Statistics Is The Science Of Conducting Studies To
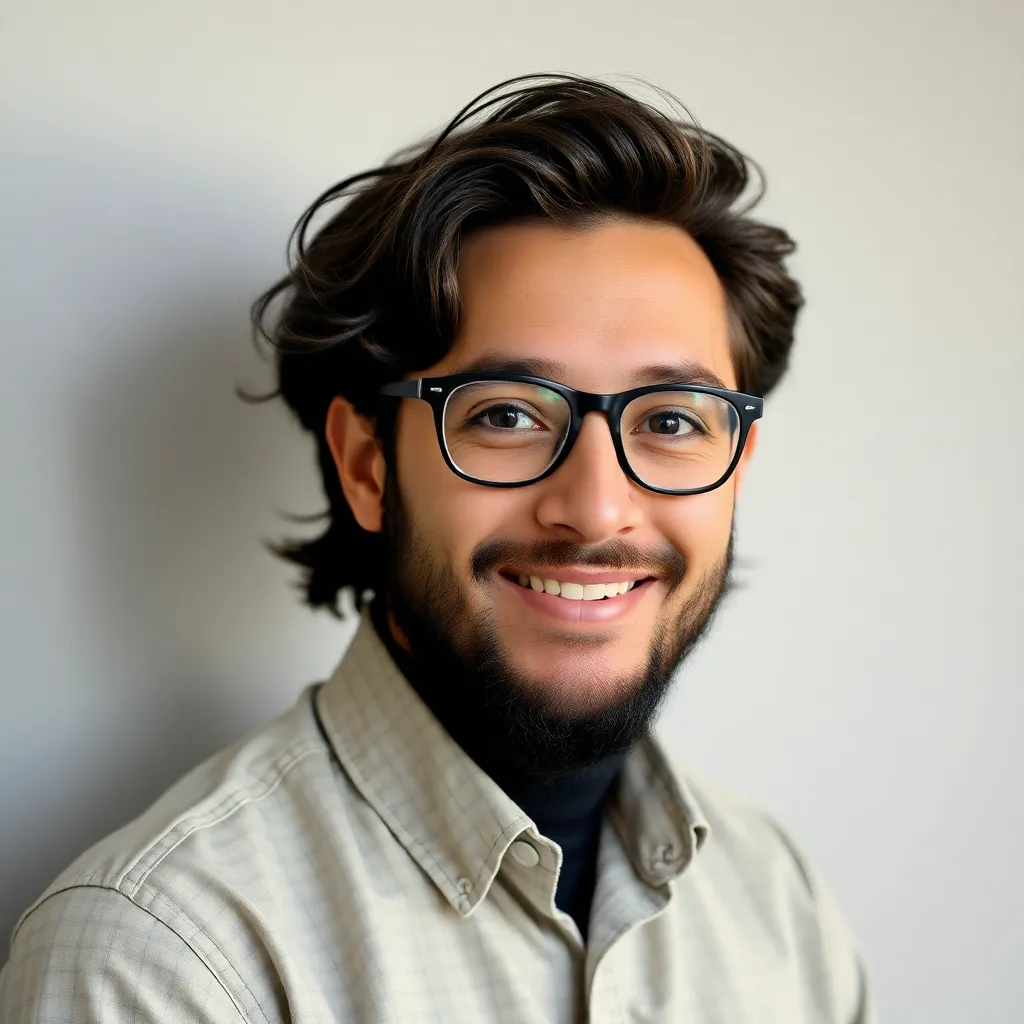
Onlines
May 09, 2025 · 6 min read
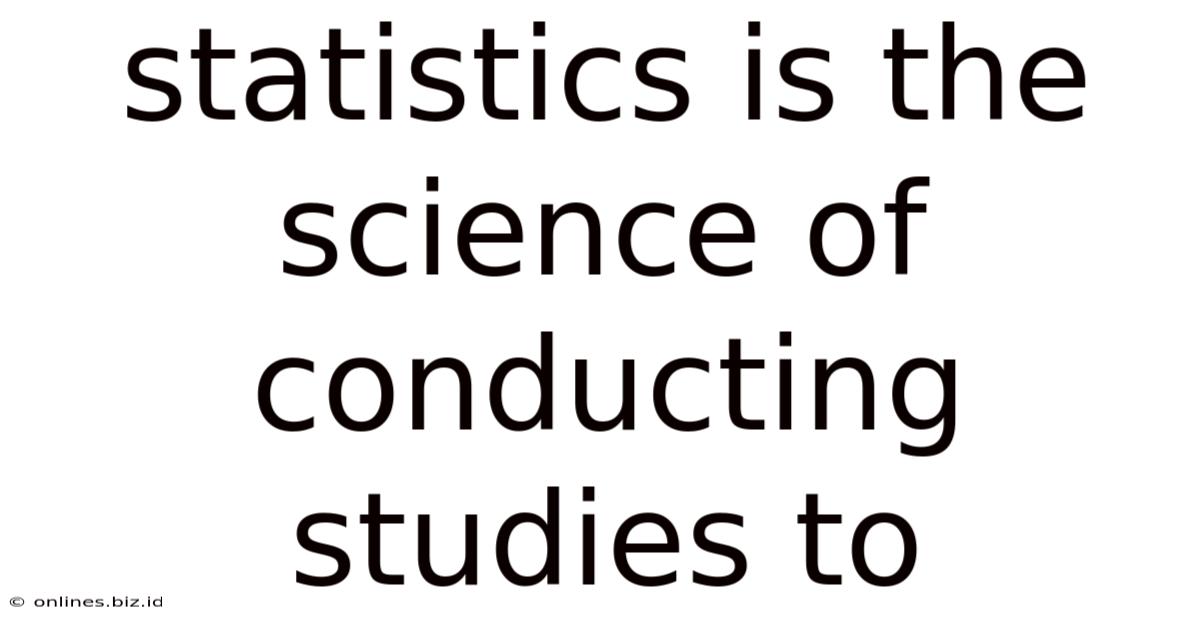
Table of Contents
Statistics: The Science of Conducting Studies to Understand and Interpret Data
Statistics is more than just numbers; it's the powerful science of collecting, analyzing, interpreting, presenting, and organizing data. It's the bedrock upon which informed decisions are made across countless fields, from healthcare and finance to marketing and environmental science. This comprehensive article delves into the core principles of statistics, exploring its various branches, applications, and the critical role it plays in shaping our understanding of the world.
Understanding the Scope of Statistics
At its heart, statistics aims to extract meaningful insights from data. This process involves several key stages:
1. Defining the Research Question:
Before diving into data collection, a clear and well-defined research question is paramount. This question dictates the type of data needed, the methods used for analysis, and ultimately, the conclusions drawn. For example, a researcher might ask: "What is the average income of households in a specific city?" or "Is there a correlation between smoking and lung cancer?"
2. Data Collection:
Data collection is the process of gathering raw information relevant to the research question. This can involve various methods, including:
- Surveys: Questionnaires distributed to a sample population to gather opinions, attitudes, and behaviors.
- Experiments: Controlled studies designed to establish cause-and-effect relationships between variables.
- Observations: Recording events or behaviors without manipulation.
- Existing Data: Utilizing readily available datasets, such as government records or company databases.
The method chosen depends on the research question and available resources. Sampling techniques, crucial for ensuring representativeness, are employed to select a subset of the population for study. This minimizes cost and time while maximizing the accuracy of inferences drawn about the broader population.
3. Data Analysis:
