Select The False Statement About Anova
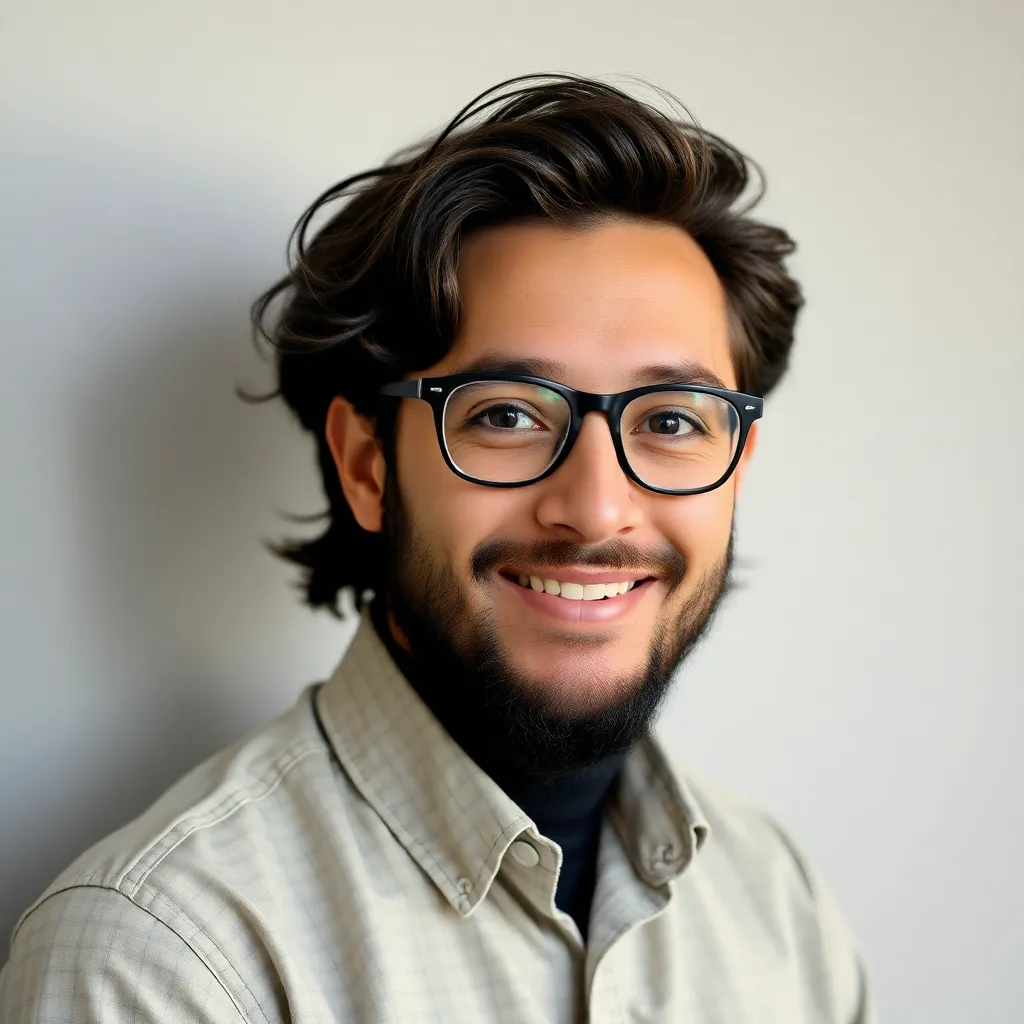
Onlines
May 03, 2025 · 6 min read
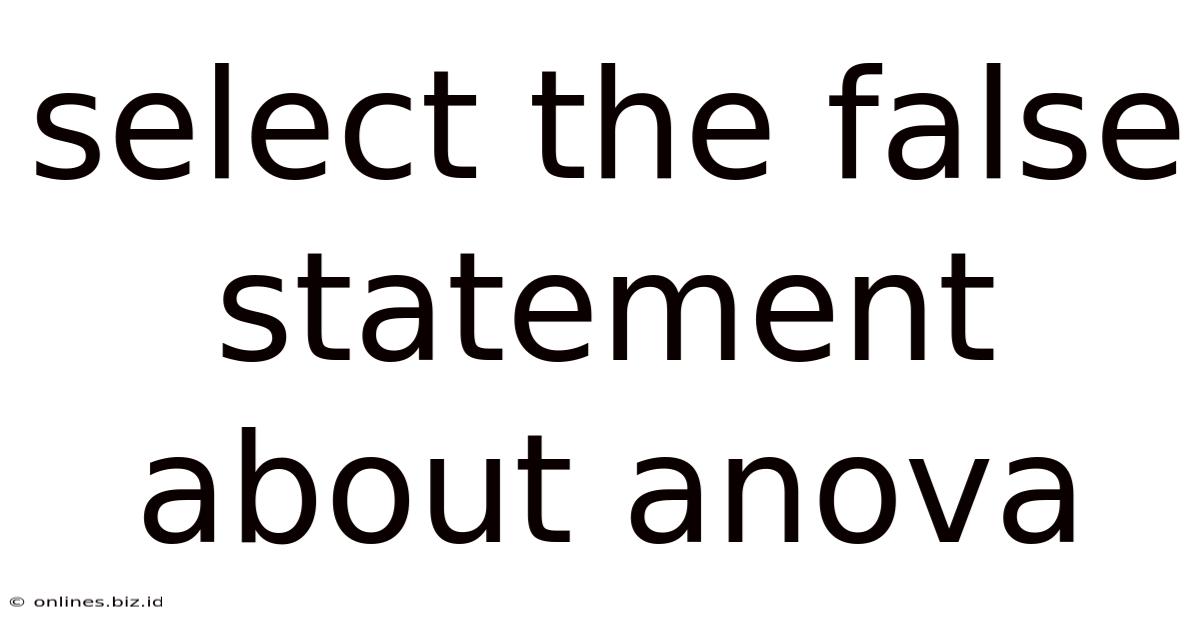
Table of Contents
Selecting the False Statement About ANOVA: A Deep Dive into Analysis of Variance
Analysis of Variance (ANOVA) is a powerful statistical tool used to compare the means of two or more groups. Understanding its nuances is crucial for accurate data interpretation and drawing valid conclusions. This article delves into common misconceptions surrounding ANOVA, ultimately helping you select the false statement from a given set. We will explore the underlying assumptions, different types of ANOVA, and potential pitfalls to avoid.
Understanding ANOVA Fundamentals
Before identifying false statements, let's solidify our understanding of ANOVA. ANOVA tests the null hypothesis that the means of all groups are equal. If the test rejects the null hypothesis, it suggests that at least one group mean is significantly different from the others. The strength of the evidence against the null hypothesis is measured by the F-statistic, which is the ratio of the between-group variance to the within-group variance.
Types of ANOVA
Several types of ANOVA exist, each suited to different experimental designs:
-
One-way ANOVA: Compares the means of two or more independent groups based on a single independent variable (factor). For example, comparing the average test scores of students from three different teaching methods.
-
Two-way ANOVA: Analyzes the means of groups based on two or more independent variables (factors) and their interactions. For instance, comparing crop yields based on different fertilizers and watering techniques.
