Stochastic Calculus University Of Nebraska Lincoln
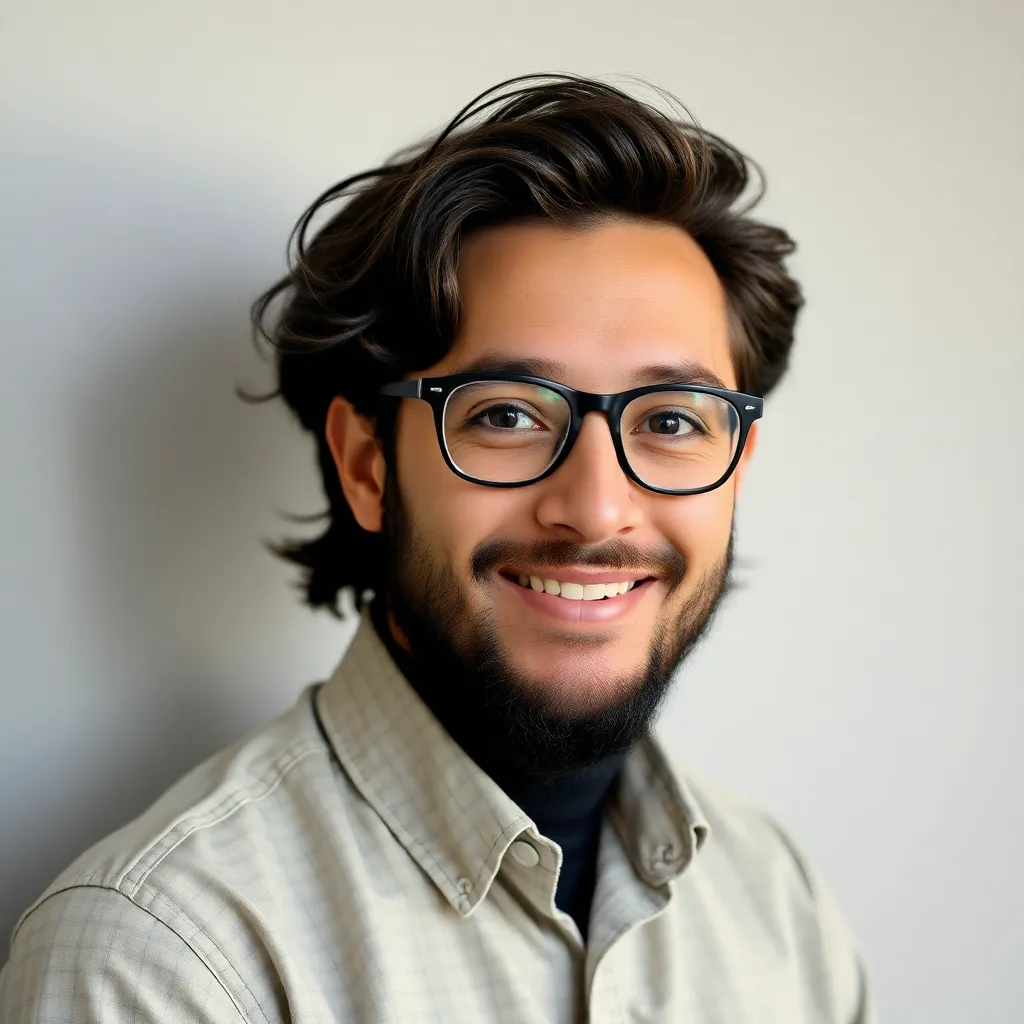
Onlines
Apr 09, 2025 · 5 min read
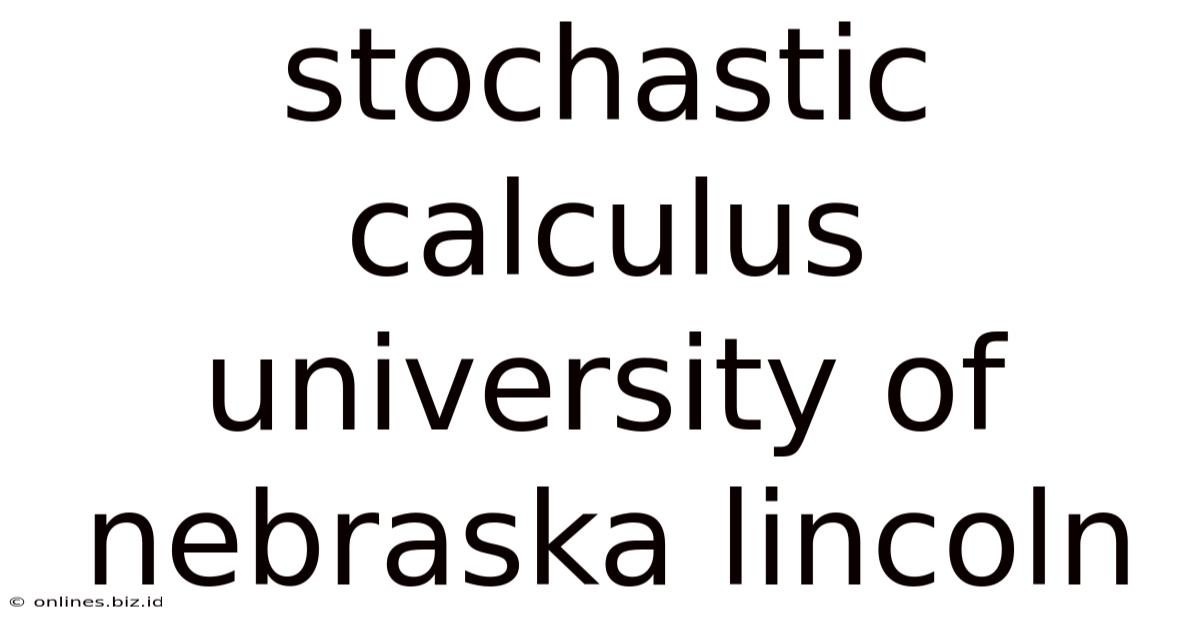
Table of Contents
- Stochastic Calculus University Of Nebraska Lincoln
- Table of Contents
- Stochastic Calculus at the University of Nebraska-Lincoln: A Deep Dive
- The Building Blocks: Probability Theory and Measure Theory
- Probability Theory: The Foundation
- Measure Theory: The Rigorous Framework
- Stochastic Processes: The Dynamic Element
- Markov Chains
- Martingales
- Brownian Motion and Stochastic Integrals
- Stochastic Differential Equations (SDEs)
- Advanced Topics and Applications
- Finding Relevant Courses at UNL
- Conclusion
- Latest Posts
- Related Post
Stochastic Calculus at the University of Nebraska-Lincoln: A Deep Dive
The University of Nebraska-Lincoln (UNL) doesn't offer a specific course explicitly titled "Stochastic Calculus." However, the mathematical foundation and advanced concepts crucial to understanding stochastic calculus are deeply embedded within their diverse mathematics curriculum. This article delves into the relevant courses and topics likely covered at UNL, exploring the core concepts of stochastic calculus and how they are likely integrated into their programs. We'll cover probability theory, measure theory, stochastic processes, and applications, providing a comprehensive overview relevant to a UNL student's potential learning path.
The Building Blocks: Probability Theory and Measure Theory
Before tackling the intricacies of stochastic calculus, a strong foundation in probability theory and measure theory is essential. At UNL, these subjects are likely covered in undergraduate and graduate-level courses.
Probability Theory: The Foundation
A solid understanding of probability theory is the cornerstone for stochastic calculus. Courses at UNL likely cover topics such as:
- Axiomatic probability: The fundamental axioms of probability, including the Kolmogorov axioms, are likely discussed rigorously. Students learn to work with probability spaces, events, and random variables.
- Discrete and continuous random variables: The characteristics of both discrete and continuous random variables are analyzed, along with their probability distributions (e.g., binomial, Poisson, normal, exponential).
- Expected value and variance: Calculating the expected value and variance of random variables is a key skill emphasized. Students learn techniques for handling both discrete and continuous cases.
- Conditional probability and independence: Understanding conditional probability and the concept of independence between random variables are crucial for modeling complex systems. Bayes' theorem is likely a key element covered.
- Joint distributions and covariance: Working with multiple random variables requires understanding joint probability distributions, covariance, and correlation.
