Select The Four Primary Traits Of The Value Of Data.
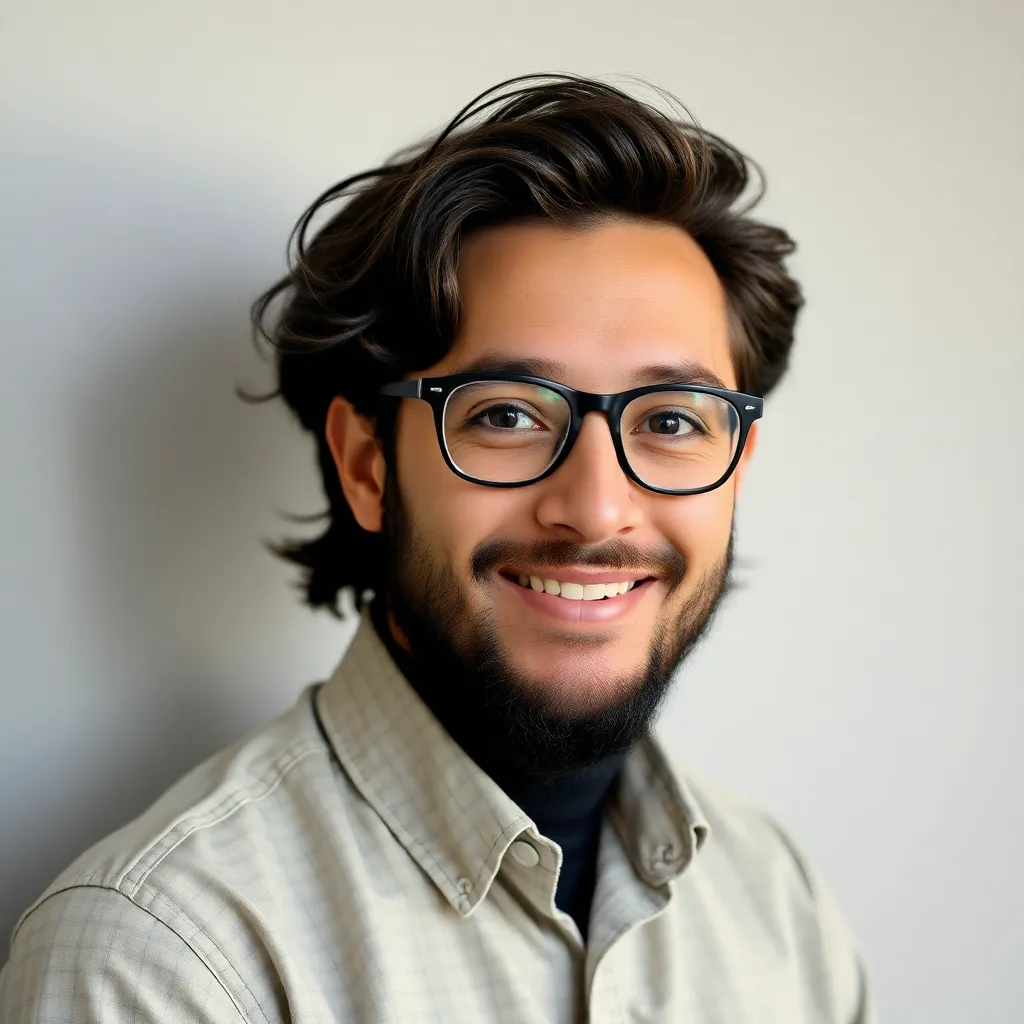
Onlines
May 12, 2025 · 6 min read
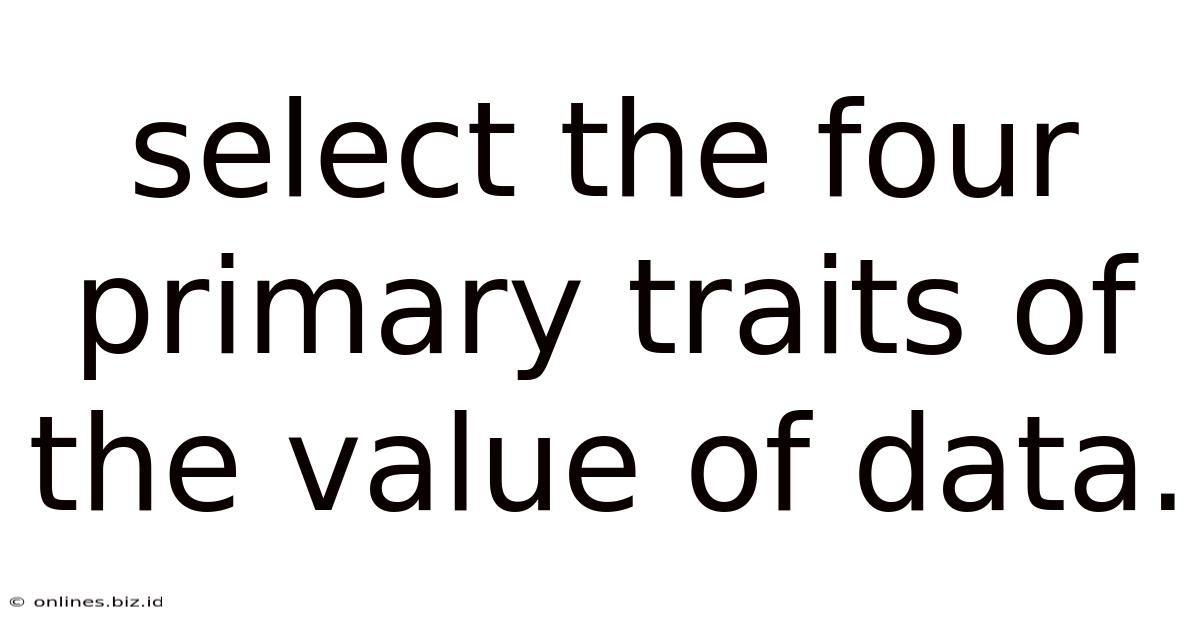
Table of Contents
Selecting the Four Primary Traits of Data Value
Data has become the lifeblood of the modern world. From informing business decisions to driving scientific breakthroughs, its importance is undeniable. But what truly makes data valuable? It's not simply the sheer volume, but rather a confluence of intrinsic characteristics that determine its worth. While numerous facets contribute to data's value, we can distill them down to four primary traits: accuracy, completeness, timeliness, and relevance. Understanding these traits is crucial for effectively leveraging data and maximizing its potential.
1. Accuracy: The Foundation of Trust
Accuracy is arguably the most fundamental trait of valuable data. Inaccurate data is not just useless; it's actively harmful. It can lead to flawed analyses, incorrect conclusions, and ultimately, poor decision-making. Imagine relying on sales figures that are off by 10% – the consequences could be significant, ranging from missed opportunities to substantial financial losses.
Ensuring Data Accuracy: A Multifaceted Approach
Achieving data accuracy requires a multifaceted approach, beginning at the point of data collection. This involves:
- Robust data validation techniques: Implementing checks and balances at every stage of the data pipeline, from input to storage, is critical. This includes data type validation, range checks, and consistency checks to ensure that the data conforms to predefined standards.
- Data cleaning and scrubbing: Real-world data is rarely perfect. Cleaning and scrubbing processes are essential to identify and correct errors, inconsistencies, and outliers. This can involve techniques like deduplication, standardization, and imputation.
- Regular audits and quality checks: Periodic audits and quality checks are crucial for maintaining data accuracy over time. These checks should cover all aspects of the data lifecycle, from source to application.
- Investing in data quality tools and technologies: Employing specialized data quality tools can automate many of these processes, reducing manual effort and improving efficiency. These tools often incorporate sophisticated algorithms for detecting and correcting errors.
The cost of inaccurate data far outweighs the investment in ensuring its accuracy. The damage caused by flawed decisions based on unreliable information can be devastating, affecting everything from financial performance to public health.
2. Completeness: The Pursuit of the Whole Picture
Completeness refers to the extent to which the data set contains all the necessary information. Incomplete data hampers analysis and can lead to biased or incomplete conclusions. For instance, a customer survey with a high number of missing responses may not accurately reflect customer sentiment. Similarly, incomplete financial data can make it impossible to accurately assess the financial health of a company.