This stage involves applying statistical methods to summarize, describe, and interpret the collected data. This encompasses:
-
Descriptive Statistics: Summarizing the main features of the data using measures like mean, median, mode, standard deviation, and variance. These provide a concise overview of the data's central tendency, dispersion, and shape. Visual representations such as histograms, box plots, and scatter plots are commonly used to communicate these descriptive statistics effectively.
-
Inferential Statistics: Drawing conclusions about a population based on a sample of data. This includes hypothesis testing, confidence intervals, and regression analysis. Inferential statistics allow researchers to make generalizations beyond the observed data and assess the reliability of their findings. Understanding the concepts of p-values, statistical significance, and confidence levels are essential for interpreting results accurately.
4. Interpretation and Presentation:
The final stage involves interpreting the analytical results in the context of the research question. This requires careful consideration of potential limitations, biases, and confounding factors. Clear and concise communication of findings is essential, often through reports, presentations, or publications. Effective data visualization plays a critical role in conveying complex information to a wider audience.
The Two Major Branches of Statistics: Descriptive and Inferential
Statistics is broadly categorized into two main branches:
Descriptive Statistics: Painting a Picture of the Data
Descriptive statistics focuses on summarizing and describing the main features of a dataset. It doesn't involve making generalizations beyond the observed data. Key aspects include:
-
Measures of Central Tendency: Mean (average), median (middle value), and mode (most frequent value) describe the central location of the data. The choice of measure depends on the data's distribution (e.g., skewed data might favor the median over the mean).
-
Measures of Dispersion: Range, variance, and standard deviation quantify the spread or variability of the data. A high standard deviation indicates greater variability, while a low standard deviation suggests data points are clustered closely around the mean.
