Predictive Analytics Is Used For All Of The Following Except
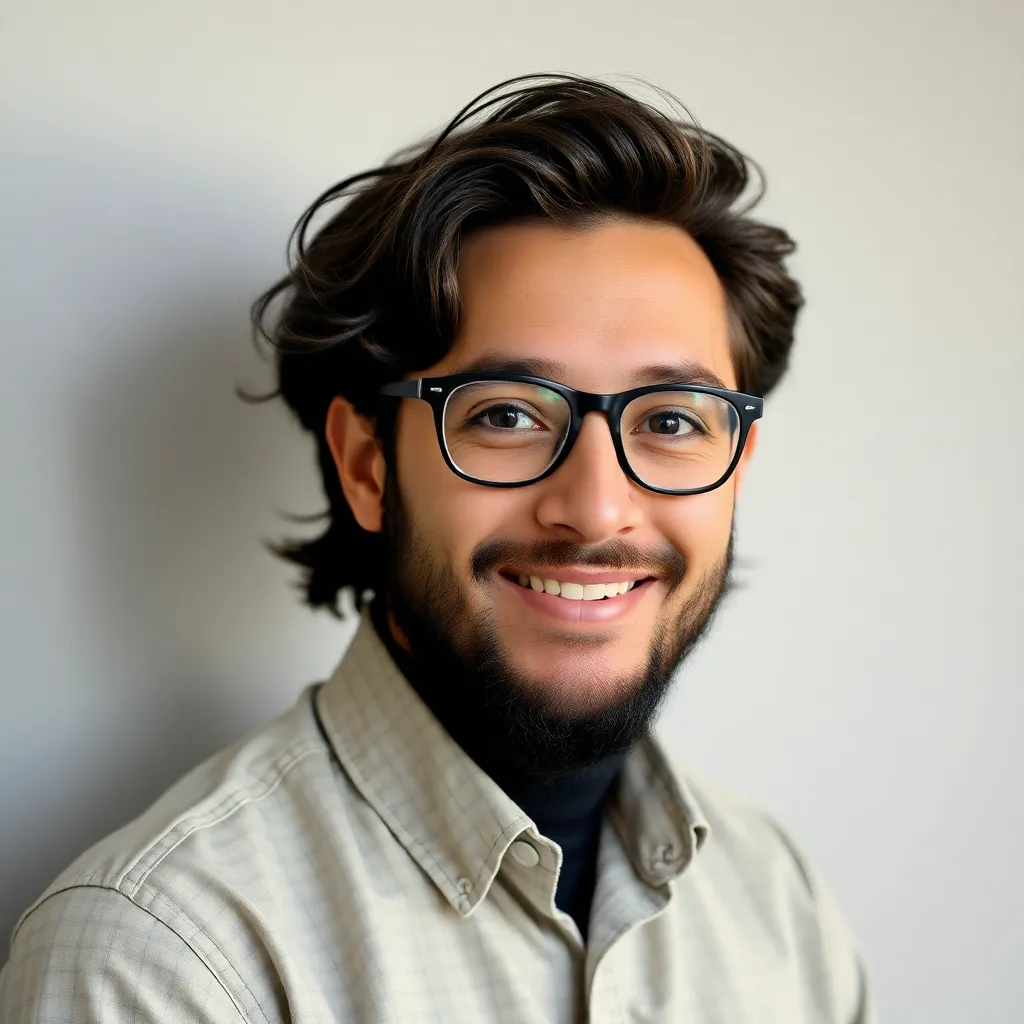
Onlines
May 10, 2025 · 6 min read
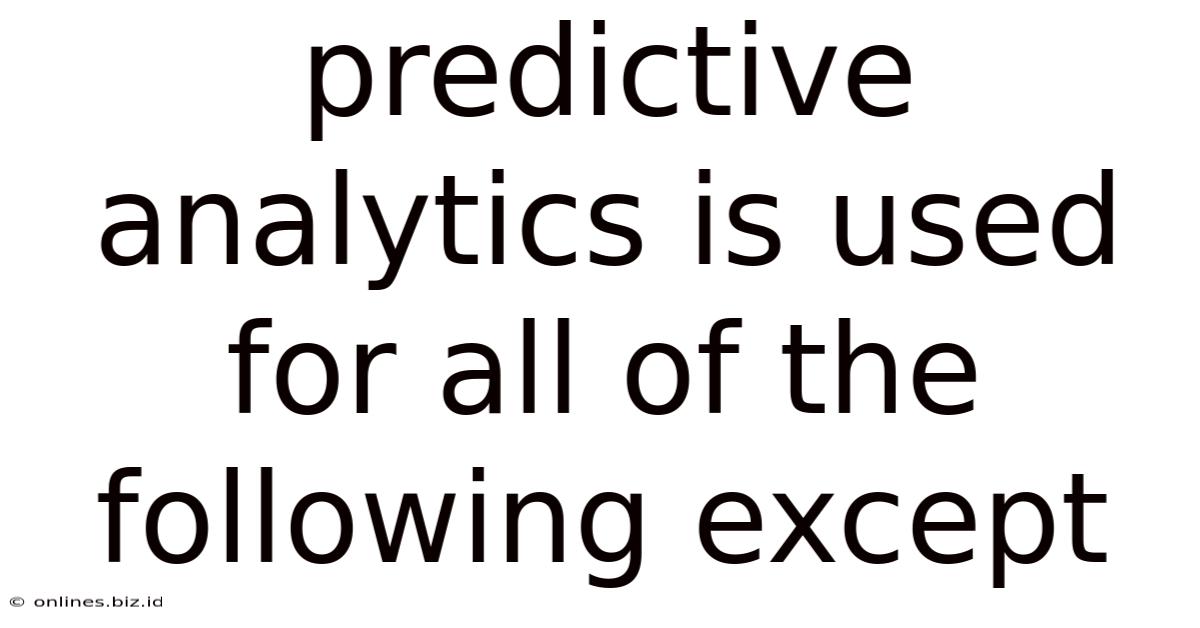
Table of Contents
Predictive Analytics: Applications and Exclusions
Predictive analytics, a powerful tool leveraging data mining, statistics, and machine learning to forecast future outcomes, is transforming numerous industries. Its ability to analyze historical data and identify patterns allows businesses to make informed decisions, optimize operations, and gain a competitive edge. However, despite its widespread applicability, there are limitations. This article explores the diverse applications of predictive analytics and importantly, identifies what it cannot do.
Where Predictive Analytics Shines: A Spectrum of Applications
Predictive analytics finds its strengths in scenarios involving quantifiable data and identifiable patterns. Let's delve into some key areas:
1. Customer Relationship Management (CRM)
- Targeted Marketing: By analyzing customer purchase history, demographics, and website behavior, predictive models identify high-potential customers and tailor marketing campaigns for maximum impact. This leads to increased conversion rates and customer lifetime value.
- Churn Prediction: Identifying customers at risk of churning allows businesses to proactively intervene with retention strategies, preventing revenue loss. This involves analyzing factors like engagement levels, customer service interactions, and recent purchases.
- Personalized Recommendations: E-commerce platforms leverage predictive analytics to suggest relevant products and services to individual users, boosting sales and enhancing the customer experience. This is based on past purchases, browsing history, and similar user behavior.
2. Risk Management and Fraud Detection
- Credit Scoring: Financial institutions use predictive models to assess creditworthiness, minimizing loan defaults and optimizing lending strategies. Factors such as income, debt, and credit history are analyzed.
