Select The True Statements About The Process Of Learning Ai.
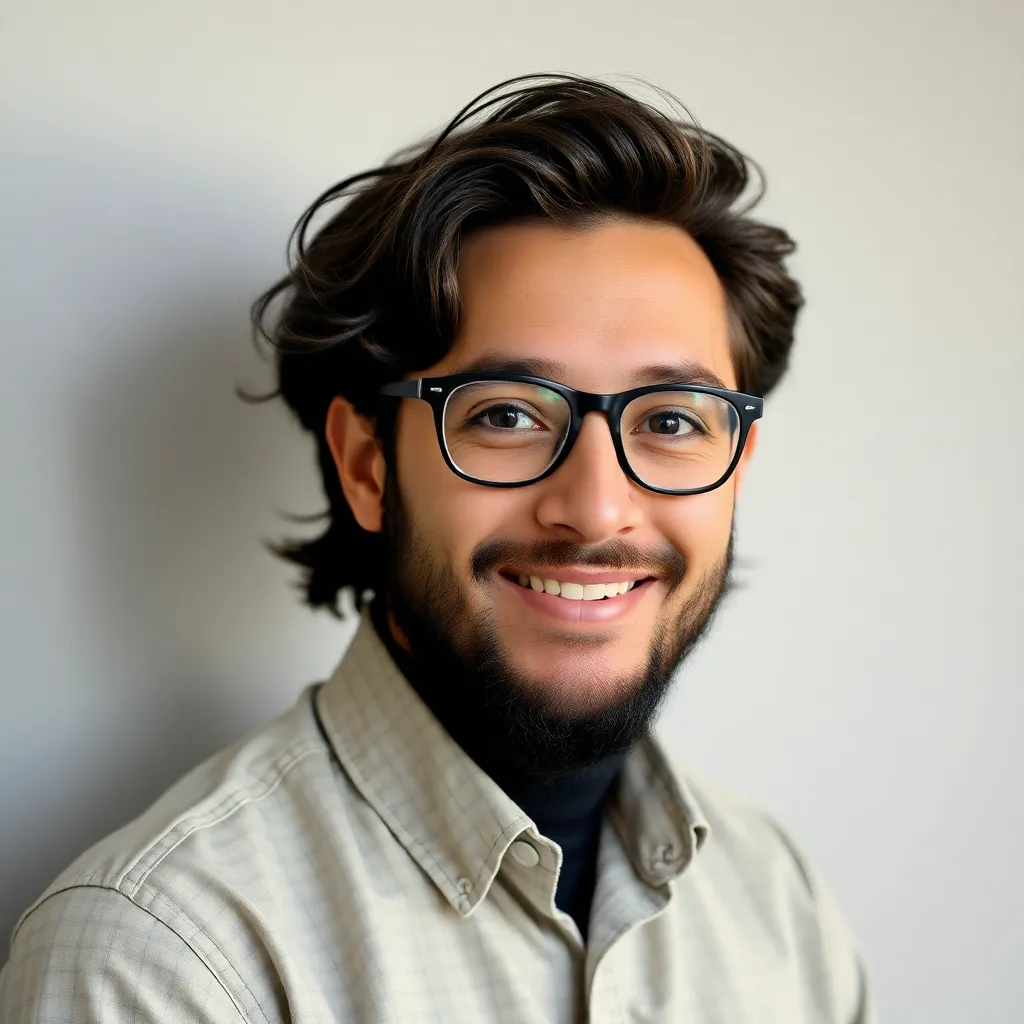
Onlines
Apr 12, 2025 · 7 min read

Table of Contents
Selecting the True Statements About the Process of Learning AI
The field of Artificial Intelligence (AI) is rapidly evolving, impacting various sectors from healthcare to finance. Understanding the learning process within AI is crucial for anyone seeking to enter this exciting domain or simply grasp its fundamental concepts. This article delves deep into the nuances of AI learning, debunking myths and highlighting key truths. We'll explore various learning paradigms, the challenges involved, and the future directions of AI learning.
Understanding AI Learning Paradigms
AI learning isn't a monolithic process; rather, it encompasses several distinct paradigms. Each method possesses unique strengths and weaknesses, applicable to different tasks and datasets.
1. Supervised Learning: Learning from Labeled Data
Supervised learning is the most common and arguably the most intuitive approach to AI learning. It involves training an AI model on a large dataset of labeled examples. Each data point is tagged with the correct output, allowing the model to learn the mapping between inputs and outputs. Think of it like teaching a child to identify different fruits: you show them apples, oranges, and bananas, labeling each one accordingly. The child learns to associate the visual features with the corresponding labels.
True Statements about Supervised Learning:
- It requires labeled data: This is a fundamental requirement. Without labeled data, the model has no way to learn the correct associations.
- It's effective for classification and regression tasks: Supervised learning excels at tasks like image classification, spam detection (classifying emails as spam or not spam), and predicting house prices (regression).
- Model performance depends on data quality: Noisy, incomplete, or biased data can significantly impact the model's accuracy and reliability. Garbage in, garbage out.
- It can be computationally expensive for large datasets: Training complex models on massive datasets requires significant computational resources and time.
- Overfitting is a potential problem: A model that overfits the training data performs poorly on unseen data. Regularization techniques are often employed to mitigate this.
2. Unsupervised Learning: Discovering Patterns in Unlabeled Data
Unlike supervised learning, unsupervised learning deals with unlabeled data. The AI model is tasked with identifying patterns, structures, and relationships within the data without any prior knowledge of the correct outputs. This is analogous to letting a child explore a box of toys and categorize them based on their similarities, without any external guidance.
True Statements about Unsupervised Learning:
- It's used for exploratory data analysis: It helps uncover hidden patterns and structures that may not be immediately apparent.
