The Automatic Identification Of Material Is Part Of/facilitated By:
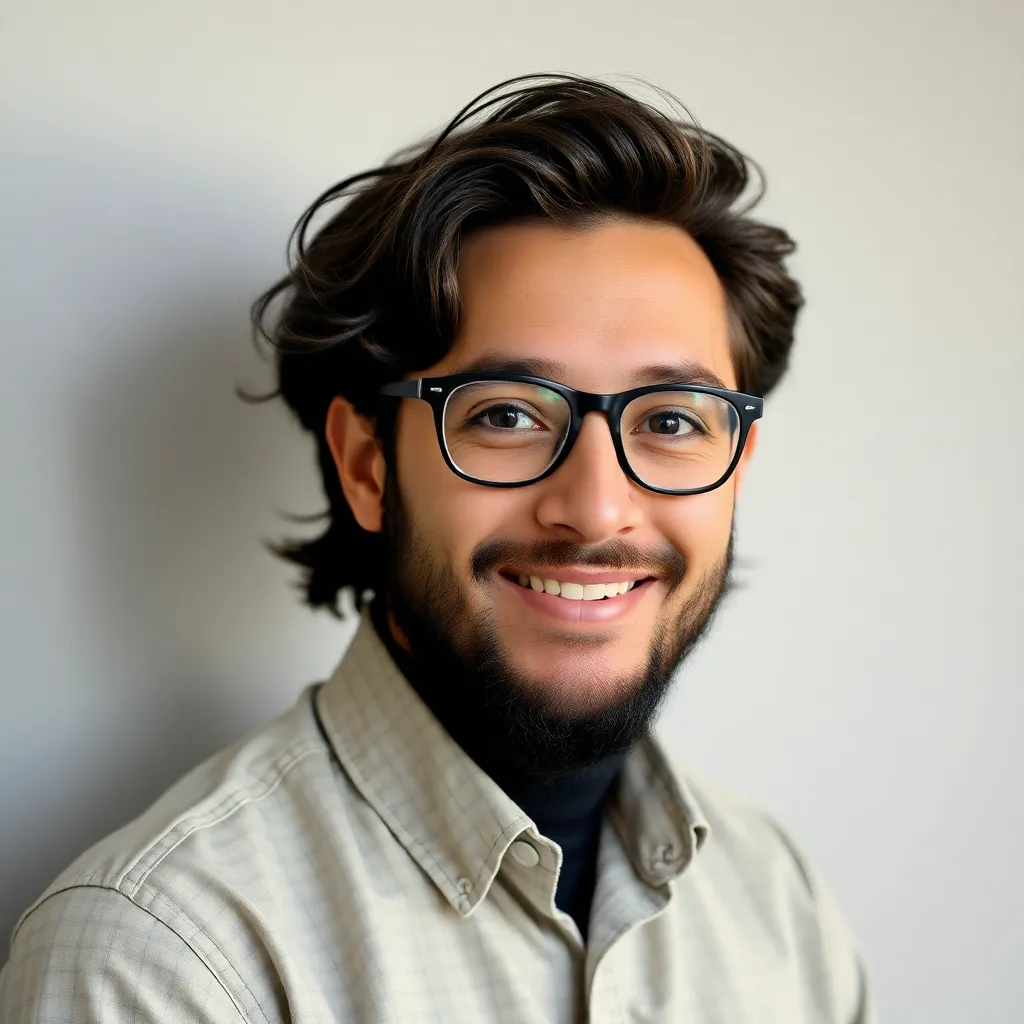
Onlines
Apr 08, 2025 · 6 min read

Table of Contents
The Automatic Identification of Material: A Deep Dive into Enabling Technologies
The automatic identification of materials is no longer a futuristic fantasy; it's a rapidly evolving reality with profound implications across numerous industries. This ability to instantly and accurately determine the composition and properties of a substance is revolutionizing manufacturing, quality control, environmental monitoring, and even healthcare. But how is this achieved? This article delves into the core technologies and processes that facilitate the automatic identification of materials, exploring their strengths, limitations, and future potential.
Spectroscopy: Unlocking Material Secrets Through Light
Spectroscopy forms the bedrock of many automatic material identification systems. This family of techniques analyzes how materials interact with electromagnetic radiation – from radio waves to gamma rays – to reveal their unique fingerprints. Different materials absorb, transmit, and reflect light differently depending on their molecular structure and composition. By measuring these interactions, we can identify the material.
1. Infrared (IR) Spectroscopy: Molecular Vibrations Unveiled
Infrared spectroscopy exploits the vibrations of molecules. When IR light interacts with a material, specific wavelengths are absorbed, creating a unique absorption spectrum. This spectrum acts as a "fingerprint," allowing for precise material identification. IR spectroscopy finds widespread application in analyzing polymers, pharmaceuticals, and organic materials. Its strengths lie in its speed, relatively low cost, and versatility. However, sample preparation can sometimes be challenging, and spectral interpretation might require expertise.
2. Raman Spectroscopy: Molecular Scattering for Material Analysis
Raman spectroscopy is another powerful vibrational spectroscopic technique. It relies on the inelastic scattering of light, where the scattered photons have slightly different energy than the incident photons. This energy shift provides information about the vibrational modes of the molecules, again producing a unique spectral signature for material identification. Raman spectroscopy is particularly advantageous for analyzing aqueous solutions and offers excellent spatial resolution. However, it can be sensitive to fluorescence, which can interfere with the signal.