- Fraud Detection: By identifying unusual patterns in transactions, predictive analytics helps detect fraudulent activities in real-time, protecting businesses and customers from financial losses. This often involves anomaly detection algorithms.
- Insurance Risk Assessment: Insurers leverage predictive analytics to assess risk profiles, determine premiums, and manage claims more effectively. Factors like age, health, location, and driving history are key inputs.
3. Supply Chain Optimization
- Demand Forecasting: Accurate prediction of future demand allows businesses to optimize inventory levels, reducing storage costs and preventing stockouts. This involves analyzing historical sales data, seasonal trends, and external factors like economic conditions.
- Supply Chain Disruption Prediction: By monitoring various factors, predictive models can anticipate potential disruptions, such as supplier delays or natural disasters, allowing businesses to develop contingency plans and mitigate risks.
- Logistics Optimization: Predictive analytics can optimize transportation routes, delivery schedules, and warehouse management, improving efficiency and reducing costs.
4. Healthcare and Medicine
- Disease Prediction and Prevention: Analyzing patient data, lifestyle factors, and genetic information helps predict the likelihood of developing certain diseases, enabling early intervention and preventative measures.
- Personalized Medicine: Predictive analytics assists in tailoring treatment plans based on individual patient characteristics, optimizing treatment efficacy and reducing side effects.
- Hospital Resource Allocation: Predicting patient flow and resource needs helps hospitals optimize staffing levels, bed allocation, and equipment utilization, improving efficiency and patient care.
5. Financial Modeling and Investment Strategies
- Stock Price Prediction: While not perfectly accurate, predictive analytics can help investors identify potential investment opportunities by analyzing historical stock prices, market trends, and economic indicators. This is often combined with other forms of financial analysis.
- Portfolio Optimization: Predictive models can help investors construct diversified portfolios that maximize returns while minimizing risk.
