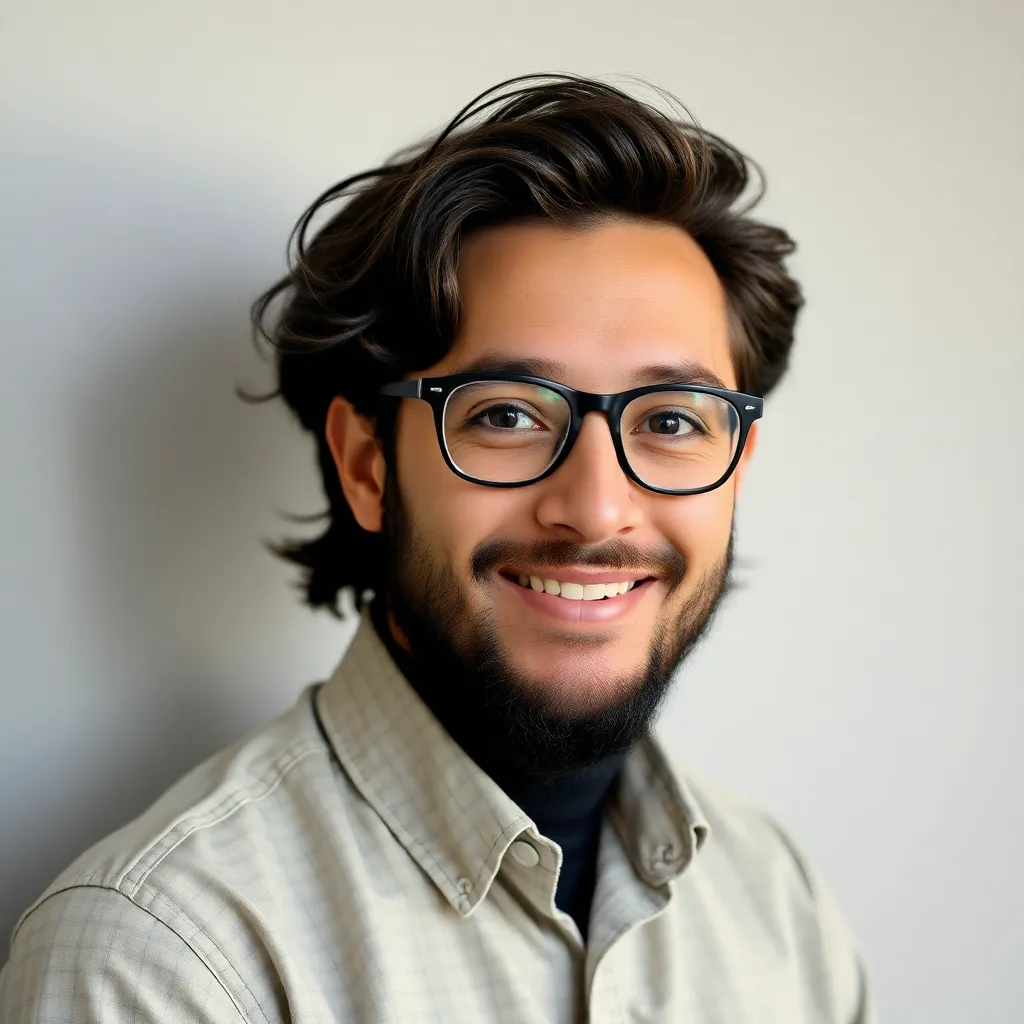
Onlines
Apr 15, 2025 · 6 min read

Table of Contents
ISYE 6501 Midterm 1 Cheat Sheet: A Comprehensive Guide to Conquering the Exam
The ISYE 6501 (Analytics Modeling) midterm exam is a significant hurdle for many students. This comprehensive cheat sheet aims to provide a structured and easily digestible overview of key concepts, formulas, and problem-solving strategies crucial for success. Remember, this cheat sheet serves as a supplement to your studies, not a replacement for thorough understanding of the material. Consistent effort throughout the semester is key.
