The Following Distribution Is Not A Probability Distribution Because
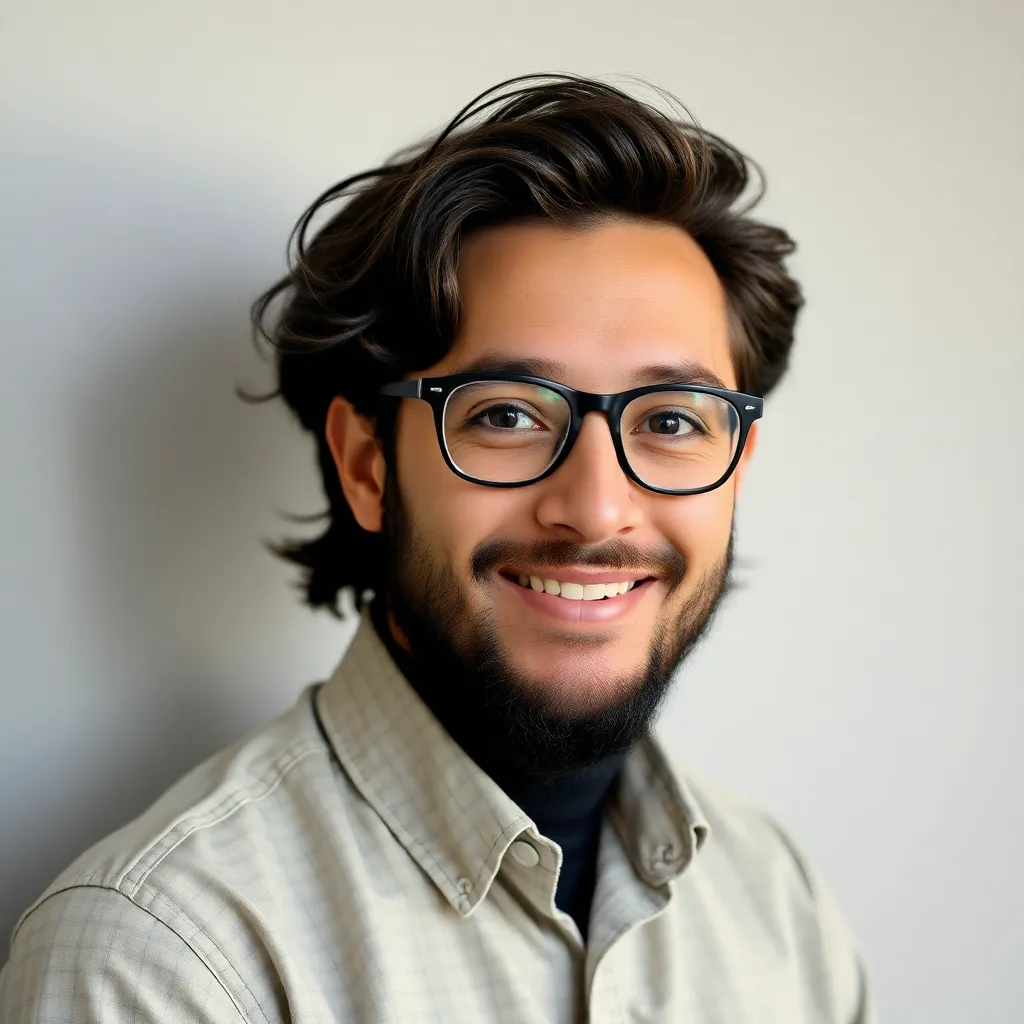
Onlines
Apr 09, 2025 · 5 min read

Table of Contents
The Following Distribution is Not a Probability Distribution Because...
A probability distribution is a fundamental concept in statistics and probability theory. It describes the likelihood of different outcomes for a random variable. To be a valid probability distribution, it must adhere to specific criteria. Understanding these criteria is crucial for accurately modeling real-world phenomena and making informed decisions based on data analysis. This article will explore the reasons why a given distribution might fail to meet these criteria and therefore not qualify as a valid probability distribution. We'll delve into the key properties, provide illustrative examples, and discuss practical implications.
The Defining Properties of a Probability Distribution
Before examining why a distribution might fail, let's clearly define the essential properties that a valid probability distribution must satisfy. These properties ensure that the distribution accurately reflects the probabilities of all possible outcomes.
1. Non-negativity:
The probability of any event must be non-negative. This is intuitive; probabilities cannot be negative values. Mathematically, for a discrete random variable X with possible outcomes x₁, x₂, ..., xₙ, and their corresponding probabilities P(X=xᵢ), we must have:
P(X=xᵢ) ≥ 0 for all i.
For a continuous random variable with probability density function f(x), we have:
f(x) ≥ 0 for all x.
2. Normalization:
The sum of probabilities for all possible outcomes must equal one. This reflects the certainty that one of the outcomes will occur. For a discrete distribution:
Σ P(X=xᵢ) = 1 (summing over all possible values of xᵢ).