-
Repeated Measures ANOVA: Used when the same subjects are measured multiple times under different conditions. This is common in longitudinal studies or experiments involving repeated treatments.
-
MANOVA (Multivariate ANOVA): Extends ANOVA to analyze multiple dependent variables simultaneously. This is useful when you have several outcome measures related to the same experimental question.
Common Misconceptions and False Statements about ANOVA
Many misconceptions surround ANOVA. Let's examine some common false statements, clarifying why they are incorrect.
False Statement 1: ANOVA assumes that the variances of all groups are equal.
Correct Statement: While ANOVA is relatively robust to violations of this assumption (homogeneity of variances), it is ideally assumed. The assumption is that the variances of the populations from which the samples are drawn are equal. Severe violations can affect the accuracy of the Type I error rate (false positives). Tests like Levene's test are used to assess the equality of variances. If the assumption is violated, transformations of the data or alternative non-parametric tests (like the Kruskal-Wallis test) might be necessary.
False Statement 2: ANOVA can only be used with normally distributed data.
Correct Statement: ANOVA is robust to moderate departures from normality, especially with larger sample sizes. The central limit theorem suggests that the sampling distribution of the means will approach normality, even if the underlying data is not perfectly normal. However, severe deviations from normality, particularly with small sample sizes, can compromise the accuracy of the results. In such cases, non-parametric alternatives are preferred.
