Quantitative Methods For Business 13th Edition
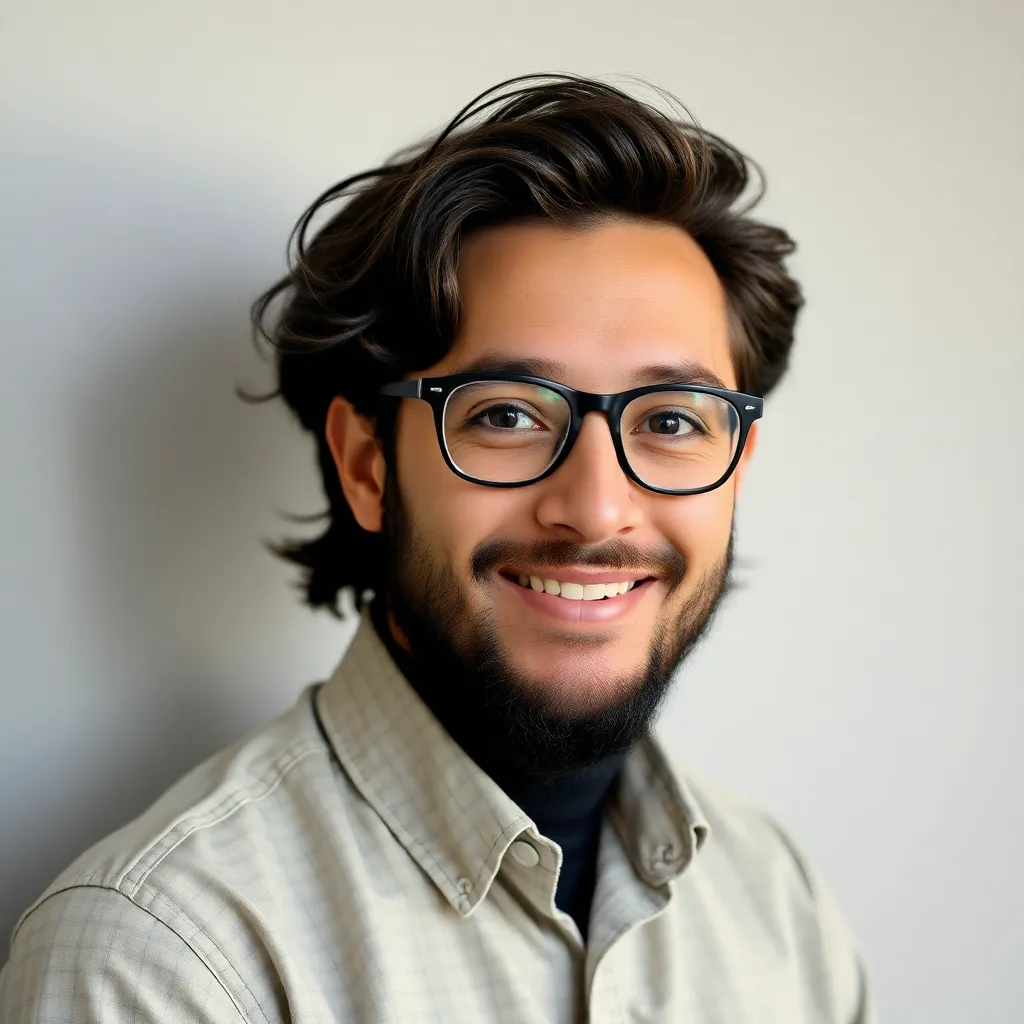
Onlines
May 10, 2025 · 7 min read
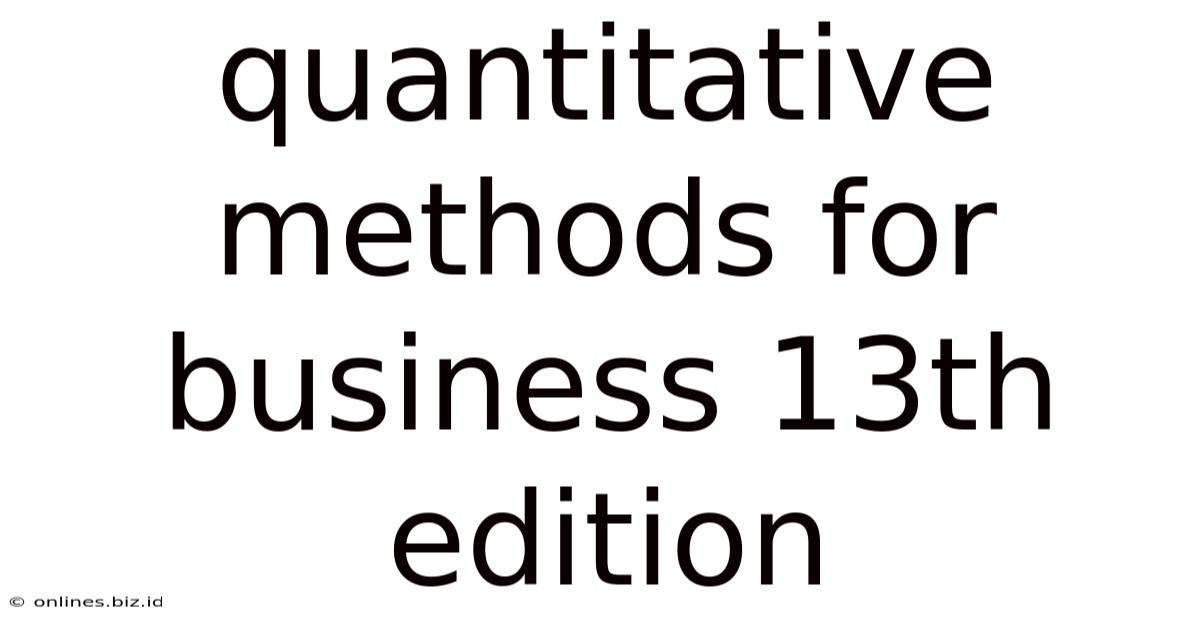
Table of Contents
Quantitative Methods for Business, 13th Edition: A Comprehensive Guide
Quantitative methods are essential tools for businesses seeking to make informed decisions, understand market trends, and optimize their operations. This comprehensive guide delves into the core concepts and applications of quantitative methods as presented in the 13th edition of a leading textbook on the subject (note: I cannot reference a specific textbook title as I lack the ability to access external websites or specific files). We'll explore key statistical techniques, their business applications, and how to effectively interpret the results.
Understanding the Fundamentals of Quantitative Methods
Before diving into specific techniques, it's crucial to grasp the underlying principles. Quantitative methods rely on numerical data to analyze patterns, relationships, and trends. This involves collecting, cleaning, analyzing, and interpreting data to answer specific business questions. The process typically involves:
1. Defining the Research Question:
This is the cornerstone of any quantitative analysis. A clearly defined question guides the entire process, determining the type of data needed and the appropriate statistical methods to use. A well-defined research question should be specific, measurable, achievable, relevant, and time-bound (SMART). For example, instead of asking "Is our marketing campaign effective?", a better question would be "What is the conversion rate of our current Facebook ad campaign compared to our previous campaign, and by what percentage has it changed?"
2. Data Collection:
Appropriate data collection methods depend on the research question. Common methods include surveys, experiments, observational studies, and accessing existing databases. The quality of the data directly impacts the reliability of the results. It's essential to consider sampling methods to ensure the data represents the target population accurately. Bias in data collection should be minimized through careful planning and execution.