I. Probability and Statistics Refresher
This section revisits fundamental concepts forming the bedrock of analytical modeling. A strong grasp of these is essential for tackling more complex topics later.
A. Descriptive Statistics:
- Mean: The average value. Formula: Σxᵢ / n (where xᵢ represents individual data points and n is the sample size).
- Median: The middle value when data is ordered. Useful for skewed distributions.
- Mode: The most frequent value. Can be multiple modes.
- Variance: Measures the spread of data around the mean. Formula: Σ(xᵢ - μ)² / (n-1) (sample variance) or Σ(xᵢ - μ)² / n (population variance). Note: (n-1) is used for sample variance due to Bessel's correction.
- Standard Deviation: The square root of the variance. Provides a more interpretable measure of spread in the same units as the data.
- IQR (Interquartile Range): Q3 - Q1 (the difference between the 75th and 25th percentiles). Resistant to outliers.
- Outliers: Data points significantly distant from the majority of the data. Often detected using box plots or z-scores.
B. Probability Distributions:
Understanding probability distributions is paramount. Knowing their properties, applications, and how to use them in different contexts will greatly benefit you. Here's a brief overview of some important distributions:
- Discrete Distributions:
- Bernoulli: Models a single binary outcome (success/failure).
- Binomial: Models the number of successes in a fixed number of independent Bernoulli trials. Key parameters: n (number of trials), p (probability of success).
- Poisson: Models the number of events occurring in a fixed interval of time or space. Key parameter: λ (average rate of events).
- Continuous Distributions:
- Normal (Gaussian): The ubiquitous bell curve. Characterized by its mean (μ) and standard deviation (σ). The Central Limit Theorem highlights its importance in statistics.
- Exponential: Models the time until an event occurs in a Poisson process. Key parameter: λ (rate parameter).
- Uniform: All values within a given range are equally likely.
C. Hypothesis Testing:
- Null Hypothesis (H₀): The statement being tested. Often a statement of "no effect."
- Alternative Hypothesis (H₁ or Hₐ): The statement you are trying to find evidence for.
- p-value: The probability of observing the data (or more extreme data) if the null hypothesis is true. A small p-value (typically < 0.05) suggests evidence against the null hypothesis.
- Type I Error: Rejecting the null hypothesis when it is true. (False positive)
- Type II Error: Failing to reject the null hypothesis when it is false. (False negative)
- Confidence Intervals: A range of values within which the true population parameter is likely to lie with a certain level of confidence (e.g., 95%).
II. Regression Analysis: The Core of ISYE 6501
Regression analysis is the heart of many analytical modeling techniques. Understanding its principles, assumptions, and applications is crucial.
A. Simple Linear Regression:
- Model: Y = β₀ + β₁X + ε (Y is the dependent variable, X is the independent variable, β₀ is the intercept, β₁ is the slope, and ε is the error term).
- Least Squares Estimation: The method used to find the best-fitting line that minimizes the sum of squared errors.
- R-squared: A measure of the goodness of fit, representing the proportion of variance in Y explained by X.
- Assumptions: Linearity, independence of errors, homoscedasticity (constant variance of errors), normality of errors.
- Interpreting Coefficients: Understanding the meaning of β₀ and β₁ in the context of the problem.
B. Multiple Linear Regression:
- Model: Y = β₀ + β₁X₁ + β₂X₂ + ... + βₖXₖ + ε (multiple independent variables).
- Interpretation of Coefficients: Understanding the effect of each independent variable on the dependent variable, holding other variables constant (ceteris paribus).
- Multicollinearity: High correlation between independent variables, leading to unstable coefficient estimates.
- Model Selection: Techniques for choosing the best subset of independent variables (e.g., stepwise regression, AIC, BIC).
C. Regression Diagnostics:
- Residual Plots: Used to check the assumptions of linear regression (linearity, constant variance, normality of errors).
- Influential Points: Data points that have a large impact on the regression results. Leverage and Cook's distance are commonly used to identify them.
