Select The Category That Would Yield Quantitative Data
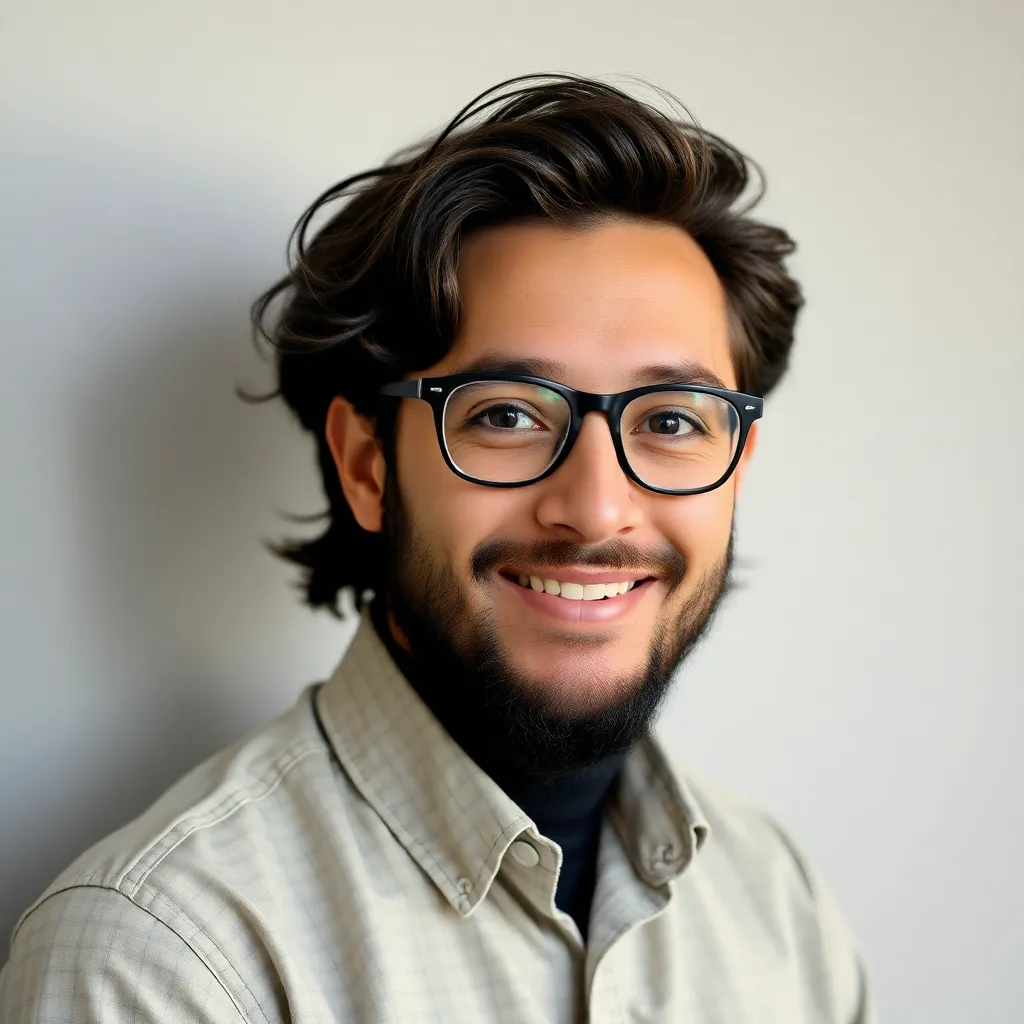
Onlines
May 07, 2025 · 6 min read
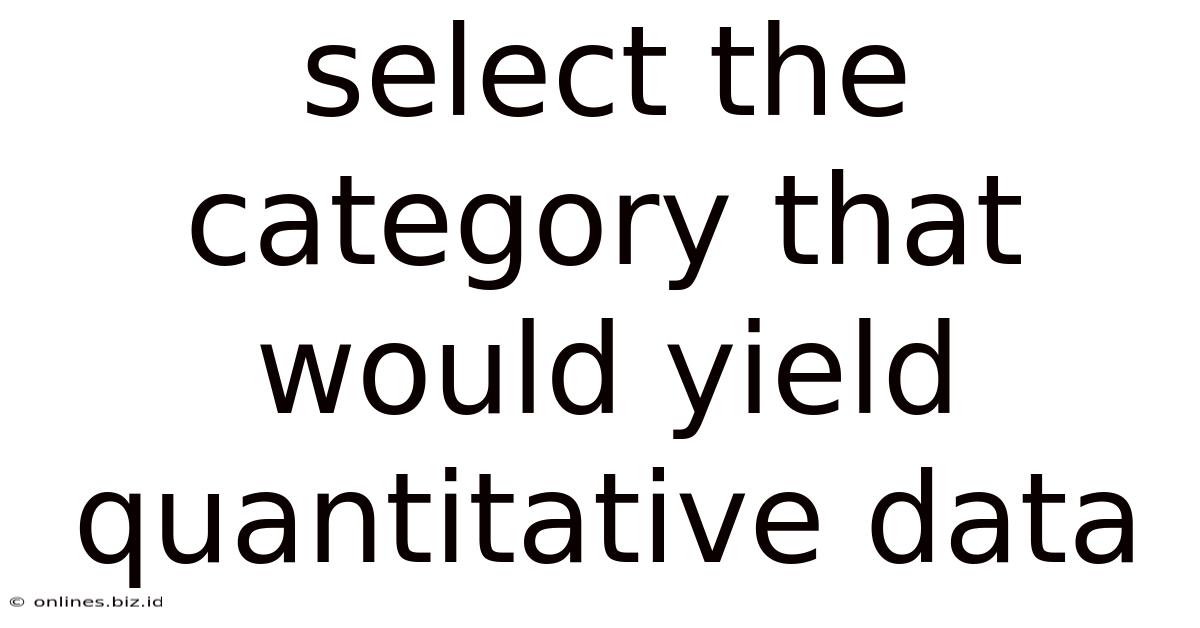
Table of Contents
Selecting Categories that Yield Quantitative Data: A Comprehensive Guide
Collecting quantitative data is crucial for many research endeavors, from scientific studies to market research and business analytics. Quantitative data, expressed numerically, allows for statistical analysis, providing valuable insights into trends, patterns, and relationships. However, selecting the right categories for data collection is paramount to ensure the data collected is both meaningful and relevant to your research objectives. This guide provides a comprehensive overview of selecting categories that will reliably yield high-quality quantitative data.
Understanding Quantitative Data and its Application
Before delving into category selection, let's solidify our understanding of quantitative data. Quantitative data is numerical data that can be measured and statistically analyzed. This contrasts with qualitative data, which is descriptive and non-numerical. Examples of quantitative data include:
- Numerical scores: Test scores, ratings on a scale (e.g., Likert scale), numerical grades.
- Measurements: Height, weight, temperature, speed, distance.
- Counts: Number of occurrences, frequency of events, population size.
- Financial data: Sales figures, income, expenses, stock prices.
Quantitative data is valuable because it allows researchers to:
- Identify trends and patterns: Analyze data to reveal recurring trends and significant patterns.
- Test hypotheses: Employ statistical tests to confirm or reject hypotheses related to your research question.
- Make predictions: Utilize statistical models to predict future outcomes based on existing data.
- Compare groups: Analyze differences and similarities between different groups or populations.