3. Data Cleaning and Preparation:
Raw data often contains errors, inconsistencies, and missing values. Data cleaning is a crucial step involving identifying and correcting these issues. This may involve removing outliers, imputing missing values, and transforming data into a suitable format for analysis.
4. Data Analysis:
This stage involves applying appropriate statistical techniques to answer the research question. The choice of technique depends on the type of data (e.g., continuous, categorical) and the research question. Common techniques include descriptive statistics, regression analysis, hypothesis testing, and time series analysis.
5. Interpretation and Reporting:
The final stage involves interpreting the results of the analysis and communicating them effectively to stakeholders. This requires a clear understanding of the statistical concepts and the ability to present findings in a concise and meaningful way. Visualizations (charts, graphs) are essential for communicating complex information effectively.
Key Quantitative Methods Used in Business
The 13th edition likely covers a range of quantitative methods crucial for various business functions. Let's explore some key techniques:
1. Descriptive Statistics:
These methods summarize and describe the main features of a dataset. They provide insights into the central tendency (mean, median, mode), dispersion (range, variance, standard deviation), and shape of the data distribution. Descriptive statistics are fundamental for understanding basic patterns in data before moving to more complex analyses. They are used extensively in business reporting, market research, and performance monitoring.
2. Regression Analysis:
This powerful technique examines the relationship between a dependent variable and one or more independent variables. Linear regression is commonly used to model linear relationships, allowing businesses to predict future outcomes based on historical data. For example, a company could use regression analysis to predict sales based on advertising spending, pricing, or economic indicators. Multiple regression allows for the consideration of multiple independent variables, providing a more comprehensive understanding of complex relationships.