False Statement 3: A significant F-statistic always indicates which specific group means differ significantly.
Correct Statement: A significant F-statistic only indicates that there is a statistically significant difference between at least two group means. It doesn't pinpoint which specific groups differ. To determine which specific groups differ, post-hoc tests (like Tukey's HSD, Bonferroni correction, or Scheffe's method) are necessary. These tests perform multiple comparisons while controlling the family-wise error rate (the probability of making at least one Type I error across all comparisons).
False Statement 4: ANOVA is only suitable for comparing the means of three or more groups.
Correct Statement: While ANOVA is often associated with comparing three or more groups, it can be used to compare two group means. However, in this case, it's statistically equivalent to a t-test. Using ANOVA for a two-group comparison is not wrong, but it's less efficient than a direct t-test.
False Statement 5: A non-significant F-statistic proves that all group means are equal.
Correct Statement: A non-significant F-statistic indicates that there is not enough evidence to reject the null hypothesis. It does not mean that the null hypothesis (all group means are equal) is true. It simply means that the observed differences between group means could be due to chance alone. There might still be true differences, but the sample size or the variability within groups might be insufficient to detect them. This highlights the importance of considering effect size along with statistical significance.
False Statement 6: ANOVA assumes independence of observations only within each group.
Correct Statement: ANOVA assumes independence of observations both within and between groups. The observations within each group should be independent of each other, and the observations in one group should be independent of observations in other groups. Violation of this assumption, such as in clustered data or repeated measures without appropriate adjustments, can lead to inflated Type I error rates.
False Statement 7: ANOVA automatically handles unequal sample sizes across groups.
Correct Statement: While ANOVA can handle unequal sample sizes, its power and the accuracy of its assumptions are most reliable when sample sizes are relatively balanced across groups. Unequal sample sizes, especially when the imbalance is substantial, can lead to a decrease in statistical power and potential biases in the results. Careful consideration of sample size balance is important during the experimental design phase.
False Statement 8: The assumptions of ANOVA are always critical and must be perfectly met.
Correct Statement: While the assumptions of ANOVA are important, the test is relatively robust to minor violations, especially with larger sample sizes. The severity of the impact of violating an assumption depends on the extent of the violation and the sample size. The consequences might be a slight increase in the Type I or Type II error rates. However, it's crucial to assess the assumptions and consider alternatives if the violations are substantial.
False Statement 9: ANOVA is the only statistical test that can compare means across multiple groups.
Correct Statement: While ANOVA is a common and powerful tool for comparing means, several other statistical methods can also be used, particularly when assumptions are violated. Non-parametric alternatives, such as the Kruskal-Wallis test (analogous to one-way ANOVA), can be employed when normality or homogeneity of variances are not met.
False Statement 10: Post-hoc tests are always necessary after a significant ANOVA result.
Correct Statement: Post-hoc tests are necessary only when the overall ANOVA test is significant. If the ANOVA result is non-significant, there is no need to perform post-hoc tests because the null hypothesis is not rejected. Performing post-hoc tests after a non-significant ANOVA is inappropriate and can lead to spurious conclusions.
Conclusion
Understanding the assumptions, strengths, and limitations of ANOVA is crucial for accurate data analysis. This article has highlighted ten common misconceptions, providing a clearer understanding of how to select the false statement from a given set. Remember, the choice of statistical test depends on the specific research question, experimental design, and characteristics of the data. Always assess the assumptions before making conclusions, and consider alternative methods when assumptions are violated or the data structure doesn't align with ANOVA's requirements. Careful planning, appropriate statistical analysis, and a nuanced understanding of ANOVA are essential for drawing valid and reliable conclusions from your research.
Latest Posts
Latest Posts
-
What Are The Valid Indexes For The String New York
May 07, 2025
-
Hideki Tells A Lie And Is Grounded
May 07, 2025
-
Progressive Po Box 31260 Tampa Fl Zip Code
May 07, 2025
-
Using Heat Reclaim Coils Will Help Improve A Buildings Overall
May 07, 2025
-
U Turns Are Never Permitted On
May 07, 2025
Related Post
Thank you for visiting our website which covers about Select The False Statement About Anova . We hope the information provided has been useful to you. Feel free to contact us if you have any questions or need further assistance. See you next time and don't miss to bookmark.