Select The True Statement For Completely Random Design
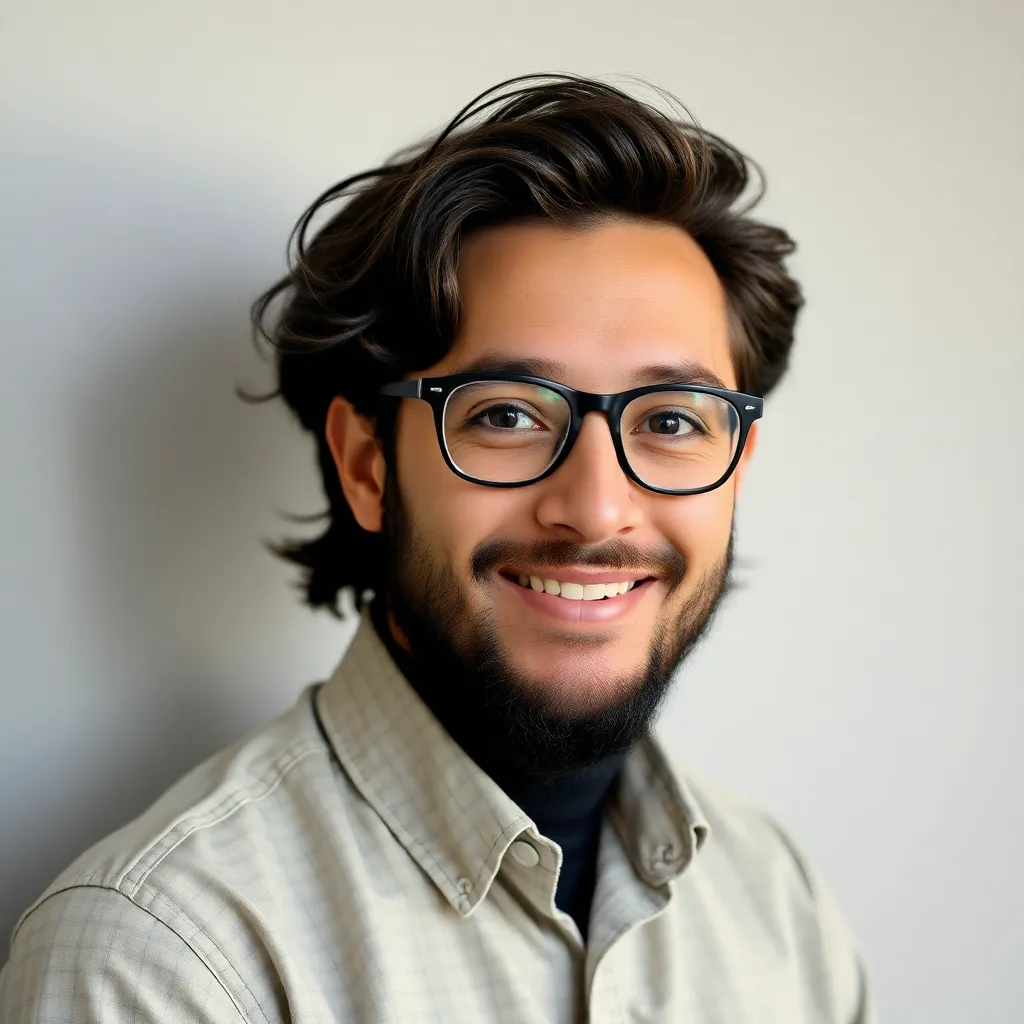
Onlines
May 10, 2025 · 6 min read
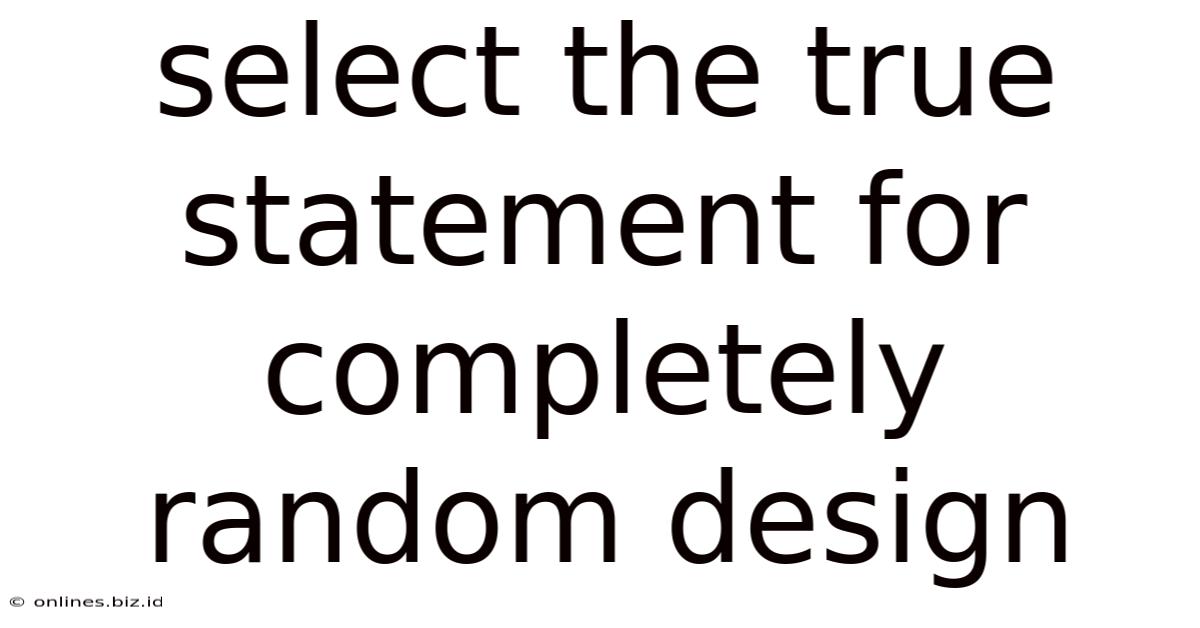
Table of Contents
Selecting the True Statement for Completely Random Design: A Deep Dive into Randomness and its Applications
Completely random design, in the context of experimentation and data analysis, refers to a design where treatments are assigned to experimental units entirely by chance. This seemingly simple concept has profound implications across various fields, from clinical trials and agricultural research to software testing and even artistic creation. Understanding the nuances of completely random design and its underlying principles is crucial for drawing valid conclusions and making informed decisions. This article delves into the intricacies of completely random design, explores its advantages and limitations, and clarifies common misconceptions. We'll also examine how to select the true statement regarding completely random design from a set of options, equipping you with the knowledge to confidently analyze and interpret experimental results.
What is Completely Random Design?
A completely random design (CRD) is a fundamental experimental design where experimental units are randomly assigned to different treatment groups. This randomness is paramount, as it ensures that any observed differences between the groups are likely due to the treatments themselves and not to pre-existing variations among the units. The absence of any systematic bias in treatment allocation is the defining characteristic of a CRD.
Key Characteristics of a CRD:
- Random Assignment: Treatments are assigned to experimental units purely by chance. This can be achieved using various randomization techniques, such as random number generators or lottery methods.
