Select The True Statements About Ai Language Models.
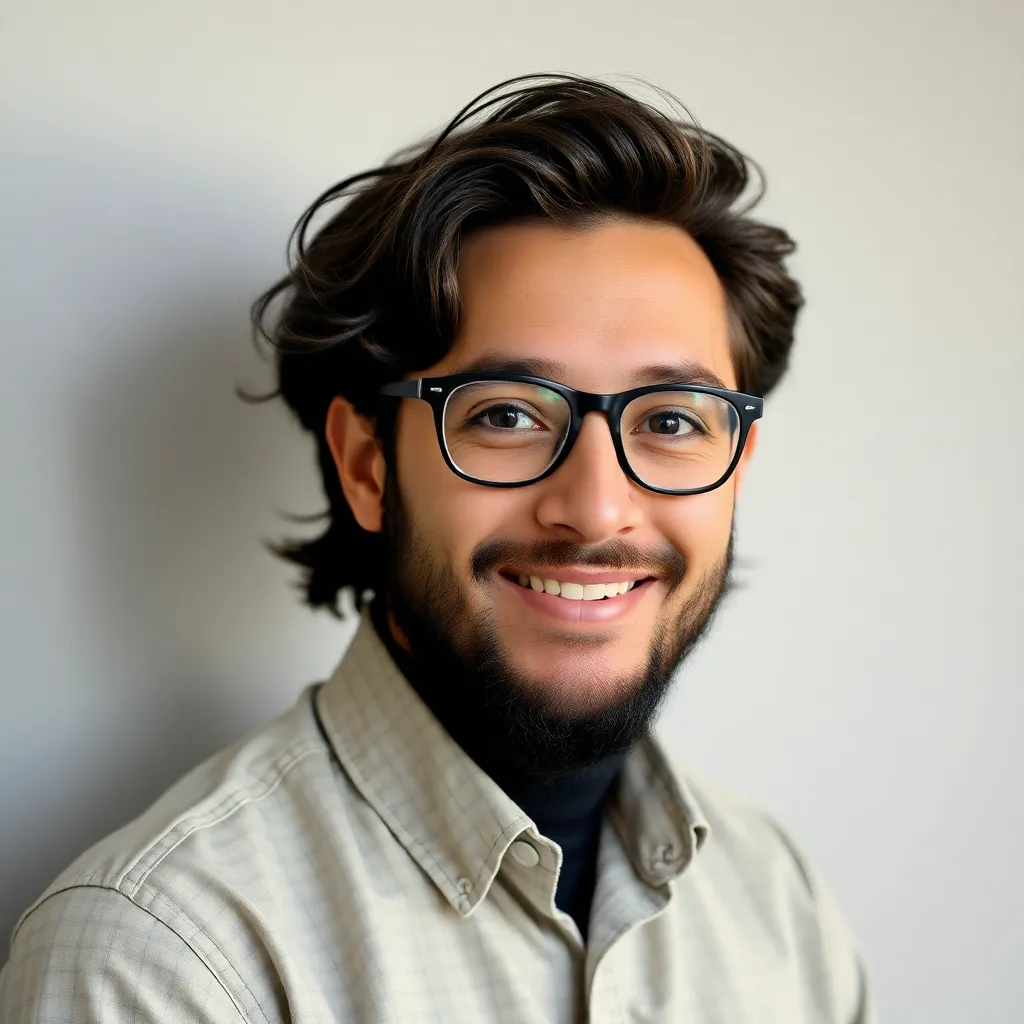
Onlines
May 08, 2025 · 8 min read
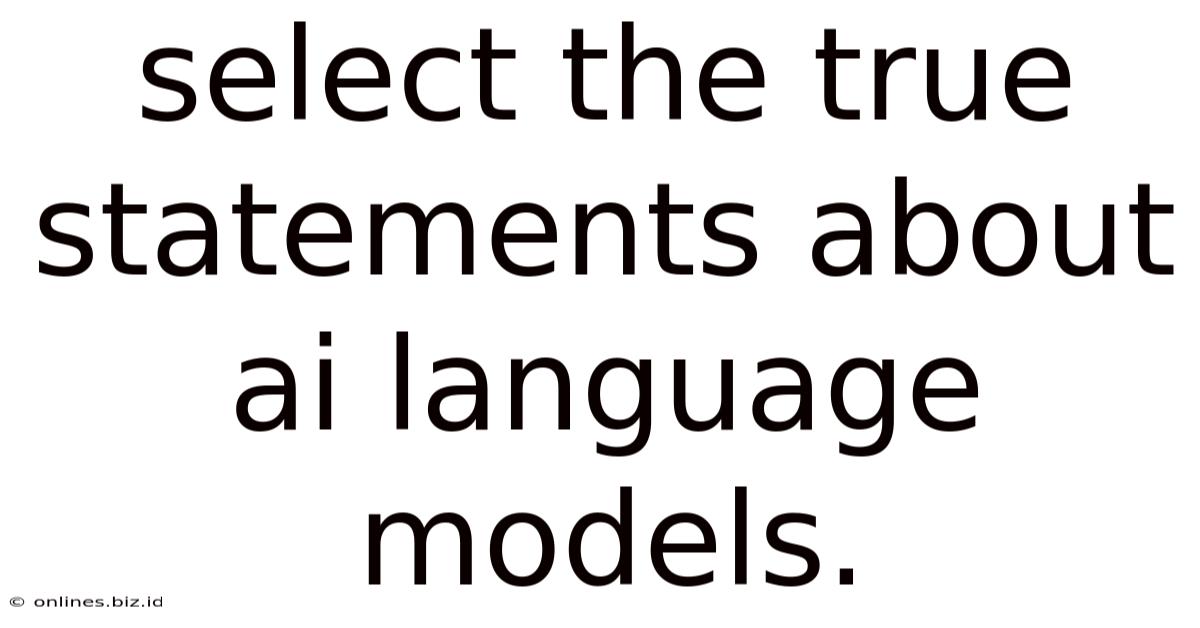
Table of Contents
Selecting the True Statements About AI Language Models: A Deep Dive
Artificial intelligence (AI) language models have rapidly evolved, transforming how we interact with technology and each other. From powering chatbots and virtual assistants to generating creative text formats, their capabilities are constantly expanding. Understanding these models, however, requires navigating a landscape of both hype and genuine innovation. This article aims to dissect several statements about AI language models, identifying the true ones and exploring the nuances behind their functionality and limitations.
Understanding the Fundamentals: What Are AI Language Models?
Before diving into specific statements, let's establish a common understanding. AI language models are sophisticated algorithms trained on massive datasets of text and code. This training allows them to learn patterns, relationships, and the statistical probabilities of word sequences. This isn't about understanding language in the human sense; instead, these models predict the most likely next word in a sequence based on the preceding words and the data they've been trained on.
This predictive capability underlies their many applications. They can:
- Generate text: Write stories, poems, articles, code, and more.
- Translate languages: Convert text from one language to another.
- Answer questions: Provide information based on their training data.
- Summarize text: Condense lengthy documents into shorter summaries.
- Complete sentences and paragraphs: Predict and generate text to complete partially written input.
However, it's crucial to recognize their limitations. They don't "think" or "understand" in the way humans do. Their output is based on statistical probabilities, not genuine comprehension. This leads to potential inaccuracies, biases, and the generation of nonsensical or inappropriate content.
Evaluating Statements About AI Language Models
Now, let's analyze several statements about AI language models, determining their veracity:
Statement 1: AI language models are trained on massive datasets of text and code.
