The Filters Quadrant Is Used To
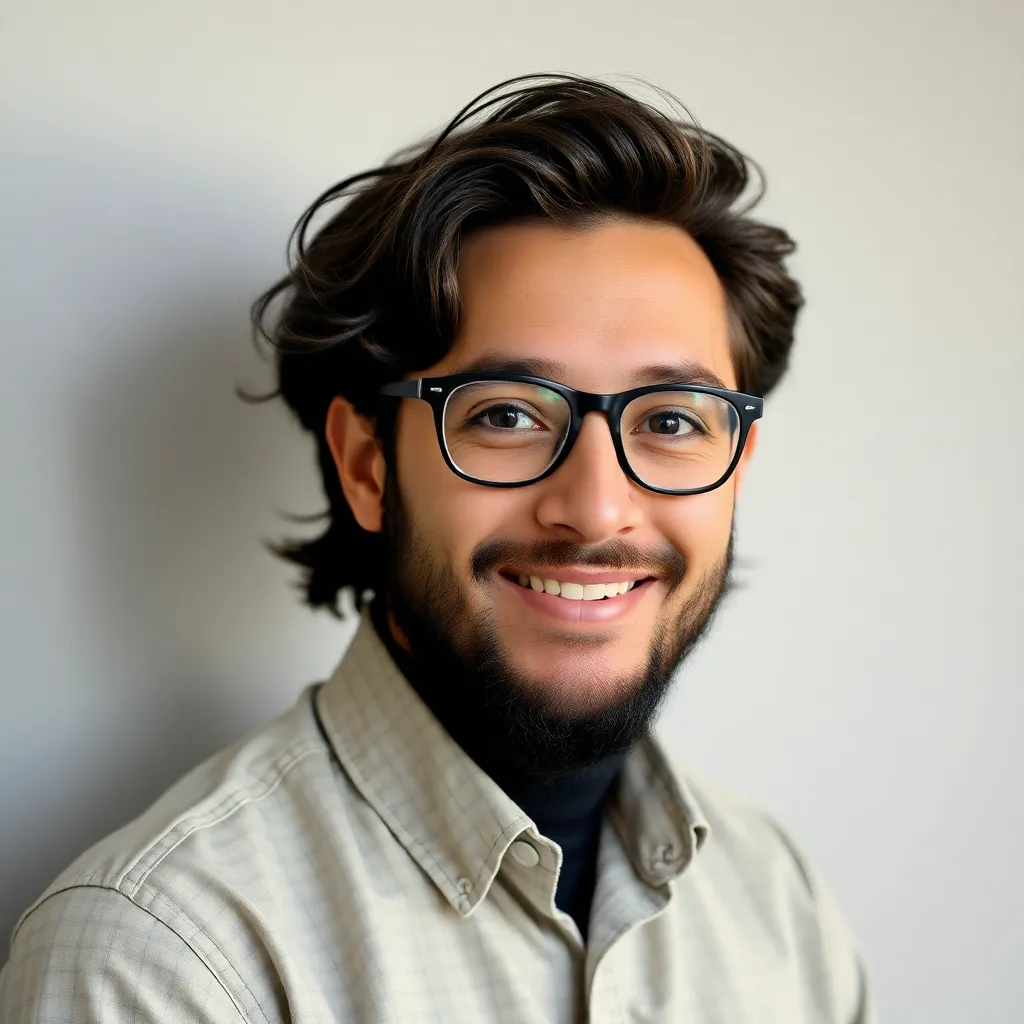
Onlines
May 06, 2025 · 6 min read

Table of Contents
The Filters Quadrant: A Deep Dive into Data Analysis and Decision-Making
The Filters Quadrant, while not a formally named or universally recognized concept in standard statistical or data analysis literature, represents a powerful conceptual framework for understanding how filtering techniques are employed to refine data and inform decision-making. It's built upon the idea that effective data analysis often requires a multi-faceted approach to filtering, using different criteria and methods to isolate relevant information and suppress noise. This article will explore the four key dimensions that constitute this hypothetical quadrant: Time, Value, Source, and Type. We'll examine how each dimension provides a unique lens for filtering data, and how their combined use leads to more accurate, actionable insights.
Understanding the Four Dimensions of the Filters Quadrant
Imagine a four-quadrant chart. Each quadrant represents a crucial filtering dimension:
-
Quadrant 1: Time Filtering: This focuses on the temporal aspect of your data. Are you interested in recent data, historical trends, or specific periods? This dimension allows you to isolate data points based on their timestamp or date range.
-
Quadrant 2: Value Filtering: Here, you filter based on the numerical or categorical value of the data itself. This could involve setting thresholds, applying ranges, or selecting specific categories. For example, you might filter for transactions over a certain amount or customers belonging to a specific demographic.
-
Quadrant 3: Source Filtering: This focuses on the origin or provider of your data. This is crucial when dealing with data from multiple sources, potentially with varying levels of accuracy or reliability. You might filter to include only data from trusted sources or exclude data from known problematic sources.
-
Quadrant 4: Type Filtering: This dimension involves filtering based on the data type itself. Are you interested in numerical data, textual data, images, or a specific data format? This helps you focus on the specific kind of information relevant to your analysis.
