The Frequency Distribution Shown Is Constructed Incorrectly
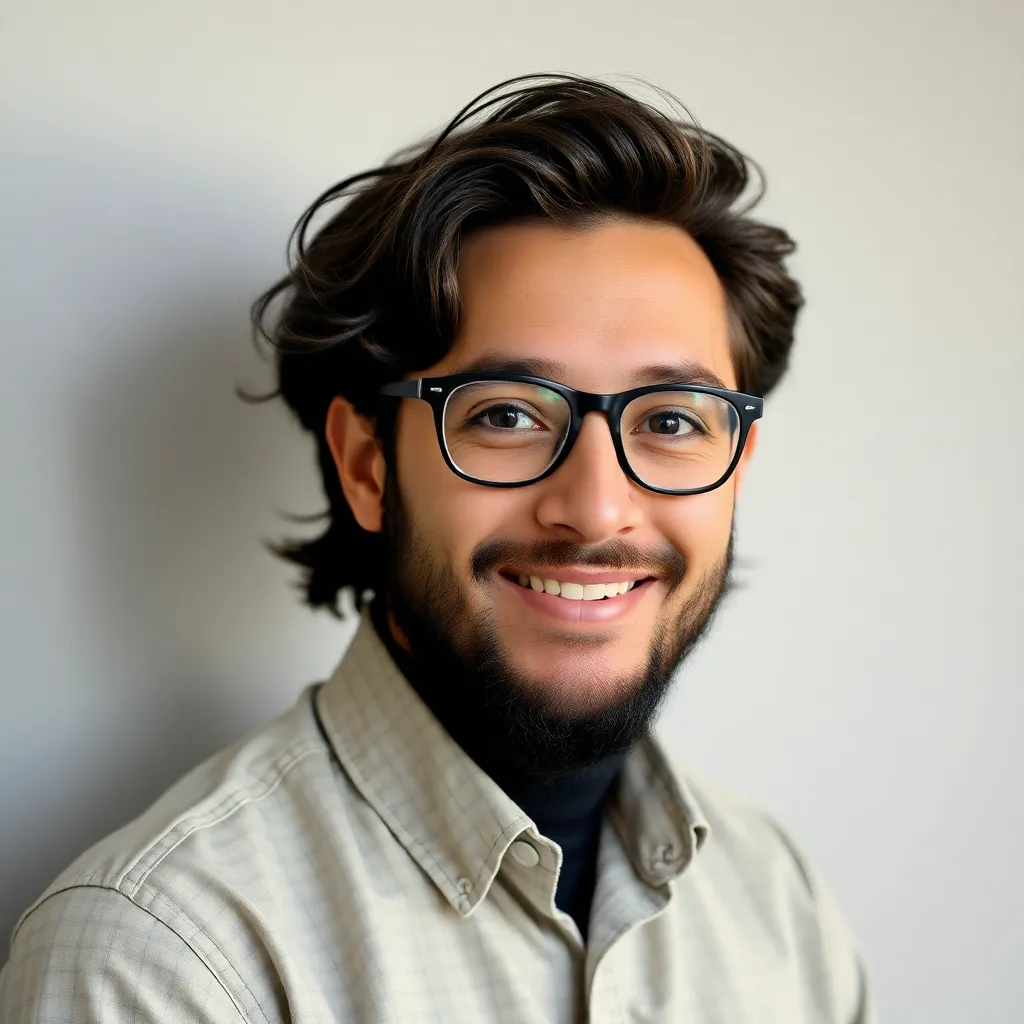
Onlines
Apr 18, 2025 · 6 min read

Table of Contents
The Frequency Distribution Shown is Constructed Incorrectly: Common Mistakes and How to Fix Them
Frequency distributions are fundamental tools in statistics, providing a clear and concise summary of data. They show how often different values or ranges of values occur within a dataset. However, creating an accurate and informative frequency distribution requires careful attention to detail. A poorly constructed frequency distribution can misrepresent the data, leading to flawed conclusions. This article delves into common errors in frequency distribution construction, explaining why they're problematic and offering solutions to rectify them.
Common Mistakes in Constructing Frequency Distributions
Several pitfalls can lead to an incorrectly constructed frequency distribution. Let's examine some of the most prevalent errors:
1. Incorrect Class Intervals: Overlapping or Unequal Intervals
One of the most frequent errors is the use of overlapping or unequal class intervals. Overlapping intervals cause ambiguity, making it unclear to which interval a particular data point belongs. For instance, consider the intervals 10-20 and 20-30. Where does the value 20 fall? This ambiguity renders the distribution unreliable.
Unequal intervals, while sometimes necessary for skewed data, complicate analysis and interpretation. They distort the visual representation of the data's distribution, making it difficult to identify patterns or trends. Analyzing data with unequal intervals requires more complex calculations and can lead to misinterpretations.
Example of Incorrect Intervals:
- Incorrect: 10-20, 20-30, 30-40 (Overlapping at 20 and 30)
- Incorrect: 10-20, 20-25, 25-40 (Unequal intervals)
- Correct: 10-19, 20-29, 30-39 (Clear, equal intervals)
2. Missing or Inconsistent Class Boundaries
Class boundaries define the precise limits of each interval. Missing or inconsistent boundaries make it impossible to accurately assign data points to the correct intervals. They create gaps or overlaps, resulting in an inaccurate frequency count for each class.
Consistent use of inclusive or exclusive boundaries is crucial. Inclusive boundaries include the upper limit of an interval, while exclusive boundaries exclude it. Consistency is essential; mixing both types within a single distribution will lead to errors.