- Independent Observations: The outcome of one experimental unit does not influence the outcome of another. This independence is a crucial assumption for many statistical analyses.
- Homogeneity of Variance: Ideally, the variability within each treatment group should be roughly equal. While not a strict requirement, significant differences in variance can affect the validity of certain statistical tests.
- No Blocking: Unlike more complex designs like randomized block designs, CRDs do not involve grouping units into blocks based on any pre-existing characteristics.
When is a CRD Appropriate?
A CRD is often the simplest and most straightforward experimental design. It's particularly suitable when:
- The experimental units are homogeneous: If there's minimal variability among the units, a CRD can be effective.
- The number of treatments is relatively small: CRD becomes less efficient as the number of treatments increases.
- Resources are limited: CRDs are less demanding in terms of resources and experimental setup compared to more sophisticated designs.
- The primary goal is to compare treatment means: CRDs are well-suited for determining whether there are significant differences in the average response between treatment groups.
Advantages of Completely Random Design
- Simplicity: CRD is easy to understand, implement, and analyze.
- Flexibility: It can be applied across diverse fields and experimental settings.
- Unbiased Estimates: Random assignment minimizes bias, leading to more reliable estimates of treatment effects.
- Statistical Inference: Standard statistical tests can be readily applied to analyze data from a CRD.
Limitations of Completely Random Design
Despite its simplicity and advantages, CRDs also have limitations:
- Inefficiency with Heterogeneous Units: If experimental units are highly heterogeneous (show substantial variability), a CRD can be inefficient and lead to imprecise estimates of treatment effects. The variation among units might mask the true treatment effects.