Time Filtering: Capturing Temporal Insights
Time filtering is arguably the most common filtering method. Whether you're analyzing website traffic, stock prices, or customer behavior, understanding the temporal patterns is critical. Effective time filtering involves:
Defining Relevant Time Frames:
- Real-time: For immediate insights, such as monitoring server performance or live social media sentiment.
- Short-term: Focusing on daily, weekly, or monthly data for identifying recent trends or anomalies.
- Long-term: Analyzing yearly or even multi-year data to identify long-term patterns and trends.
- Specific periods: Focusing on particular events, such as holidays, product launches, or marketing campaigns, to assess their impact.
Techniques for Time Filtering:
- Date ranges: Selecting data within a specific start and end date.
- Rolling windows: Analyzing moving averages or other metrics over a defined time window, useful for smoothing out data fluctuations.
- Time series analysis: Employing techniques like ARIMA or exponential smoothing to model and forecast temporal trends.
- Event-based filtering: Focusing on data points related to specific events or occurrences.
Value Filtering: Isolating Key Data Points
Value filtering is essential for highlighting the most significant data points within your dataset. This often involves:
Setting Thresholds and Ranges:
- Upper and lower bounds: Filtering data points above or below specific values.
- Percentile-based filtering: Selecting data points within a certain percentile range, such as the top 10% or bottom 25%.
- Standard deviation filtering: Identifying outliers by filtering data points outside a certain number of standard deviations from the mean.
Categorical Value Filtering:
- Specific categories: Selecting data points belonging to specific categories, such as product types or customer segments.
- Exclusion of categories: Removing data points belonging to specific categories.
- Combination of categories: Selecting data points matching specific combinations of categories.
Source Filtering: Ensuring Data Reliability
Source filtering is crucial for maintaining the integrity and reliability of your analysis. This involves:
Identifying Data Sources:
- Internal databases: Data collected within your organization, such as CRM data or sales records.
- External APIs: Data sourced from third-party providers, such as weather data or social media analytics.
- Public datasets: Data available from government agencies or research institutions.
Evaluating Data Quality:
- Accuracy: Assessing the correctness and validity of the data.
- Completeness: Determining whether the data is comprehensive and free of missing values.
- Consistency: Checking for uniformity and lack of contradictions in the data.
- Relevance: Ensuring the data is relevant to the research question.
Type Filtering: Focusing on Relevant Data Types
Type filtering allows you to concentrate on the specific data types needed for your analysis:
Common Data Types:
- Numerical data: Quantitative data such as sales figures, temperatures, or stock prices.
- Categorical data: Qualitative data representing categories or groups, such as customer segments or product types.
- Textual data: Unstructured data such as customer reviews, social media posts, or survey responses.
- Image data: Visual data used for image recognition or analysis.
- Time series data: Data collected over time, such as stock prices or weather patterns.
Methods for Type Filtering:
- Data type selection: Choosing only the relevant data types for your analysis.
- Data transformation: Converting data from one type to another, such as converting text data into numerical data using techniques like tokenization and one-hot encoding.
- Data cleaning: Handling missing or inconsistent data, which often involves removing or imputing missing values.
Combining the Dimensions for Powerful Analysis
The true power of the Filters Quadrant lies in combining these four dimensions. By strategically applying filters across multiple dimensions, you can drastically refine your data and gain more precise insights. For instance, you might filter website traffic data to show only:
- Time: Data from the last month
- Value: Sessions with an average session duration greater than 5 minutes
- Source: Traffic originating from Google Ads
- Type: Only numerical data representing session duration and bounce rate
This combined filtering approach provides a far more focused and actionable analysis than examining the raw, unfiltered data.
Practical Applications of the Filters Quadrant
The Filters Quadrant finds applications across numerous fields:
- Business Analytics: Analyzing sales data, customer behavior, marketing campaign performance, and website traffic.
- Financial Analysis: Evaluating investment portfolios, identifying market trends, and managing risk.
- Healthcare: Analyzing patient data, tracking disease outbreaks, and improving treatment outcomes.
- Scientific Research: Analyzing experimental data, identifying patterns, and drawing conclusions.
- Social Sciences: Analyzing survey data, studying social trends, and understanding public opinion.
Conclusion: Mastering the Art of Data Filtering
The Filters Quadrant, although a conceptual model, provides a robust framework for thinking about data filtering. By systematically considering the four dimensions – Time, Value, Source, and Type – you can significantly improve the accuracy, efficiency, and effectiveness of your data analysis. Mastering the art of data filtering is crucial for anyone working with data, enabling them to extract meaningful insights and make informed decisions. Remember that the specific filters and their combinations will vary greatly depending on the specific context and the questions you're trying to answer. The key is to think critically about your data, understand its limitations, and employ the appropriate filtering techniques to gain actionable knowledge.
Latest Posts
Latest Posts
-
The Secret Structure Of Great Talks
May 06, 2025
-
Using This Grid Color A Perfect Square Larger Than 16
May 06, 2025
-
Global Economy George Sarraf Pdf Free Download
May 06, 2025
-
The Following Events Pertain To Super Cleaning Company
May 06, 2025
-
Which Of The Following Does Not Belong To Holding Costs
May 06, 2025
Related Post
Thank you for visiting our website which covers about The Filters Quadrant Is Used To . We hope the information provided has been useful to you. Feel free to contact us if you have any questions or need further assistance. See you next time and don't miss to bookmark.