- Heteroscedasticity: Non-constant variance of errors. Can be addressed through transformations or weighted least squares.
III. Optimization Techniques: Finding the Best Solution
Optimization forms a vital part of ISYE 6501. Grasping the core concepts will equip you to solve complex problems efficiently.
A. Linear Programming (LP):
- Formulation: Defining the objective function (to be maximized or minimized) and constraints.
- Graphical Method: Solving small LPs graphically.
- Simplex Method: An iterative algorithm used to solve larger LPs. (Understanding the underlying principles is more important than the rote memorization of the algorithm itself).
- Duality: The relationship between a primal LP and its dual. Understanding duality helps with interpretation and sensitivity analysis.
B. Integer Programming (IP):
- Binary Variables: Variables that can only take on values of 0 or 1.
- Integer Variables: Variables that can only take on integer values.
- Branch and Bound: A common algorithm used to solve IPs.
- Applications: Many real-world problems involve integer decision variables (e.g., assigning workers to tasks, facility location).
C. Non-Linear Programming (NLP):
- Gradient Descent: An iterative optimization algorithm used to find local optima in NLP problems.
IV. Simulation and Modeling
This section focuses on using simulation to analyze complex systems.
A. Monte Carlo Simulation:
- Random Number Generation: Generating random numbers from various probability distributions.
- Simulating Stochastic Processes: Using random numbers to model uncertain events.
- Estimating Statistics: Using simulation to estimate the mean, variance, and other statistics of interest.
B. Discrete Event Simulation:
- Events: Occurrences that change the state of the system.
- Event Scheduling: Managing the timing of events.
- Applications: Modeling queuing systems, supply chains, manufacturing processes.
V. Forecasting Methods
Accurate forecasting is crucial for effective decision-making. Understanding the key forecasting methods is important.
A. Time Series Decomposition:
- Trend: The long-term pattern in the data.
- Seasonality: Recurring patterns within a fixed period (e.g., monthly, yearly).
- Cyclical Variations: Long-term fluctuations that are not necessarily periodic.
- Irregular Components: Random fluctuations in the data.
B. Moving Averages:
- Simple Moving Average: The average of the last 'n' data points.
- Weighted Moving Average: Assigns different weights to different data points.
- Exponential Smoothing: A method that gives more weight to recent data points.
C. ARIMA Models:
Understanding the basics of ARIMA models (Autoregressive Integrated Moving Average) is beneficial but a deeper dive might be beyond the scope of the midterm. Focus on the key concepts rather than complex model specifications.
VI. Important Reminders for Exam Success
- Practice Problems: Solve a wide variety of problems from the textbook, lecture notes, and practice exams. This is crucial.
- Understand Concepts: Don't just memorize formulas; understand the underlying concepts and intuition behind them.
- Review Your Notes: Thoroughly review your lecture notes and textbook readings.
- Form Study Groups: Collaborating with classmates can enhance understanding and provide different perspectives.
- Manage Your Time: Allocate sufficient time for studying each topic. Prioritize areas where you feel less confident.
- Stay Calm: On the day of the exam, stay calm and focus on what you know. If you encounter a challenging question, move on and come back to it later.
This cheat sheet provides a comprehensive overview of key topics for the ISYE 6501 Midterm 1. Remember, effective learning requires a combination of understanding the concepts, practicing problem-solving, and managing your time efficiently. Good luck!
Latest Posts
Latest Posts
-
Which Statement Best Describes How An Author Uses Indirect Characterization
Apr 15, 2025
-
Ode To The West Wind Summary
Apr 15, 2025
-
Tier 3 Investigation Is Designated For The Following Positions
Apr 15, 2025
-
Protein Synthesis Escape Room Answer Key
Apr 15, 2025
-
Using Multiple Medications Before Ambulating Can Affect
Apr 15, 2025
Related Post
Thank you for visiting our website which covers about Isye 6501 Midterm 1 Cheat Sheet . We hope the information provided has been useful to you. Feel free to contact us if you have any questions or need further assistance. See you next time and don't miss to bookmark.