- Limit theorems: The Law of Large Numbers and the Central Limit Theorem are essential for understanding the behavior of sums of random variables and their asymptotic properties. This is critical for understanding stochastic convergence.
Measure Theory: The Rigorous Framework
While probability theory provides intuition, measure theory offers the rigorous mathematical framework necessary for advanced probability and stochastic calculus. UNL’s advanced courses likely cover:
- Measure spaces and sigma-algebras: The concepts of sigma-algebras, measurable functions, and measure spaces are fundamental. Students learn to rigorously define probability measures on these spaces.
- Integration theory: Lebesgue integration is crucial for working with more general random variables and probability distributions. Students learn about integration with respect to a measure.
- Convergence theorems: Important convergence theorems like the Monotone Convergence Theorem, Dominated Convergence Theorem, and Fatou's Lemma provide tools for manipulating limits of integrals. This is essential for proving many results in stochastic calculus.
- Radon-Nikodym theorem: This theorem is crucial for understanding conditional expectation and other important concepts in stochastic processes.
Stochastic Processes: The Dynamic Element
Stochastic processes are sequences of random variables indexed by time, forming the core of stochastic calculus. UNL's graduate-level courses would likely cover:
Markov Chains
- Discrete-time Markov chains: Analyzing the transition probabilities, stationary distributions, and long-run behavior of Markov chains is vital.
- Continuous-time Markov chains: Understanding the transition rates and solving the Kolmogorov forward and backward equations is essential.
Martingales
- Definition and properties: Understanding the concept of a martingale and its properties (e.g., optional stopping theorem) is fundamental.
- Martingale convergence theorems: These theorems are essential for understanding the long-run behavior of martingales.
Brownian Motion and Stochastic Integrals
- Brownian motion: The properties of Brownian motion (a key stochastic process) are studied extensively. Students likely learn about its continuity, non-differentiability, and Markov properties.
- Stochastic integrals: Constructing stochastic integrals with respect to Brownian motion is a central component. This involves defining integrals using Riemann sums, dealing with issues of non-differentiability, and understanding Itô's lemma.
- Itô's Lemma: This fundamental theorem is the cornerstone of many applications of stochastic calculus, enabling the calculation of differentials of functions of stochastic processes.
Stochastic Differential Equations (SDEs)
- Introduction to SDEs: Learning how to model dynamic systems with randomness using stochastic differential equations is a critical part of the curriculum.
- Solution methods: Exploring different methods for solving SDEs, such as numerical methods, is important for practical applications.
- Applications of SDEs: Students likely examine applications in various fields such as finance (modeling asset prices), physics (modeling diffusion processes), and biology (modeling population dynamics).
Advanced Topics and Applications
Depending on the specific courses and research interests, UNL's graduate program might delve into more advanced topics:
- Lévy processes: Generalizations of Brownian motion with jumps.
- Stochastic control theory: Optimizing stochastic systems over time.
- Malliavin calculus: A differential calculus for stochastic processes.
- Financial modeling: Applying stochastic calculus to financial derivatives pricing and risk management.
- Numerical methods for SDEs: Developing and applying numerical algorithms for solving SDEs.
Finding Relevant Courses at UNL
To pinpoint the exact courses covering these topics, a prospective student should consult the UNL Department of Mathematics and Statistics website. Look for courses in probability, measure theory, stochastic processes, and potentially applied mathematics that might incorporate stochastic calculus elements. Examining course descriptions and syllabi will provide the most accurate information.
Conclusion
While UNL doesn't explicitly advertise a "Stochastic Calculus" course, the foundational mathematical concepts and advanced topics are undeniably part of their rigorous mathematics curriculum. By taking a sequence of courses in probability, measure theory, and advanced stochastic processes, students can gain a strong understanding of stochastic calculus and its numerous applications. This deep dive provides a roadmap for navigating UNL's offerings and acquiring the expertise in this vital area of mathematics. Remember to consult UNL's official website for the most up-to-date information on course offerings and curriculum details. The journey into stochastic calculus at UNL promises a rewarding experience for dedicated students.
Latest Posts
Related Post
Thank you for visiting our website which covers about Stochastic Calculus University Of Nebraska Lincoln . We hope the information provided has been useful to you. Feel free to contact us if you have any questions or need further assistance. See you next time and don't miss to bookmark.