Example of Missing/Inconsistent Boundaries:
- Incorrect: 10-20, 21-31, 32-42 (Inconsistent boundaries)
- Correct: 10-19.99, 20-29.99, 30-39.99 (Consistent exclusive boundaries)
3. Inaccurate Frequency Counts
Human error is a significant source of mistakes in frequency distributions. Inaccurate counting of data points within each interval leads to a distorted representation of the data. Double-checking the counts and using appropriate software or tools can significantly reduce the likelihood of these errors.
Techniques to prevent inaccurate frequency counts:
- Tally marks: Use tally marks to keep track of data points for each interval. This simple method helps improve accuracy and prevents skipping data points.
- Software and Spreadsheet Applications: Utilize software like Excel, SPSS, or R to generate frequency distributions. These tools provide automated counts and reduce the potential for human error.
4. Inappropriate Number of Classes
Choosing the right number of classes is crucial for a clear and informative frequency distribution. Too few classes obscure the underlying data pattern, while too many classes can result in a cluttered and uninterpretable distribution.
The optimal number of classes depends on the dataset's size and variability. Rules of thumb like Sturges' formula can be helpful, but the final choice often involves judgment based on the dataset's characteristics and the intended use of the distribution.
Sturges' Formula: k = 1 + 3.322 * log₁₀(n), where 'k' is the number of classes and 'n' is the number of data points.
Example of Inappropriate Number of Classes:
- Too few: A dataset of 100 data points might be inappropriately summarized with only 3 classes.
- Too many: The same dataset with 20 classes might result in a highly fragmented and uninformative distribution.
5. Lack of Clear Labels and Titles
A well-constructed frequency distribution must be easily understandable. Missing or unclear labels and titles make it difficult to interpret the data. The distribution should have a clear title, labels for each class interval, and a clear indication of the frequency counts for each interval. Units of measurement should also be specified if applicable.
Example of Poorly Labeled Distribution:
- Incorrect: 10-20, 20-30, 30-40 (No title, no labels for frequency)
- Correct: Frequency Distribution of Student Exam Scores
- 10-19: 5 students
- 20-29: 12 students
- 30-39: 18 students
6. Misinterpretation of Relative Frequency and Cumulative Frequency
Relative frequency and cumulative frequency are valuable additions to a frequency distribution, providing additional insights into the data. However, misinterpreting or miscalculating these values can lead to inaccurate conclusions.
- Relative frequency: Represents the proportion of data points in each interval. It's calculated by dividing the frequency of each interval by the total number of data points.
- Cumulative frequency: Represents the running total of frequencies up to a particular interval. It's obtained by adding the frequencies of all preceding intervals to the frequency of the current interval.
Incorrect calculations or a lack of understanding of these concepts can lead to faulty interpretations of the data’s distribution.
How to Correctly Construct a Frequency Distribution
Building a correct frequency distribution requires a systematic approach:
- Determine the range: Find the difference between the highest and lowest values in your dataset.
- Choose the number of classes: Use Sturges' formula or a similar rule of thumb, or choose a number that provides a clear and informative visualization.
- Determine the class width: Divide the range by the desired number of classes. Round up to a convenient number.
- Set the class boundaries: Establish clear and non-overlapping boundaries for each class. Maintain consistency in using either inclusive or exclusive boundaries.
- Tally the data: Count the number of data points falling within each class. Use tally marks to improve accuracy.
- Record the frequencies: Record the frequency (count) for each class.
- Calculate relative and cumulative frequencies (optional): Calculate the relative and cumulative frequencies to provide additional insights.
- Create a table or chart: Present the frequency distribution in a clear and well-labeled table or chart. Use appropriate titles and axis labels.
Impact of Incorrect Frequency Distributions
An incorrectly constructed frequency distribution can have several detrimental effects:
- Misleading conclusions: Incorrectly summarized data can lead to wrong inferences and flawed decision-making.
- Inaccurate statistical analysis: Subsequent statistical analyses based on the incorrect distribution will yield inaccurate results.
- Poor data visualization: A flawed distribution hampers the ability to effectively visualize and communicate the data's key features.
- Erosion of trust: Presenting inaccurate data erodes confidence in the researcher or organization presenting it.
Software Tools for Frequency Distribution Creation
Many statistical software packages and spreadsheet programs can simplify the creation of frequency distributions. These tools automate the process, minimizing the risk of manual errors and ensuring accuracy. These include:
- Microsoft Excel: Offers built-in functions to generate frequency distributions.
- SPSS: A comprehensive statistical package with powerful features for data analysis, including frequency distribution creation.
- R: A versatile open-source programming language and environment used extensively for statistical computing. It provides many packages specifically designed for data analysis and visualization.
Conclusion
Creating an accurate frequency distribution is crucial for effective data analysis and interpretation. By understanding and avoiding common errors, such as using overlapping or unequal intervals, making inaccurate frequency counts, and neglecting clear labeling, you can ensure your data is represented accurately and reliably. Utilizing appropriate software tools can also aid in this process, reducing the risk of manual errors and facilitating efficient data analysis. Remember that a well-constructed frequency distribution is the foundation for sound statistical inference and informed decision-making. Always double-check your work and ensure the distribution accurately reflects the underlying data.
Latest Posts
Latest Posts
-
When Dealing With Performance Evaluations Pim Has What Meaning
Apr 19, 2025
-
Next To Of Course God America I Analysis
Apr 19, 2025
-
The Pit And The Pendulum Theme
Apr 19, 2025
-
Periodic Table Of Acquisition Innovations Prize Competitions
Apr 19, 2025
-
Fine Print Renters Insurance Agreement Answer Key
Apr 19, 2025
Related Post
Thank you for visiting our website which covers about The Frequency Distribution Shown Is Constructed Incorrectly . We hope the information provided has been useful to you. Feel free to contact us if you have any questions or need further assistance. See you next time and don't miss to bookmark.