Strategies for Achieving Data Completeness
Ensuring data completeness demands a proactive approach that addresses potential gaps throughout the data lifecycle. Key strategies include:
- Comprehensive data collection processes: Designing data collection methods that capture all relevant information is paramount. This includes identifying all key variables and developing effective mechanisms for collecting data from various sources.
- Data integration techniques: Often, data resides in disparate systems and formats. Effective data integration techniques are essential for combining data from multiple sources into a unified and complete view.
- Data imputation methods: When data is missing, imputation methods can be used to estimate missing values based on existing data. However, it's crucial to use appropriate imputation techniques to avoid introducing bias.
- Data governance policies: Establishing clear data governance policies can help ensure that data is collected and maintained consistently across all systems and departments.
The absence of crucial information can significantly distort the picture, rendering the analysis meaningless. Strive for completeness to gain a holistic understanding and make informed decisions.
3. Timeliness: The Importance of Real-Time Insights
Timeliness refers to the speed at which data is available and its relevance to the current situation. Outdated data is often useless for decision-making. In today's fast-paced business environment, real-time or near real-time data is often crucial for competitive advantage.
Maximizing the Value of Timely Data
The value of data diminishes rapidly over time. Therefore, strategies for ensuring timeliness are critical:
- Real-time data pipelines: Establishing efficient data pipelines that deliver data quickly and reliably is paramount. This may involve utilizing technologies such as stream processing and real-time databases.
- Automated data ingestion and processing: Automating the data ingestion and processing workflow can significantly reduce latency and ensure that data is available promptly.
- Data warehousing and data lakes: Effective data warehousing and data lake architectures are essential for storing and accessing large volumes of data quickly and efficiently.
- Data visualization and reporting tools: Powerful data visualization and reporting tools allow for rapid analysis and interpretation of data, enabling timely insights.
Timely data empowers businesses to react swiftly to changing market conditions, identify emerging trends, and make proactive decisions. Delay in access can lead to missed opportunities and compromised decision-making.
4. Relevance: Focusing on the Essential Information
Relevance refers to the extent to which the data is applicable to the specific problem or question being addressed. Collecting irrelevant data is a waste of resources. Focusing on relevant data improves the efficiency of analysis and decision-making.
Strategies for Selecting Relevant Data
Determining what data is relevant requires careful consideration of the specific context and goals. Key steps include:
- Clearly defined objectives: Begin with clearly defined objectives and goals. This will help to identify the specific information needed to achieve those goals.
- Data profiling and analysis: Before collecting data, conduct thorough data profiling and analysis to understand the available data and its potential relevance to your objectives.
- Data filtering and selection: Use data filtering and selection techniques to isolate the relevant data from the irrelevant data.
- Data transformation and aggregation: Transform and aggregate data to create meaningful summaries and insights relevant to the decision-making process.
Irrelevant data clutters the picture, obscuring important insights. Focusing on relevant data streamlines analysis and ensures that resources are used effectively.
Conclusion: The Interplay of Data Traits
While we've discussed these four traits – accuracy, completeness, timeliness, and relevance – separately, it's important to understand that they are interconnected. For example, incomplete data can compromise accuracy, and outdated data is often less relevant. A successful data strategy requires a holistic approach that addresses all four traits simultaneously. By prioritizing these characteristics, organizations can unlock the true potential of their data and gain a significant competitive advantage in today's data-driven world. This ensures that data is not just collected, but effectively utilized to drive strategic decision-making, improve operational efficiency, and foster innovation. The pursuit of these four primary traits is an ongoing process, requiring constant monitoring, refinement, and adaptation to evolving business needs and technological advancements. Continuous improvement in data quality and management ensures the long-term value and impactful contribution of data to an organization's success.
Latest Posts
Related Post
Thank you for visiting our website which covers about Select The Four Primary Traits Of The Value Of Data. . We hope the information provided has been useful to you. Feel free to contact us if you have any questions or need further assistance. See you next time and don't miss to bookmark.