Match The Scale Type To Its Corresponding Description.
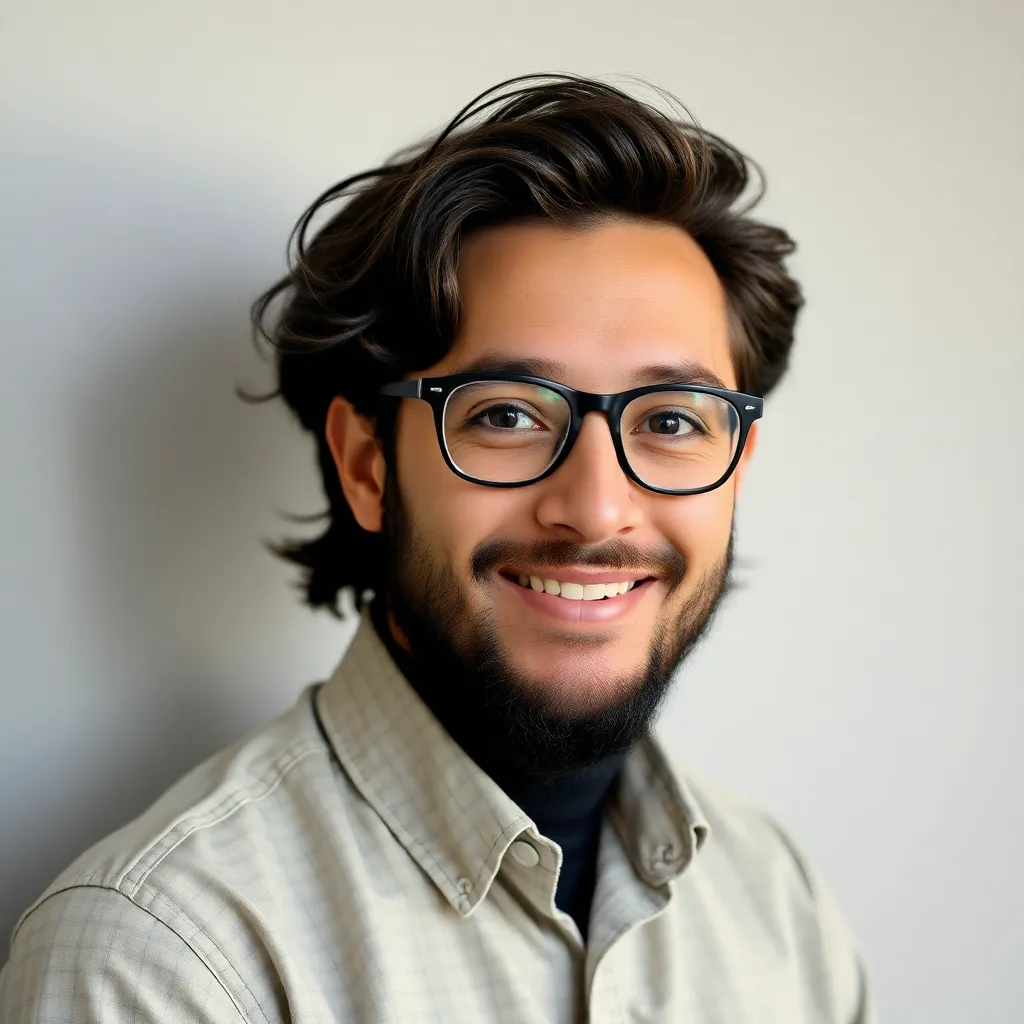
Onlines
Apr 16, 2025 · 7 min read

Table of Contents
Match the Scale Type to its Corresponding Description: A Comprehensive Guide
Choosing the right scale type for your research is crucial for obtaining meaningful and reliable results. Understanding the nuances of each scale—nominal, ordinal, interval, and ratio—is paramount for accurate data analysis and interpretation. This comprehensive guide will delve into each scale type, providing clear descriptions, examples, and highlighting their strengths and limitations. We'll also explore how to correctly match a scale type to its description, ensuring your research methodology is robust and your conclusions are valid.
