The Empirical Rule States All The Following Except
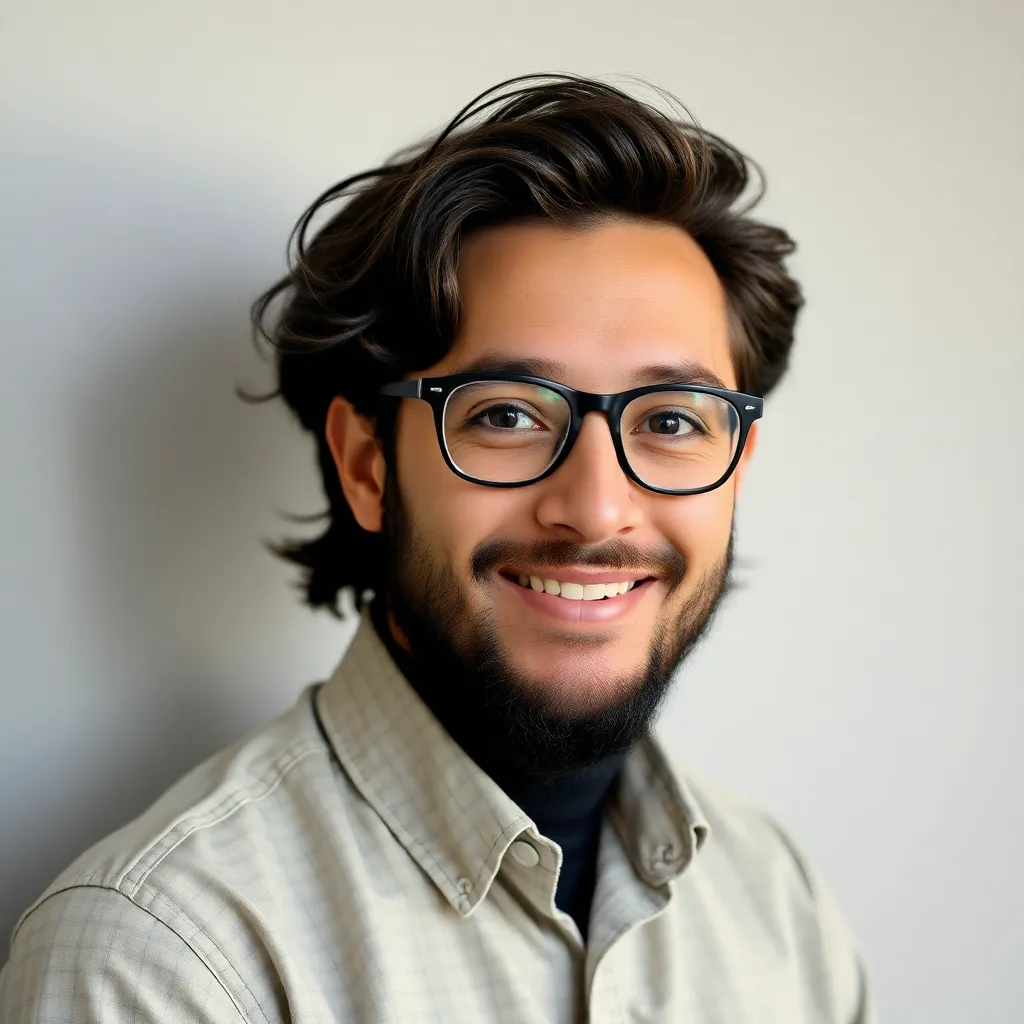
Onlines
May 03, 2025 · 6 min read

Table of Contents
The Empirical Rule: All the Following Except…
The Empirical Rule, also known as the 68-95-99.7 rule, is a fundamental concept in statistics that describes the distribution of data in a normal distribution. Understanding this rule is crucial for interpreting data and making informed decisions in various fields, from finance and healthcare to engineering and social sciences. This rule provides a handy guideline for estimating probabilities within one, two, and three standard deviations of the mean. But what does the Empirical Rule not state? Let's delve into the specifics and explore its limitations.
What the Empirical Rule Does State
Before we address the exception, it's vital to understand precisely what the Empirical Rule claims. It states that for a normal distribution:
-
Approximately 68% of the data falls within one standard deviation of the mean. This means that if you calculate the mean and standard deviation of your data, about 68% of the data points will lie between one standard deviation below the mean and one standard deviation above the mean. This range can be expressed as (µ - σ, µ + σ), where µ represents the mean and σ represents the standard deviation.
-
Approximately 95% of the data falls within two standard deviations of the mean. Extending the range, approximately 95% of the data points will lie between two standard deviations below the mean and two standard deviations above the mean. This is represented as (µ - 2σ, µ + 2σ).
-
Approximately 99.7% of the data falls within three standard deviations of the mean. Finally, the vast majority, roughly 99.7%, of the data points are expected to fall within three standard deviations of the mean: (µ - 3σ, µ + 3σ).
These percentages are approximations, and the actual percentages may vary slightly depending on the specific dataset. However, the Empirical Rule provides a reasonably accurate estimation for many normally distributed datasets.