-
Data Visualization: Histograms, bar charts, pie charts, scatter plots, and box plots are powerful tools for visualizing data distributions, trends, and relationships between variables. Effective visualization enhances understanding and communication of findings.
Inferential Statistics: Drawing Conclusions Beyond the Data
Inferential statistics moves beyond simply describing the data to making inferences and predictions about a larger population based on a sample. This involves:
-
Hypothesis Testing: Formulating a hypothesis (a statement about the population) and testing it using sample data. This involves calculating a test statistic and comparing it to a critical value to determine whether to reject or fail to reject the null hypothesis.
-
Confidence Intervals: Estimating a range of values within which a population parameter (e.g., mean, proportion) is likely to fall with a certain level of confidence.
-
Regression Analysis: Examining the relationship between two or more variables. Linear regression models, for instance, predict the value of one variable based on the value of another.
Key Statistical Concepts and Methods
Several crucial concepts underpin statistical analysis:
-
Population and Sample: The population is the entire group of interest, while the sample is a subset selected from the population for study. Proper sampling techniques are crucial for ensuring the sample is representative of the population.
-
Variables: Characteristics or attributes that can be measured or observed. Variables can be categorical (e.g., gender, eye color) or numerical (e.g., age, height, income).
-
Probability Distributions: Mathematical functions that describe the probability of different outcomes of a random variable. Common distributions include the normal distribution, binomial distribution, and Poisson distribution.
-
Statistical Significance: The probability of observing the obtained results (or more extreme results) if the null hypothesis were true. A statistically significant result suggests that the observed effect is unlikely due to chance.
-
Correlation vs. Causation: Correlation measures the association between two variables, but it doesn't necessarily imply causation. A strong correlation doesn't automatically mean one variable causes the other.
Applications of Statistics Across Diverse Fields
Statistics plays a vital role in a vast array of fields:
Healthcare:
- Clinical Trials: Evaluating the effectiveness of new drugs and treatments.
- Epidemiology: Investigating the distribution and determinants of diseases.
- Public Health: Monitoring health trends and planning interventions.
Finance:
- Investment Analysis: Assessing risk and return of investments.
- Actuarial Science: Calculating insurance premiums and reserves.
- Financial Modeling: Forecasting financial performance.
Marketing:
- Market Research: Understanding consumer behavior and preferences.
- Advertising Effectiveness: Measuring the impact of advertising campaigns.
- Customer Segmentation: Grouping customers based on shared characteristics.
Environmental Science:
- Environmental Monitoring: Tracking pollution levels and environmental changes.
- Climate Change Research: Analyzing climate data and modeling future scenarios.
- Conservation Biology: Studying endangered species and habitat loss.
Social Sciences:
- Sociology: Studying social structures and behaviors.
- Psychology: Investigating human behavior and mental processes.
- Political Science: Analyzing political opinions and voting patterns.
The Importance of Data Integrity and Ethical Considerations
The reliability of statistical analyses hinges on the integrity of the data. Data quality issues, such as missing values, outliers, and measurement errors, can significantly impact the results. Robust data cleaning and preprocessing techniques are vital to ensure accuracy.
Furthermore, ethical considerations are paramount. Researchers must adhere to ethical guidelines regarding data collection, analysis, and reporting. This includes obtaining informed consent from participants, protecting privacy, and avoiding bias in the research process.
Conclusion: Statistics – A Foundation for Evidence-Based Decision-Making
Statistics is an indispensable tool for understanding and interpreting data. Its applications are ubiquitous, impacting decisions across all sectors of society. By mastering the principles of data collection, analysis, and interpretation, individuals and organizations can make informed decisions based on evidence, leading to better outcomes and improved understanding of the complex world around us. The future of statistics lies in harnessing the power of big data and advanced analytical techniques to address increasingly complex challenges and unlock new insights. Continuous learning and adaptation to evolving methodologies are essential for anyone seeking to utilize the full potential of this powerful science.
Latest Posts
Latest Posts
-
An Ignition Switch Uses A Switch
May 11, 2025
-
Una Estudiante Desea Hablar Con Su Profesora De Biologia
May 11, 2025
-
For Which Writing Prompt Would You Use An Explanatory Thesis
May 11, 2025
-
Old Lithosphere Is Destroyed In Association With
May 11, 2025
-
Qualitative Energy Storage And Conservation With Bar Graphs
May 11, 2025
Related Post
Thank you for visiting our website which covers about Statistics Is The Science Of Conducting Studies To . We hope the information provided has been useful to you. Feel free to contact us if you have any questions or need further assistance. See you next time and don't miss to bookmark.