Understanding the Four Levels of Measurement
The four fundamental levels of measurement—nominal, ordinal, interval, and ratio—differ significantly in the type of information they provide. Choosing the incorrect scale can lead to misinterpretations and flawed conclusions. Let's examine each one in detail:
1. Nominal Scale: Categorical Data with No Order
The nominal scale is the most basic level of measurement. It categorizes data into distinct groups without implying any order or ranking. The numbers assigned to these categories are simply labels and don't represent any quantitative value.
Key Characteristics:
- Categorical data: Data is grouped into distinct categories.
- No inherent order: There's no meaningful ranking or hierarchy among categories.
- Qualitative data: Primarily represents qualities or characteristics.
Examples:
- Gender: Male, Female, Other
- Eye color: Brown, Blue, Green, Hazel
- Country of origin: USA, Canada, Mexico, etc.
- Favorite color: Red, Blue, Green, etc.
Limitations:
- Cannot perform arithmetic operations (addition, subtraction, etc.).
- Cannot calculate meaningful statistics like mean or standard deviation.
- Only suitable for descriptive statistics like frequencies and percentages.
Appropriate Statistical Analyses:
- Mode (most frequent category)
- Frequency counts
- Contingency tables
- Chi-square test
2. Ordinal Scale: Ranked Data with Unequal Intervals
The ordinal scale categorizes data and also ranks them in a specific order. However, the intervals between these ranks are not necessarily equal.
Key Characteristics:
- Ranked data: Categories are ordered based on a specific characteristic.
- Unequal intervals: The difference between ranks isn't necessarily consistent.
- Qualitative or quantitative data: Can represent both.
Examples:
- Educational level: High school, Bachelor's degree, Master's degree, PhD
- Customer satisfaction: Very satisfied, Satisfied, Neutral, Dissatisfied, Very dissatisfied
- Socioeconomic status: Low, Middle, High
- Rankings in a competition: 1st, 2nd, 3rd, etc.
Limitations:
- Cannot perform arithmetic operations.
- The difference between ranks isn't quantifiable.
- Limited statistical analysis compared to interval and ratio scales.
Appropriate Statistical Analyses:
- Median (middle value)
- Percentiles
- Rank correlation (Spearman's rank correlation)
- Non-parametric tests (e.g., Mann-Whitney U test, Kruskal-Wallis test)
3. Interval Scale: Ranked Data with Equal Intervals and Arbitrary Zero Point
The interval scale maintains the properties of ordinal scales (ranking and order) but adds the crucial element of equal intervals between points. However, the zero point on an interval scale is arbitrary and doesn't represent the absence of the measured attribute.
Key Characteristics:
- Ranked data with equal intervals: The difference between consecutive points is consistent.
- Arbitrary zero point: Zero doesn't indicate the complete absence of the measured attribute.
- Quantitative data: Represents numerical values.
Examples:
- Temperature in Celsius or Fahrenheit: The difference between 10°C and 20°C is the same as the difference between 20°C and 30°C. However, 0°C doesn't mean the absence of temperature.
- Year: The difference between 2022 and 2023 is the same as the difference between 1900 and 1901. However, year 0 doesn't represent the absence of time.
- IQ scores: Equal intervals between scores, but a score of 0 doesn't mean the absence of intelligence.
Limitations:
- Ratios are not meaningful. For instance, 20°C is not twice as hot as 10°C.
Appropriate Statistical Analyses:
- Mean (average)
- Standard deviation
- Variance
- Correlation (Pearson's correlation)
- T-tests
- ANOVA
4. Ratio Scale: Ranked Data with Equal Intervals and a True Zero Point
The ratio scale represents the highest level of measurement. It incorporates all the characteristics of interval scales but adds a true zero point. This true zero point signifies the absence of the measured attribute.
Key Characteristics:
- Ranked data with equal intervals: The difference between consecutive points is consistent.
- True zero point: Zero indicates the complete absence of the measured attribute.
- Quantitative data: Represents numerical values.
Examples:
- Height: A height of 0 cm indicates the absence of height.
- Weight: A weight of 0 kg indicates the absence of weight.
- Income: An income of $0 indicates the absence of income.