True. This is a fundamental aspect of how these models function. The larger and more diverse the dataset, the better the model's ability to generate coherent and relevant text. These datasets often include books, articles, websites, code repositories, and other sources of textual data. The sheer scale of these datasets is a key factor contributing to their impressive capabilities. The process involves feeding the data to the model, allowing it to learn statistical patterns and relationships between words and phrases. This process is computationally intensive and requires significant resources. The quality and diversity of the data significantly impact the model's performance. Biased or incomplete datasets can lead to biased or inaccurate outputs.
Statement 2: AI language models understand the meaning of the text they generate.
False. This is a crucial distinction to make. While AI language models can generate grammatically correct and contextually relevant text, they do not genuinely understand the meaning of the words or sentences they produce. Their output is based on statistical probabilities derived from the training data. They identify patterns and relationships between words but lack the cognitive abilities to comprehend meaning in the human sense. This limitation is significant, leading to potential errors and misinterpretations, particularly when dealing with complex or nuanced topics. A model might produce grammatically perfect sentences that are semantically nonsensical, highlighting this critical difference between pattern recognition and true understanding.
Statement 3: Larger models consistently outperform smaller models.
Mostly True, but with Nuances. Generally, larger models with more parameters (the variables that govern the model's behavior) tend to achieve better performance on a range of tasks. This is because larger models can capture more complex patterns and relationships in the data. However, simply increasing the size of a model isn't a guarantee of success. Other factors, such as the quality of the training data, the model architecture, and the training process, also play a significant role. A smaller, well-designed model trained on high-quality data can sometimes outperform a larger, poorly trained model. Furthermore, extremely large models can be computationally expensive and challenging to train and deploy, presenting practical limitations. The optimal model size depends on the specific task and the available resources.