- Clustering is a common unsupervised learning technique: Clustering algorithms group similar data points together, useful for customer segmentation or anomaly detection.
- Dimensionality reduction is another key application: Techniques like Principal Component Analysis (PCA) reduce the number of variables while preserving important information, simplifying data analysis and improving model performance.
- Evaluating performance is more challenging: Without labeled data, assessing the quality of the learned patterns is more subjective and requires careful consideration of domain expertise.
- It can be used for anomaly detection: By identifying data points that deviate significantly from the established patterns, unsupervised learning can detect outliers, such as fraudulent transactions or faulty equipment.
3. Reinforcement Learning: Learning Through Trial and Error
Reinforcement learning involves an agent that interacts with an environment, learning through trial and error. The agent receives rewards for desirable actions and penalties for undesirable ones. The goal is to learn a policy—a strategy for selecting actions—that maximizes cumulative rewards over time. This is similar to teaching a dog a trick: rewarding it when it performs the trick correctly and correcting it when it makes a mistake.
True Statements about Reinforcement Learning:
- It's used for control problems: Reinforcement learning is particularly well-suited for controlling robots, autonomous vehicles, and game-playing agents.
- It involves an agent, environment, and reward function: These three components are essential for defining a reinforcement learning problem.
- Exploration-exploitation dilemma is a key challenge: The agent must balance exploring new actions to discover better strategies with exploiting the currently known best actions.
- Model-free and model-based approaches exist: Model-free methods learn directly from experience, while model-based methods build a model of the environment to guide learning.
- It requires careful design of the reward function: A poorly designed reward function can lead to unintended and undesirable behavior from the agent.
The Challenges of AI Learning
The journey of building and training AI models is far from straightforward. Numerous challenges can hinder the process, requiring careful consideration and skillful problem-solving.
Data Acquisition and Preparation: A Foundation for Success
The quality and quantity of data are paramount. Gathering sufficient, high-quality data can be a significant hurdle, especially for specialized domains. Data cleaning, preprocessing, and feature engineering are essential steps to ensure the data is suitable for training. Data bias is a critical concern; biased data leads to biased models, perpetuating and amplifying existing societal inequalities.
Model Selection and Hyperparameter Tuning: Finding the Right Fit
Choosing the right AI model for a specific task is crucial. Different models have different strengths and weaknesses, and the optimal choice depends on the data and the problem's characteristics. Hyperparameter tuning—optimizing the model's internal parameters—is essential for achieving optimal performance. This process is often iterative and can be computationally expensive.
Computational Resources: The Demands of Deep Learning
Training complex AI models, particularly deep learning models, requires significant computational resources. This can be a barrier for individuals or organizations with limited access to powerful hardware like GPUs or cloud computing infrastructure.
Interpretability and Explainability: Understanding the "Black Box"
Many advanced AI models, especially deep neural networks, are often referred to as "black boxes" due to their complexity and lack of transparency. Understanding how these models arrive at their predictions is crucial for building trust and ensuring accountability. Research into explainable AI (XAI) is actively addressing this challenge.
Ethical Considerations: Responsible AI Development
The development and deployment of AI systems raise several ethical concerns. Bias in data and algorithms, job displacement, privacy issues, and the potential for misuse are all critical considerations. Responsible AI development requires careful attention to these ethical implications.
The Future of AI Learning: Emerging Trends
The field of AI learning is constantly evolving, with several emerging trends shaping its future:
Transfer Learning: Leveraging Pre-trained Models
Transfer learning involves leveraging pre-trained models, trained on massive datasets, to solve new problems with limited data. This significantly reduces training time and data requirements, making AI more accessible.
Federated Learning: Collaborative Learning without Data Sharing
Federated learning allows multiple entities to collaboratively train a shared AI model without directly sharing their data. This addresses privacy concerns while still enabling the development of powerful, data-rich models.
Reinforcement Learning with Human Feedback: Bridging the Gap
Reinforcement learning with human feedback incorporates human expertise to guide the learning process, improving the efficiency and effectiveness of reinforcement learning algorithms.
Continual Learning: Adapting to Evolving Data
Continual learning aims to enable AI models to continuously learn and adapt to new data without forgetting previously learned information. This is crucial for building AI systems that can operate reliably in dynamic environments.
Explainable AI (XAI): Opening the "Black Box"
XAI focuses on developing techniques that make AI models more interpretable and transparent, increasing trust and accountability. This is essential for building responsible and reliable AI systems.
Conclusion: A Journey of Continuous Learning
The process of learning in AI is a multifaceted journey, encompassing diverse paradigms, significant challenges, and exciting future directions. By understanding the fundamental principles of supervised, unsupervised, and reinforcement learning, along with the inherent challenges and emerging trends, we can better navigate the complexities of this rapidly evolving field. The true statements outlined above provide a robust foundation for those seeking to deepen their knowledge and contribute to the advancement of AI. Continuous learning and adaptation are not just crucial for AI systems but equally important for those working within this exciting domain. The future of AI hinges on our ability to build responsible, robust, and ethical AI systems—a future shaped by those who embrace the continuous learning process.
Latest Posts
Latest Posts
-
Catcher In The Rye Chapter 20 Summary
Apr 18, 2025
-
Customer Segments Cannot Be Based On Sales Volume And Profitability
Apr 18, 2025
-
Why Would A Technician Enter The Command Copy Startup Config Running Config
Apr 18, 2025
-
A 20 Year Old Male Has A Large Laceration
Apr 18, 2025
-
Who Killed Barry Cuda Answer Key
Apr 18, 2025
Related Post
Thank you for visiting our website which covers about Select The True Statements About The Process Of Learning Ai. . We hope the information provided has been useful to you. Feel free to contact us if you have any questions or need further assistance. See you next time and don't miss to bookmark.