- Increased Error Variance: In the presence of substantial heterogeneity, the error variance (the variability within treatment groups) increases, reducing the power of the statistical tests to detect significant differences.
- Limited Applicability with Large Numbers of Treatments: As the number of treatments increases, the number of experimental units required for a CRD can become excessively large, making it impractical.
Selecting the True Statement: Example Scenarios
Let's consider some example statements about completely random design and determine which are true.
Scenario 1:
- Statement A: In a completely random design, treatments are assigned to experimental units systematically to minimize bias.
- Statement B: In a completely random design, treatments are assigned to experimental units randomly to minimize bias.
- Statement C: A completely random design requires blocking of experimental units.
- Statement D: A completely random design assumes that there are no differences between experimental units.
The correct answer is B. Statement A is incorrect because systematic assignment introduces bias. Statement C is incorrect because blocking is a characteristic of other designs, not CRD. Statement D is incorrect; while homogeneity is desirable, CRDs don't assume perfect uniformity among units. The strength of CRD lies in its ability to handle some heterogeneity through random allocation.
Scenario 2:
- Statement A: A completely random design is always the most efficient experimental design.
- Statement B: A completely random design is suitable for experiments with a large number of treatments and heterogeneous experimental units.
- Statement C: Randomization in a completely random design ensures that any observed differences between treatment groups are likely due to the treatments themselves.
- Statement D: Analysis of data from a completely random design requires advanced statistical techniques.
The correct answer is C. Statement A is false because efficiency depends on the characteristics of the experimental units and the number of treatments. Statement B is false because CRDs are less efficient with many treatments and heterogeneous units. Statement D is false because standard statistical tests (like ANOVA) are generally suitable.
Scenario 3:
- Statement A: Completely random designs are only applicable to agricultural experiments.
- Statement B: Completely random designs are prone to confounding variables.
- Statement C: Proper randomization in a completely random design helps control for confounding variables.
- Statement D: The assumption of independence is crucial in analyzing data from a completely random design.
The correct answer is C & D. Statement A is false because CRDs are used across numerous fields. Statement B is partially true; confounding is a risk in any design, but proper randomization minimizes it in CRD. Statement C is true because randomization helps to distribute any confounding factors evenly across treatment groups. Statement D is true because the independence assumption underlies many statistical analyses used with CRDs.
Misconceptions about Completely Random Design
Several misconceptions surround completely random design. It's crucial to clarify these to ensure a clear understanding:
- Misconception 1: CRD requires perfectly homogeneous units. Reality: While homogeneity is desirable, CRD can handle some heterogeneity through random assignment.
- Misconception 2: CRD is always the best choice. Reality: The suitability of CRD depends on the specifics of the experiment. Other designs might be more efficient in certain situations.
- Misconception 3: Random assignment guarantees identical results. Reality: Random assignment minimizes bias, but it doesn't guarantee identical results due to inherent variability among units and random error.
- Misconception 4: CRD is only useful for simple experiments. Reality: CRDs can be used for complex experiments, although other designs may be more suitable for greater efficiency.
Conclusion
Completely random design is a cornerstone of experimental design. Understanding its principles, advantages, and limitations is vital for conducting valid and reliable experiments. By carefully considering the characteristics of your experimental units and the nature of your research question, you can determine whether a CRD is appropriate and avoid common pitfalls. Remember, the key to a successful CRD lies in the meticulous and unbiased execution of random treatment assignment. This, combined with appropriate statistical analysis, allows for robust conclusions and informed decision-making. Selecting the true statement regarding a completely random design requires a solid grasp of these core principles and the ability to critically evaluate different experimental scenarios.
Latest Posts
Latest Posts
-
Acid And Base Reactions Worksheet Answers
May 10, 2025
-
In Persuasive Requests The Reasons And Explanations Usually The Request
May 10, 2025
-
Why Would Dental Units Have More Bacteria Than A Faucet
May 10, 2025
-
Marlowe Wakes Up From A Dream
May 10, 2025
-
Which Statement Is An Objective Summary Of The Passage
May 10, 2025
Related Post
Thank you for visiting our website which covers about Select The True Statement For Completely Random Design . We hope the information provided has been useful to you. Feel free to contact us if you have any questions or need further assistance. See you next time and don't miss to bookmark.