- Measure effectiveness: Evaluate the impact of interventions or programs using numerical indicators.
Key Considerations When Selecting Categories for Quantitative Data
The selection of categories for quantitative data collection is a critical step. Poorly chosen categories can lead to inaccurate, unreliable, and ultimately useless data. Here's a breakdown of key considerations:
1. Alignment with Research Objectives:
The most fundamental aspect is ensuring your categories directly relate to your research objectives. What are you trying to measure? What questions are you trying to answer? Your categories should be designed to provide the specific information needed to address these questions. For instance, if your research focuses on customer satisfaction, categories should directly assess aspects of satisfaction like product quality, customer service, and pricing.
2. Mutually Exclusive Categories:
Each data point should fit into only one category. Overlapping categories create ambiguity and make data analysis extremely difficult. For example, if you're categorizing age groups, avoid overlapping ranges like "18-25" and "25-35". Instead, use distinct ranges like "18-24" and "25-34".
3. Exhaustive Categories:
Your categories should cover all possible responses or values. Every data point should have a place to belong. If you're categorizing income levels, ensure your categories span the entire range of possible incomes, potentially including a "Other" category for unusual or extremely high values. Failing to account for all possibilities leads to incomplete data and biased results.
4. Clear and Unambiguous Definitions:
The definition of each category should be clear, concise, and unambiguous to prevent misinterpretations. Avoid vague or subjective terms. For example, instead of using "high income," define specific income brackets (e.g., >$100,000). Precise definitions guarantee consistent data collection and minimize errors.
