Researchers Propose A Model To Explain Variation In Phytoplankton
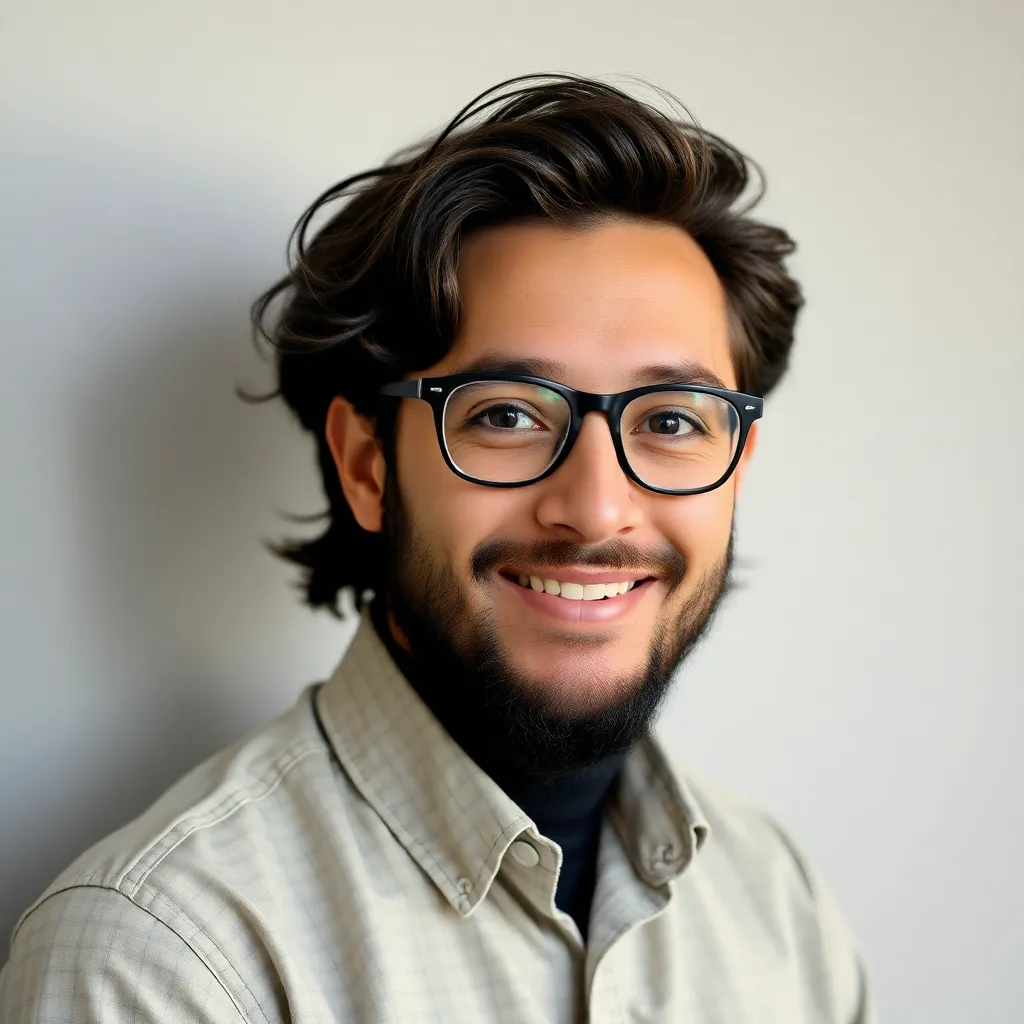
Onlines
Apr 24, 2025 · 6 min read

Table of Contents
Researchers Propose a Model to Explain Variation in Phytoplankton: Unraveling the Complexities of Ocean Life
Phytoplankton, the microscopic plants forming the base of the marine food web, exhibit remarkable variability in their abundance and distribution across the world's oceans. This variation significantly impacts marine ecosystems, global biogeochemical cycles, and even the Earth's climate. Understanding the drivers of this variability is crucial, yet it remains a complex challenge. Recently, researchers have proposed a novel model that attempts to explain this intricate variation, offering valuable insights into the dynamics of ocean life. This article delves deep into this groundbreaking research, exploring the model's core components, its implications, and future research directions.
The Intricacies of Phytoplankton Variability: A Complex Puzzle
Phytoplankton abundance and distribution are influenced by a complex interplay of factors, making it challenging to pinpoint the exact causes of observed variations. These factors can be broadly categorized into:
1. Environmental Drivers: The Physical Stage
-
Nutrient Availability: Phytoplankton, like all plants, require nutrients such as nitrates, phosphates, and silicates for growth. The availability of these nutrients, often dictated by ocean currents, upwelling events, and river runoff, directly impacts phytoplankton growth rates. Nutrient limitation is a significant factor in controlling phytoplankton blooms and distribution.
-
Light Availability: Sunlight is essential for photosynthesis, the process by which phytoplankton convert light energy into chemical energy. Light penetration depth in the ocean varies with water clarity, influencing the depth distribution of phytoplankton communities. Light limitation is particularly relevant in deeper waters or areas with high turbidity.
-
Temperature: Temperature affects the metabolic rates of phytoplankton, influencing their growth and survival. Optimal temperature ranges vary among different phytoplankton species, leading to shifts in community composition based on temperature fluctuations.
-
Grazing Pressure: Zooplankton, tiny animals that feed on phytoplankton, exert significant grazing pressure, controlling phytoplankton populations. The intensity of grazing pressure can vary based on zooplankton abundance and their feeding preferences.
2. Biological Interactions: The Living Web
-
Species Interactions: Phytoplankton communities are composed of a diverse range of species, each with unique physiological characteristics and ecological roles. Competition for resources, allelopathy (the production of chemicals that inhibit the growth of other organisms), and symbiotic relationships all influence the overall structure and dynamics of phytoplankton communities.