For a continuous distribution:
∫ f(x) dx = 1 (integral over the entire range of x).
These two conditions, non-negativity and normalization, are the cornerstones of a valid probability distribution. Any distribution violating either is invalid.
Common Reasons Why a Distribution Fails to be a Probability Distribution
Let's explore specific scenarios where a distribution fails to meet the criteria and therefore is not a valid probability distribution:
1. Negative Probabilities:
This is a straightforward violation. If a distribution assigns negative probabilities to any outcome, it immediately disqualifies itself. Consider this example:
Example: Suppose we have a distribution for the number of heads in two coin flips:
- P(0 heads) = 0.2
- P(1 head) = 0.8
- P(2 heads) = -0.2
This is not a valid probability distribution because P(2 heads) = -0.2 is negative. Probabilities must always be non-negative.
2. Probabilities Summing to More or Less Than One:
If the sum of probabilities for all possible outcomes deviates from one, the distribution is invalid. This indicates an inconsistency in how probabilities are assigned.
Example: Consider a distribution for the outcome of rolling a six-sided die:
- P(1) = 0.1
- P(2) = 0.2
- P(3) = 0.3
- P(4) = 0.2
- P(5) = 0.1
- P(6) = 0.2
The sum of these probabilities is 1.2, which is greater than 1. This violates the normalization condition, making it an invalid probability distribution. The probabilities are overestimated.
3. Improperly Defined Ranges or Domains:
For continuous distributions, the integral of the probability density function over its entire domain must equal one. If the domain isn't correctly defined, or the function isn't integrable, the normalization condition will be violated.
Example: Imagine a probability density function defined as:
f(x) = x for 0 ≤ x ≤ 2
This is not a valid probability density function because the integral of f(x) from 0 to 2 is 2, not 1. The function needs to be scaled appropriately to satisfy the normalization condition.
4. Discrete Distributions with Non-Integer Probabilities:
While probabilities can be expressed as decimals, they represent proportions. It's important to avoid scenarios where a probability is so small or so large that it implies a non-integer number of successful occurrences within a fixed number of trials.
Example: Consider a scenario where there are only three possible outcomes: A, B, C.
- P(A) = 0.4
- P(B) = 0.5
- P(C) = 0.2
The probabilities sum to 1.1, which violates the normalization condition. This is an example where assigning probabilities without ensuring proper normalization leads to an invalid distribution.
5. Distributions with Infinite Probabilities:
In cases involving continuous random variables, the probability density function (PDF) can become unbounded (i.e., approach infinity). This does not in itself invalidate the distribution if the integral across the whole domain still integrates to 1. However, it can lead to complications in statistical calculations and interpretations. It's important to always check for integrability and normalization.
Example: A poorly defined PDF might include a point where it approaches infinity without an offsetting negative section. While it might integrate to 1, it would be highly impractical and lead to unrealistic results.
Implications of Using an Invalid Probability Distribution
Using an invalid probability distribution can have severe consequences in various applications. Some of the implications are:
-
Incorrect inferences and predictions: Analyzing data with an invalid distribution will lead to inaccurate conclusions. Any statistical models or predictions based on such a distribution will be flawed.
-
Biased estimates: Parameters estimated using an invalid probability distribution will be biased and unreliable, leading to erroneous interpretations.
-
Invalid hypothesis testing: Statistical hypothesis tests rely on the correctness of the probability distribution. Using an invalid one will invalidate the results of the test, leading to incorrect conclusions about the underlying hypothesis.
-
Misleading decision-making: Decisions made based on incorrect statistical analysis arising from invalid probability distributions can be costly and have significant real-world impacts.
Conclusion: Ensuring Validity for Accurate Analysis
The validity of a probability distribution is paramount in any statistical analysis. By carefully checking for non-negativity and normalization, and ensuring a well-defined range for continuous variables, one can ensure the accuracy and reliability of their statistical work. Using invalid distributions leads to erroneous results and unreliable conclusions. Understanding the properties and potential pitfalls associated with probability distributions is essential for anyone working with statistical data and making informed decisions based on data analysis. Always double-check your distributions to avoid pitfalls and ensure the validity of your conclusions. Remember to carefully consider the context of the problem and the nature of the data when assigning probabilities. Thorough verification guarantees the robustness of any statistical inference or predictive model built upon it.
Latest Posts
Latest Posts
-
Juror 3 Quotes With Page Numbers
Apr 17, 2025
-
Forensic Files Tourist Trap Answer Key
Apr 17, 2025
-
Review Sheet 13 Gross Anatomy Of The Muscular System
Apr 17, 2025
-
9 4 4 Implement An Enterprise Wireless Network
Apr 17, 2025
-
Civil Rights Movement Webquest Answer Key
Apr 17, 2025
Related Post
Thank you for visiting our website which covers about The Following Distribution Is Not A Probability Distribution Because . We hope the information provided has been useful to you. Feel free to contact us if you have any questions or need further assistance. See you next time and don't miss to bookmark.