- Age: An age of 0 years indicates the absence of age.
Limitations:
- Relatively few limitations compared to other scales.
Appropriate Statistical Analyses:
- All statistical analyses applicable to interval scales are also applicable to ratio scales.
- Ratios are meaningful. For example, a person weighing 100 kg weighs twice as much as a person weighing 50 kg.
- Geometric mean
Matching Scale Types to Descriptions: Practical Examples
Let's solidify our understanding by matching some descriptions to their corresponding scale types:
Example 1:
Description: Participants are asked to rate their satisfaction with a product on a scale from 1 (Very Dissatisfied) to 5 (Very Satisfied).
Scale Type: Ordinal. While there's a clear order, the intervals between levels of satisfaction aren't necessarily equal. The difference between "Very Dissatisfied" and "Dissatisfied" might not be the same as the difference between "Satisfied" and "Very Satisfied".
Example 2:
Description: Researchers record the number of hours students spend studying each week.
Scale Type: Ratio. Zero hours of study represents a true absence of study time. The intervals between hours are equal.
Example 3:
Description: Participants are asked to indicate their favorite type of music from a list of genres (Pop, Rock, Classical, Jazz).
Scale Type: Nominal. There's no inherent order or ranking among the music genres.
Example 4:
Description: Participants are categorized into age groups: 18-25, 26-35, 36-45, 46-55, 56+.
Scale Type: Ordinal. The age groups are ordered, but the intervals are not equal (the first group is 8 years, while others are 10 years).
Example 5:
Description: Researchers measure the temperature of a room in degrees Celsius.
Scale Type: Interval. There is an equal interval between each degree, but 0 degrees Celsius doesn't represent the absence of temperature.
Choosing the Right Scale: Implications for Data Analysis
The choice of scale directly impacts the types of statistical analyses you can perform. Using inappropriate statistical techniques can lead to misleading results. Always consider the nature of your data and the research question when choosing a scale.
For instance, calculating the mean of nominal data is meaningless. Similarly, using parametric tests (like t-tests or ANOVA) on ordinal data can be inappropriate unless certain assumptions are met. Understanding the strengths and limitations of each scale is crucial for ensuring the validity and reliability of your research findings.
Beyond the Basics: Advanced Considerations
While the four basic scale types provide a solid foundation, some research situations might involve more complex scales or combinations of scales. For instance, a Likert scale, often used to measure attitudes or opinions, typically falls under the ordinal scale, although some researchers argue for its treatment as an interval scale under specific conditions. Understanding these nuances requires a deeper dive into psychometrics and statistical theory.
Furthermore, consider the potential for errors in measurement. Even with the correct scale type, issues like response bias, sampling error, and instrument reliability can affect the accuracy of your data. Addressing these potential sources of error is essential for drawing valid conclusions.
Conclusion: Mastering Scale Types for Robust Research
Matching the scale type to its corresponding description is a fundamental aspect of research methodology. A thorough understanding of the four levels of measurement—nominal, ordinal, interval, and ratio—is essential for designing effective studies, conducting appropriate analyses, and drawing valid conclusions. By carefully considering the nature of your data and the research question, you can choose the right scale and ensure the robustness and reliability of your findings. Remember that the choice of scale directly impacts the types of statistical analyses that can be performed, highlighting the importance of selecting the appropriate scale type from the outset. By mastering this crucial aspect of research, you can enhance the quality and impact of your work significantly.
Latest Posts
Latest Posts
-
A Client Is Prescribed A Second Dose Of Iv Ceftriaxone
Apr 16, 2025
-
Vanessa Jason Biology Roots Answer Key Pdf
Apr 16, 2025
-
Marios Math Tutoring Act Equations Sheet
Apr 16, 2025
-
What Is The Appropriate Description For A Distributorship
Apr 16, 2025
-
Which Statement Regarding Urinary Tract Infections Is False
Apr 16, 2025
Related Post
Thank you for visiting our website which covers about Match The Scale Type To Its Corresponding Description. . We hope the information provided has been useful to you. Feel free to contact us if you have any questions or need further assistance. See you next time and don't miss to bookmark.