-
Viral Infections: Viruses play a significant role in regulating phytoplankton populations through lysis (cell bursting), impacting their abundance and distribution. Viral infection dynamics are poorly understood, but are crucial in shaping phytoplankton communities.
The Proposed Model: A Framework for Understanding Variability
The newly proposed model attempts to integrate these multiple factors into a cohesive framework to predict and explain phytoplankton variability. The core of this model rests on several key components:
1. Nutrient Dynamics: A Foundation for Growth
The model explicitly incorporates nutrient dynamics, accounting for the supply, uptake, and regeneration of essential nutrients. It considers the spatial and temporal variations in nutrient concentrations, reflecting the influence of physical processes like upwelling and mixing. Nutrient limitation thresholds are incorporated to realistically simulate phytoplankton growth under different nutrient regimes.
2. Light Limitation: The Energy Source
The model accurately simulates the effect of light availability on phytoplankton growth. It accounts for light attenuation in the water column, incorporating factors like water clarity and depth. The model's representation of light-dependent photosynthesis allows for the prediction of phytoplankton growth rates under different light conditions.
3. Temperature Sensitivity: The Metabolic Driver
The model incorporates temperature-dependent growth rates, reflecting the influence of temperature on phytoplankton metabolism. This component includes species-specific temperature responses, allowing for the simulation of shifts in community composition under varying temperature regimes. Thermal stratification and its impact on nutrient mixing are also considered.
4. Grazing Pressure: Top-Down Control
The model incorporates the effects of zooplankton grazing, acknowledging its role in controlling phytoplankton populations. The model accounts for the varying grazing pressure exerted by different zooplankton species and their interactions with phytoplankton. This element helps simulate the top-down control on phytoplankton populations.
5. Species Interactions: The Competitive Landscape
The model also addresses the complexities of interspecies interactions, including competition for resources and allelopathy. The incorporation of species-specific growth parameters and interaction coefficients enables the model to simulate the competitive dynamics within phytoplankton communities.
6. Viral Infection: A Biological Regulator
Unlike many previous models, this model incorporates the effects of viral infection on phytoplankton populations. This component accounts for the viral lysis rate and its influence on phytoplankton mortality, enhancing the realism of the model.
Model Validation and Implications
The model's validity is tested against extensive observational data from various oceanic regions. The researchers compare model predictions with observed phytoplankton biomass, species composition, and distribution patterns. The model shows a strong agreement with observed data, suggesting its ability to capture the essential processes governing phytoplankton variability.
The implications of this model are far-reaching:
-
Improved Ecosystem Predictions: The model provides a more accurate and comprehensive tool for predicting changes in marine ecosystems under varying environmental conditions. This has implications for managing fisheries, conserving marine biodiversity, and mitigating the impacts of climate change.
-
Enhanced Biogeochemical Cycle Understanding: The model helps in understanding the complex interplay between phytoplankton and global biogeochemical cycles, including the carbon cycle and nutrient cycling. This provides valuable insights for climate change modeling and prediction.
-
Targeted Conservation Strategies: The model can guide the development of targeted conservation strategies for marine ecosystems by pinpointing areas most vulnerable to environmental change or human impacts.
-
Climate Change Impacts Assessment: The model can be used to assess the potential impacts of climate change on phytoplankton communities, allowing for better predictions of the future state of marine ecosystems.
Future Research and Refinements
While this model represents a significant advancement in understanding phytoplankton variability, there is always room for improvement and refinement. Future research directions could include:
-
Increased Species Resolution: Incorporating a larger number of phytoplankton species into the model to enhance the representation of species interactions and community dynamics.
-
Improved Parameterization: Further refining the model's parameters to improve its accuracy and predictive power. This could involve incorporating more detailed physiological data and incorporating advanced statistical techniques.
-
Coupling with Other Models: Integrating the phytoplankton model with other oceanographic models (e.g., circulation models, biogeochemical models) to create a more comprehensive understanding of the coupled ocean system.
-
Incorporating Human Impacts: Explicitly incorporating the impact of human activities, such as pollution and fishing, to better predict the future of marine ecosystems under anthropogenic pressures.
-
Investigating the Role of Mixotrophy: Many phytoplankton species are mixotrophic, meaning they can both photosynthesize and consume other organisms. Incorporating the complexities of mixotrophy into the model will improve its accuracy and predictive ability.
Conclusion: A Step Towards a Holistic Understanding
The proposed model marks a significant advancement in our understanding of phytoplankton variability. By integrating multiple factors, it provides a powerful tool for predicting and explaining the dynamics of these crucial organisms. While further refinements are necessary, this model offers invaluable insights into the complex interplay between physical, chemical, and biological processes governing marine ecosystems. Its application in future research promises to significantly enhance our ability to understand and manage the world's oceans in the face of ongoing environmental change. The journey towards a truly holistic understanding of phytoplankton variability is ongoing, and this model represents a crucial step in that journey.
Latest Posts
Latest Posts
-
Which Of The Following Segments Is A Diameter Of O
Apr 24, 2025
-
Which Describes The Bass Line In The Third Section
Apr 24, 2025
-
Theme Of The Story Oliver Twist
Apr 24, 2025
-
Ribbons That Sailors Are Authorized To Wear
Apr 24, 2025
-
Ap Chemistry Unit 4 Progress Check Frq
Apr 24, 2025
Related Post
Thank you for visiting our website which covers about Researchers Propose A Model To Explain Variation In Phytoplankton . We hope the information provided has been useful to you. Feel free to contact us if you have any questions or need further assistance. See you next time and don't miss to bookmark.