5. Appropriate Measurement Scale:
The choice of measurement scale (nominal, ordinal, interval, ratio) influences the type of analysis you can perform and the conclusions you can draw.
- Nominal Scale: Categories are named but have no inherent order (e.g., colors, gender).
- Ordinal Scale: Categories have a rank order but the distances between ranks are not equal (e.g., education levels – high school, bachelor's, master's).
- Interval Scale: Categories have a rank order, and the intervals between ranks are equal, but there's no true zero point (e.g., temperature in Celsius).
- Ratio Scale: Categories have a rank order, equal intervals, and a true zero point (e.g., height, weight, income).
Selecting the appropriate scale ensures the data collected is suitable for the intended statistical analysis. For instance, you cannot calculate a mean for nominal data.
6. Practicality and Feasibility:
Consider the resources available for data collection. Will the categories be easy to collect and record? Will they be easy to understand for both data collectors and respondents? Avoid overly complex or time-consuming categories that may hinder data collection efforts.
7. Data Validation and Reliability:
Before implementing your data collection, pilot test your categories with a small sample to identify any issues with clarity, comprehensiveness, or practicality. This allows for refinements and ensures data accuracy and reliability.
Examples of Categories Yielding Quantitative Data
Let's illustrate with some examples across various fields:
Market Research:
- Customer Satisfaction: Use a Likert scale (1-5) to measure satisfaction with different aspects of a product or service (e.g., product quality, customer service, ease of use).
- Market Segmentation: Categorize customers based on demographics (age, gender, income, location) and psychographics (lifestyle, interests, values).
- Sales Performance: Track sales figures by product, region, sales representative, or time period.
- Website Analytics: Measure website traffic, bounce rate, conversion rate, time on site using website analytics tools.
Healthcare Research:
- Disease Prevalence: Count the number of individuals diagnosed with a specific disease within a defined population.
- Treatment Effectiveness: Measure the effectiveness of a treatment using numerical indicators such as recovery rates, survival rates, or symptom reduction scores.
- Patient Satisfaction: Employ surveys with numerical rating scales to assess patient satisfaction with healthcare services.
Educational Research:
- Student Performance: Use test scores, grades, and standardized assessment results to measure student achievement.
- Attendance Rates: Track student attendance to quantify participation in educational programs.
- Graduation Rates: Calculate the percentage of students who graduate within a specific timeframe.
Environmental Science:
- Pollution Levels: Measure air and water pollution using numerical indicators (e.g., particulate matter concentration, water pH).
- Biodiversity: Count the number of species in a given area to quantify biodiversity.
- Climate Change Indicators: Monitor temperature changes, sea levels, and greenhouse gas concentrations using numerical data.
Avoiding Common Pitfalls in Category Selection
Several pitfalls can compromise the quality of quantitative data. Let's address some common mistakes:
- Vague or subjective categories: Avoid ambiguous terms; use precise and operational definitions.
- Overlapping categories: Ensure each data point belongs to only one category.
- Non-exhaustive categories: Account for all possible responses or values.
- Inappropriate measurement scales: Choose a scale suitable for the type of data and the intended analysis.
- Leading or biased questions: Avoid questions that influence responses.
- Poorly designed survey instruments: Ensure surveys are easy to understand and complete.
- Insufficient sample size: A small sample size can lead to inaccurate generalizations.
By meticulously selecting categories and adhering to best practices, researchers can ensure high-quality, reliable quantitative data suitable for robust statistical analysis and meaningful insights. Careful consideration of research objectives, appropriate measurement scales, and clear definitions will ultimately contribute to accurate findings and impactful research conclusions. Remember, the foundation of a successful quantitative study lies in the meticulous planning and thoughtful selection of data categories.
Latest Posts
Latest Posts
-
The Value Of Information Comes From The Characteristics It Possesses
May 08, 2025
-
Functional Anatomy Of The Digestive System Exercise 25
May 08, 2025
-
Effective Performance Appraisals Are Based On
May 08, 2025
-
Which Of The Following Is Considered Objective Information
May 08, 2025
-
Production Units Have An Optimal Rate Of Output Where
May 08, 2025
Related Post
Thank you for visiting our website which covers about Select The Category That Would Yield Quantitative Data . We hope the information provided has been useful to you. Feel free to contact us if you have any questions or need further assistance. See you next time and don't miss to bookmark.