- Risk Assessment in Investments: Analyzing various market factors and economic indicators allows investors to assess potential risks associated with different investment strategies.
What Predictive Analytics CANNOT Do: Limitations and Misconceptions
While predictive analytics offers significant advantages, it's crucial to understand its limitations. It's not a crystal ball, and its predictions are based on past data and identified patterns. Here are some key areas where predictive analytics falls short:
1. Predicting Truly Novel Events: Black Swan Events
Predictive models excel at forecasting events that fall within the range of historical data. However, they struggle with truly unprecedented events, often referred to as "black swan events." These are unpredictable, high-impact occurrences that lie outside the scope of past observations. Examples include unexpected economic crises, natural disasters of unprecedented scale, or the sudden emergence of a completely novel technology that disrupts existing markets. Predictive models, by their nature, cannot foresee events that have never happened before.
2. Accounting for Human Behavior: Unpredictability of Individuals
While predictive models can identify trends in human behavior, they often struggle to accurately predict the actions of individuals. Human behavior is complex and influenced by a multitude of factors, including emotions, biases, and unpredictable circumstances. This limits the accuracy of predictions in areas like consumer behavior, political forecasting, and even certain aspects of healthcare. Models may provide probabilities, but individual actions remain inherently difficult to forecast with certainty.
3. Guaranteed Accuracy: The Role of Uncertainty
Predictive analytics provides probabilities, not certainties. The accuracy of predictions depends on the quality and quantity of data, the appropriateness of the model used, and the inherent randomness in the phenomena being modeled. Over-reliance on predictive models without considering the inherent uncertainty can lead to poor decision-making. It is crucial to understand the confidence intervals and error margins associated with any prediction.
4. Causality vs. Correlation: Understanding the "Why"
Predictive models identify correlations between variables but don't necessarily establish causality. Just because two variables are correlated doesn't mean one causes the other. Understanding the underlying causal relationships requires additional investigation and domain expertise. For example, a correlation between ice cream sales and crime rates doesn't imply that ice cream consumption causes crime. Both are likely influenced by a third variable, such as warmer weather. Predictive analytics highlights the correlation but cannot definitively explain the causation.
5. Data Bias and Model Limitations: Garbage In, Garbage Out
The accuracy of predictive models heavily relies on the quality of the data used to train them. Biased or incomplete data can lead to inaccurate and unreliable predictions. Furthermore, the choice of model itself can influence the results. Using an inappropriate model for the data can lead to inaccurate or misleading predictions. The principle of "garbage in, garbage out" applies strongly to predictive analytics. Careful data cleaning, feature engineering, and model selection are critical for obtaining reliable results.
6. Ethical Considerations and Bias Amplification
The use of predictive analytics raises ethical concerns, particularly regarding bias. Models trained on biased data can perpetuate and even amplify existing societal biases, leading to unfair or discriminatory outcomes. For example, a model used for loan applications that is trained on historically biased data may unfairly discriminate against certain demographic groups. Addressing these ethical concerns requires careful attention to data fairness, model transparency, and responsible deployment.
7. Explaining Complex Predictions: The Black Box Problem
Some advanced machine learning models, particularly deep learning algorithms, are often referred to as "black boxes" because their decision-making processes are difficult to understand. This lack of transparency can make it challenging to explain or interpret the predictions generated by these models, hindering trust and accountability. This opacity can be particularly problematic in high-stakes applications like healthcare and criminal justice.
Conclusion: Harnessing the Power Responsibly
Predictive analytics is a powerful tool with the potential to revolutionize various industries. However, it's crucial to understand its limitations. While it excels at forecasting trends and identifying patterns based on historical data, it cannot predict truly novel events, guarantee accuracy, definitively establish causality, or account for the complexities of human behavior. Responsible use of predictive analytics requires careful consideration of data quality, model selection, ethical implications, and the inherent uncertainties associated with prediction. By understanding both the strengths and limitations of this powerful tool, we can harness its capabilities effectively and responsibly, maximizing its benefits while mitigating its risks.
Latest Posts
Latest Posts
-
Which Option Uses Dialogue Most Effectively
May 10, 2025
-
How Is The Word Pl Ay Divided In This Example
May 10, 2025
-
Identity By Julio Noboa Polanco Answer Key
May 10, 2025
-
Suppose A Pigeon That Is Homozygous For The Grouse Allele
May 10, 2025
-
Espresso Express Operates A Number Of
May 10, 2025
Related Post
Thank you for visiting our website which covers about Predictive Analytics Is Used For All Of The Following Except . We hope the information provided has been useful to you. Feel free to contact us if you have any questions or need further assistance. See you next time and don't miss to bookmark.