Visualizing the Empirical Rule
It’s often easier to grasp the Empirical Rule visually. Imagine a bell curve, the classic representation of a normal distribution. The mean sits at the center of this curve. The standard deviation determines the spread or width of the curve.
The Empirical Rule can be visualized as follows:
-
One Standard Deviation: The area under the curve between one standard deviation below the mean and one standard deviation above the mean represents approximately 68% of the total area under the curve.
-
Two Standard Deviations: The area under the curve between two standard deviations below the mean and two standard deviations above the mean represents approximately 95% of the total area.
-
Three Standard Deviations: The area between three standard deviations below and three standard deviations above the mean encompasses almost the entire distribution (approximately 99.7%).
Understanding the Limitations: What the Empirical Rule Doesn't State
The Empirical Rule, while incredibly useful, is not a universally applicable principle. Its accuracy is heavily dependent on the data following a normal distribution. This is the key limitation. The Empirical Rule does not state:
-
It applies to all data sets: This is the most crucial point. The Empirical Rule is only accurate for data that is approximately normally distributed. Many real-world datasets are not normally distributed; they might be skewed, bimodal, or follow entirely different distributions. Applying the Empirical Rule to such data will yield inaccurate and misleading results. This is the primary answer to the question "The Empirical Rule states all the following except..." It doesn't state it applies to all datasets.
-
Precise percentages for any range: The Empirical Rule provides approximations. The percentages (68%, 95%, 99.7%) are not exact and will vary slightly depending on the specific normal distribution. More precise values require using the Z-table or statistical software.
-
Information about data outside three standard deviations: While 99.7% of data falls within three standard deviations, the rule doesn't explicitly address the remaining 0.3%. This small percentage, though seemingly insignificant, can be crucial in certain applications.
-
Information on specific percentiles beyond the 68-95-99.7 range: The Empirical Rule specifically targets the percentages within one, two, and three standard deviations. It doesn't directly provide information about, for example, the percentage of data within 1.5 standard deviations or the percentile associated with a specific z-score.
-
Applicability to small sample sizes: The Empirical Rule is more accurate with larger sample sizes. With smaller sample sizes, the observed distribution may not accurately reflect the true underlying distribution, leading to inaccuracies in applying the rule.
Beyond the Empirical Rule: Dealing with Non-Normal Data
When dealing with datasets that are not normally distributed, alternative methods are necessary to estimate probabilities and percentiles. These methods include:
-
Data Transformation: Transforming the data using techniques like logarithmic or square root transformations can sometimes make the data more closely resemble a normal distribution.
-
Non-parametric methods: Non-parametric methods, like the rank-based methods (e.g., Spearman's rank correlation), do not assume a specific distribution and can be used for analyzing data that is not normally distributed.
-
Bootstrapping: Bootstrapping is a resampling technique that can be used to estimate the sampling distribution of a statistic, regardless of the underlying data distribution.
-
Kernel Density Estimation (KDE): KDE is a non-parametric method used to estimate the probability density function of a random variable. It's particularly useful when the underlying distribution is unknown or non-normal.
Applications and Importance of the Empirical Rule
Despite its limitations, the Empirical Rule remains a valuable tool in many statistical applications because of its simplicity and intuitive nature. Its applications include:
-
Quality Control: In manufacturing, the Empirical Rule helps determine acceptable tolerances and identify outliers.
-
Risk Assessment: In finance, it can be used to model and assess the risk associated with investments.
-
Healthcare: In medical research, it helps to understand and interpret the distribution of biological data.
-
Environmental Science: It's useful in analyzing environmental data and identifying unusual patterns.
-
Educational Assessment: In education, it helps understand student performance distribution around average scores.
Conclusion: A Practical Guide to the Empirical Rule
The Empirical Rule is a powerful and easily understood concept that provides a quick approximation of probabilities in normally distributed data. It helps to quickly assess the spread and characteristics of a dataset. However, it's vital to remember that it is only an approximation and only applies to approximately normal distributions. Failing to acknowledge this limitation can lead to erroneous conclusions. Always check for normality before applying the Empirical Rule, and consider alternative methods when dealing with non-normal data. Understanding both the capabilities and limitations of the Empirical Rule is essential for effective data analysis and informed decision-making in diverse fields. By using it appropriately and in conjunction with other statistical methods, you can unlock valuable insights from your data.
Latest Posts
Latest Posts
-
The Climax Of The Star Spangled Banner Falls On What Text
May 03, 2025
-
Biology Roots Llc Cells Answer Key
May 03, 2025
-
Which Document Contains A Format For The Systems Engineering Plan
May 03, 2025
-
Draw The Product Formed By The Reaction Of Potassium T Butoxide
May 03, 2025
-
Which Statement About Greek Sculpture Is False
May 03, 2025
Related Post
Thank you for visiting our website which covers about The Empirical Rule States All The Following Except . We hope the information provided has been useful to you. Feel free to contact us if you have any questions or need further assistance. See you next time and don't miss to bookmark.