3. UV-Vis Spectroscopy: Electronic Transitions and Material Characterization
Ultraviolet-visible (UV-Vis) spectroscopy analyzes the absorption and transmission of light in the ultraviolet and visible regions. This technique is sensitive to electronic transitions within molecules, providing information about their electronic structure and concentration. UV-Vis spectroscopy is widely used in the pharmaceutical industry for purity analysis and in environmental monitoring for detecting pollutants. Its simplicity and speed make it a valuable tool, although it may not be as specific as other spectroscopic techniques for complex mixtures.
4. X-ray Spectroscopy: Unveiling Elemental Composition
X-ray spectroscopy goes beyond molecular structure, focusing on the elemental composition of materials. Techniques like X-ray fluorescence (XRF) and X-ray diffraction (XRD) use X-rays to probe the material, providing detailed information about the elements present and their arrangement. XRF is particularly useful for rapid, non-destructive analysis of metals, alloys, and geological samples. XRD, on the other hand, provides information about the crystal structure of the material, vital for identifying crystalline phases and their orientations.
Imaging Techniques: Visualizing Material Properties
Beyond spectroscopy, imaging techniques play a crucial role in automatic material identification. These methods provide visual information about the material's structure, texture, and surface characteristics, which can be combined with spectroscopic data for a more comprehensive analysis.
1. Microscopy: Zooming in on Material Microstructures
Microscopy encompasses a range of techniques that magnify the material's structure to reveal fine details. Optical microscopy, electron microscopy (SEM, TEM), and atomic force microscopy (AFM) each offer different levels of magnification and provide information about the material's surface morphology, internal structure, and even atomic arrangement. Microscopy is indispensable in materials science, enabling the characterization of defects, grain boundaries, and other microstructural features that significantly influence material properties. However, these techniques often require specialized expertise and can be time-consuming.
2. Computed Tomography (CT) Scanning: Three-Dimensional Material Insights
CT scanning uses X-rays to create detailed three-dimensional images of the material's internal structure. This non-destructive technique is particularly useful for inspecting complex components, detecting internal defects, and analyzing the distribution of different phases within a material. CT scanning is widely used in industrial quality control, medical imaging, and geological exploration. Its ability to provide volumetric information makes it a powerful tool for understanding the internal structure of materials.
Other Enabling Technologies
Several other technologies contribute significantly to the automatic identification of materials.
1. Machine Learning and Artificial Intelligence (AI): Data-Driven Material Identification
Machine learning algorithms are transforming material identification by analyzing vast amounts of spectroscopic and imaging data. These algorithms can identify patterns and relationships that may not be immediately apparent to human experts, leading to more accurate and efficient identification. AI-powered systems can learn from existing datasets and improve their accuracy over time, making them increasingly valuable for complex material identification tasks.
2. Hyperspectral Imaging: Combining Imaging and Spectroscopy
Hyperspectral imaging combines the spatial information of imaging with the spectral information of spectroscopy. This technique captures hundreds or thousands of narrow spectral bands for each pixel in an image, providing rich spectral and spatial data. Hyperspectral imaging is a powerful tool for identifying materials in complex scenes, such as remotely sensing vegetation or identifying minerals in geological samples. However, processing and analyzing the large datasets generated by hyperspectral imaging requires significant computational resources.
Applications Across Industries
The ability to automatically identify materials has far-reaching implications across various sectors:
- Manufacturing: Quality control, process optimization, and failure analysis.
- Recycling: Sorting and classifying materials for efficient recycling processes.
- Healthcare: Diagnosing diseases, monitoring patient conditions, and developing new medical devices.
- Environmental Monitoring: Detecting pollutants, assessing environmental risks, and monitoring ecosystem health.
- Forensic Science: Analyzing evidence, identifying materials of interest in criminal investigations.
- Food Safety: Ensuring food quality, detecting contaminants, and preventing foodborne illnesses.
Challenges and Future Directions
Despite significant advancements, several challenges remain:
- Complex mixtures: Identifying materials in complex mixtures can be challenging, especially when multiple materials have overlapping spectral signatures.
- Data analysis: Processing and interpreting large datasets generated by advanced techniques like hyperspectral imaging require substantial computational resources.
- Standardization: Lack of standardization in data formats and analysis methods can hinder the development of interoperable systems.
- Cost: Advanced instrumentation and software can be expensive, limiting access for smaller organizations.
Future research will likely focus on:
- Developing more robust and versatile algorithms for data analysis.
- Improving the sensitivity and specificity of spectroscopic and imaging techniques.
- Developing portable and cost-effective instruments for on-site analysis.
- Integrating different technologies to create more comprehensive material identification systems.
- Expanding the application of AI and machine learning for automated material identification.
In conclusion, the automatic identification of materials is a rapidly evolving field driven by significant advancements in spectroscopy, imaging, and data analysis techniques. This technology is transforming industries by enabling faster, more efficient, and more accurate material characterization. Addressing the remaining challenges and continued research will further unlock the potential of automatic material identification, shaping a future where materials analysis is faster, cheaper, and more accessible than ever before.
Latest Posts
Latest Posts
-
Bohr Model Of Hydrogen Gizmo Answers
Apr 17, 2025
-
Me Aburren Las Matematicas Bore Please Bother Enchant
Apr 17, 2025
-
Which Option Below Is An Example Of A Rhetorical Question
Apr 17, 2025
-
3 1 Periodic Phenomena Practice Set 1
Apr 17, 2025
-
The Metering Pins In Oleo Shock Struts Serve To
Apr 17, 2025
Related Post
Thank you for visiting our website which covers about The Automatic Identification Of Material Is Part Of/facilitated By: . We hope the information provided has been useful to you. Feel free to contact us if you have any questions or need further assistance. See you next time and don't miss to bookmark.