Statement 4: AI language models can be used to create realistic fake news articles.
True. This is a serious ethical concern associated with AI language models. Their ability to generate grammatically correct and contextually relevant text makes them a powerful tool for creating convincing but false information. The potential for misuse in spreading misinformation and propaganda is significant. This capability highlights the need for careful consideration of the ethical implications of these technologies and the development of strategies to detect and mitigate the spread of AI-generated fake news. The ease with which realistic text can be generated underscores the importance of media literacy and critical thinking skills in navigating the digital landscape.
Statement 5: AI language models are completely unbiased.
False. AI language models inherit the biases present in the data they are trained on. If the training data reflects societal biases related to gender, race, religion, or other factors, the model will likely perpetuate these biases in its output. This is a significant limitation, highlighting the need for careful curation of training data and the development of methods to mitigate biases in these models. The challenge lies in identifying and correcting these biases without compromising the model's performance. Ongoing research focuses on developing techniques to detect and mitigate biases, but it remains an active area of development.
Statement 6: AI language models can learn and adapt without retraining.
Partially True (through techniques like fine-tuning). While AI language models are not designed to learn and adapt in the same way as humans, they can be adapted to specific tasks through a process called fine-tuning. This involves training the model on a smaller, more targeted dataset related to the specific task. This fine-tuning allows the model to adapt its behavior and improve its performance on that particular task without requiring a complete retraining from scratch. However, this process is not truly "learning" in the same way humans do. It's a form of adaptation based on adjusting existing parameters within the model's architecture. The model's underlying architecture and initial training remain unchanged. True continuous learning and adaptation, similar to human learning, is a significant challenge for AI language models.
Statement 7: AI language models can be used for creative writing tasks.
True. AI language models are proving to be powerful tools for creative writing, assisting writers in generating ideas, overcoming writer's block, and exploring different writing styles. While they cannot replace human creativity, they can serve as valuable aids in the writing process, offering suggestions, generating different variations of text, and assisting with tasks like outlining and drafting. However, it's crucial to remember that the output still requires human editing and refinement to achieve a polished and meaningful piece of writing. The human element remains crucial in bringing creativity, emotion, and nuanced understanding to the final product.
Statement 8: The output of an AI language model is always accurate.
False. It is crucial to emphasize that the output of an AI language model is not always accurate. The models rely on statistical probabilities, and they can generate incorrect or misleading information. Critical thinking and fact-checking are necessary when using the output of these models, especially for tasks requiring accuracy and reliability. This limitation emphasizes the need for responsible use of these technologies and the importance of human oversight in verifying the accuracy of the generated content. Blindly trusting the output of an AI language model can lead to significant errors and misinterpretations.
Statement 9: AI language models are only useful for generating text.
False. While text generation is a prominent application, AI language models have a much broader range of applications. They are used in machine translation, question answering, text summarization, code generation, and various other natural language processing tasks. Their versatility and adaptability make them valuable tools in diverse fields, such as healthcare, finance, education, and research. The potential applications are constantly expanding as researchers and developers explore new ways to leverage their capabilities.
Statement 10: Developing and deploying AI language models is inexpensive.
False. Developing and deploying large-scale AI language models requires significant computational resources, specialized expertise, and considerable financial investment. The training process alone can consume vast amounts of energy and time. Furthermore, maintaining and updating these models requires ongoing investment. The high cost of development and deployment is a significant barrier to entry for many organizations and individuals. This emphasizes that while the technology is widely accessible through APIs, the development of the underlying models is a computationally intensive and costly endeavor.
Conclusion: Navigating the Future of AI Language Models
AI language models represent a significant advancement in artificial intelligence. Their ability to generate human-quality text has numerous applications across various fields. However, it is essential to understand their limitations and potential biases. The statements analyzed above highlight the need for critical evaluation and responsible use of this powerful technology. As these models continue to evolve, addressing ethical concerns and fostering responsible innovation will be crucial in harnessing their potential while mitigating their risks. The future of AI language models hinges on a careful balance between technological advancement and ethical considerations.
Latest Posts
Latest Posts
-
Ap Bio Unit 6 Progress Check Frq
May 09, 2025
-
Why Is Modeling Optimism Helpful To Families
May 09, 2025
-
House On Mango Street One Pager
May 09, 2025
-
Summary Of To Kill A Mockingbird Chapter 29
May 09, 2025
-
Algeria My Father Writes To My Mother
May 09, 2025
Related Post
Thank you for visiting our website which covers about Select The True Statements About Ai Language Models. . We hope the information provided has been useful to you. Feel free to contact us if you have any questions or need further assistance. See you next time and don't miss to bookmark.