3. Hypothesis Testing:
This is a crucial method for making inferences about a population based on sample data. Businesses use hypothesis testing to evaluate the effectiveness of marketing campaigns, compare the performance of different products, or assess the impact of changes in operations. It involves formulating a null hypothesis (a statement of no effect) and an alternative hypothesis and then using statistical tests (t-tests, ANOVA, chi-square tests) to determine whether there is sufficient evidence to reject the null hypothesis.
4. Time Series Analysis:
This technique analyzes data collected over time to identify trends, seasonality, and cyclical patterns. It is particularly useful for forecasting future outcomes, such as sales, demand, or stock prices. Methods like moving averages, exponential smoothing, and ARIMA models are commonly employed in time series analysis. Understanding seasonal trends allows businesses to optimize inventory management, staffing levels, and marketing campaigns.
5. Forecasting Methods:
Predicting future outcomes is a core function of quantitative methods in business. Various forecasting methods exist, ranging from simple moving averages to sophisticated econometric models. The choice of method depends on the complexity of the data, the desired accuracy, and the available resources. Accurate forecasting is crucial for inventory management, production planning, financial planning, and strategic decision-making.
6. Probability and Distributions:
Understanding probability and various probability distributions is fundamental to many quantitative methods. This knowledge is crucial for risk assessment, decision-making under uncertainty, and quality control. Different distributions, such as the normal, binomial, and Poisson distributions, are used to model various phenomena in business.
Advanced Quantitative Methods
The 13th edition likely also covers more advanced quantitative methods relevant to specialized business applications:
1. Econometrics:
This field applies statistical methods to economic data to analyze economic relationships and test economic theories. Businesses can use econometric techniques to model market demand, forecast economic indicators, and evaluate the impact of economic policies on their operations.
2. Operations Research:
This field uses mathematical models and algorithms to optimize business processes and decision-making. Techniques like linear programming, simulation, and queuing theory are used to improve efficiency, reduce costs, and enhance decision-making in areas such as supply chain management, logistics, and production planning.
3. Data Mining and Machine Learning:
With the proliferation of big data, data mining and machine learning techniques are increasingly important for businesses. These methods involve using advanced algorithms to extract patterns, insights, and predictions from large datasets. Applications include customer segmentation, fraud detection, risk management, and personalized recommendations.
Interpreting Results and Avoiding Common Pitfalls
The success of quantitative methods hinges not only on the correct application of techniques but also on the accurate interpretation of results and the awareness of potential pitfalls.
-
Context Matters: Statistical significance doesn't always equate to practical significance. A statistically significant result might be too small to be of real-world importance. Always consider the context of your findings and their relevance to the business problem.
-
Causation vs. Correlation: Correlation between variables doesn't imply causation. While regression analysis can show relationships, it doesn't prove that one variable causes changes in another. Careful consideration of other factors and potential confounding variables is crucial.
-
Data Quality: Garbage in, garbage out. The accuracy of your results depends heavily on the quality of your data. Thorough data cleaning and validation are paramount.
-
Assumptions: Many statistical methods rely on certain assumptions about the data (e.g., normality, independence). Violating these assumptions can lead to inaccurate or misleading results. It's crucial to assess the validity of these assumptions before applying the chosen techniques.
-
Overfitting: In machine learning, overfitting occurs when a model fits the training data too well but performs poorly on new, unseen data. Techniques like cross-validation are used to prevent overfitting and ensure the model generalizes well to real-world scenarios.
-
Communicating Results Effectively: The value of your analysis is limited unless you can communicate your findings clearly and concisely to stakeholders. Use clear language, visualizations, and avoid technical jargon where possible.
Conclusion
Quantitative methods are indispensable tools for modern businesses navigating a complex and data-rich environment. Mastering these methods equips professionals with the skills to analyze data, draw insightful conclusions, and make informed decisions that drive business success. The 13th edition of a leading quantitative methods textbook provides a strong foundation in these vital techniques, enabling students and professionals to apply these powerful tools to solve real-world business problems. By carefully applying these methods and understanding their limitations, businesses can unlock valuable insights, optimize their operations, and achieve a competitive advantage in the marketplace. Remember that continuous learning and adaptation are crucial as new techniques and data sources emerge in the ever-evolving business landscape.
Latest Posts
Latest Posts
-
Eye Of The Tiger Figurative Language
May 10, 2025
-
If There Is No Variation In Shell Thickness
May 10, 2025
-
Colonists In New England Participated In Congregational Hymn Singing
May 10, 2025
-
A Grocery Store Manager Claims That 75
May 10, 2025
-
What Does It Mean When You Dream About Piojos
May 10, 2025
Related Post
Thank you for visiting our website which covers about Quantitative Methods For Business 13th Edition . We hope the information provided has been useful to you. Feel free to contact us if you have any questions or need further assistance. See you next time and don't miss to bookmark.