The Height Of 200 Adults Were Recorded And Divided
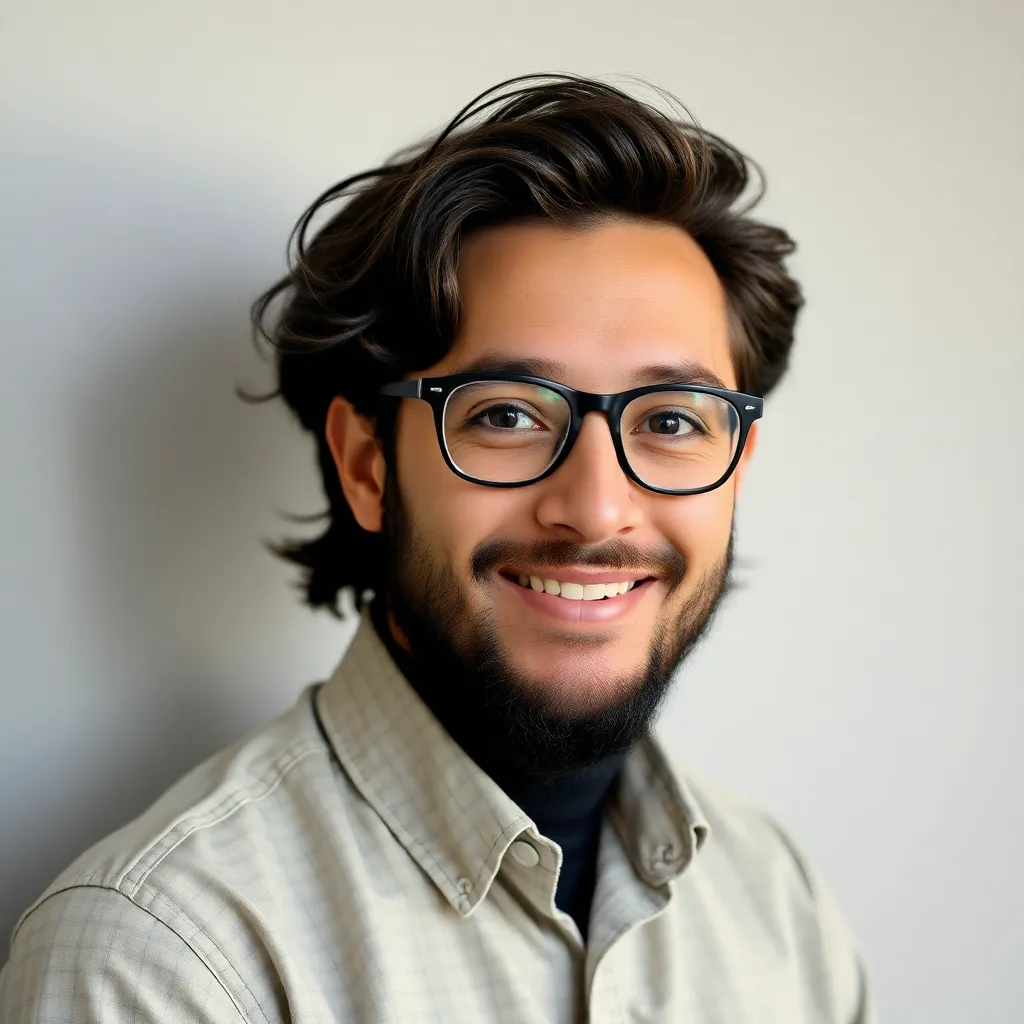
Onlines
May 12, 2025 · 6 min read
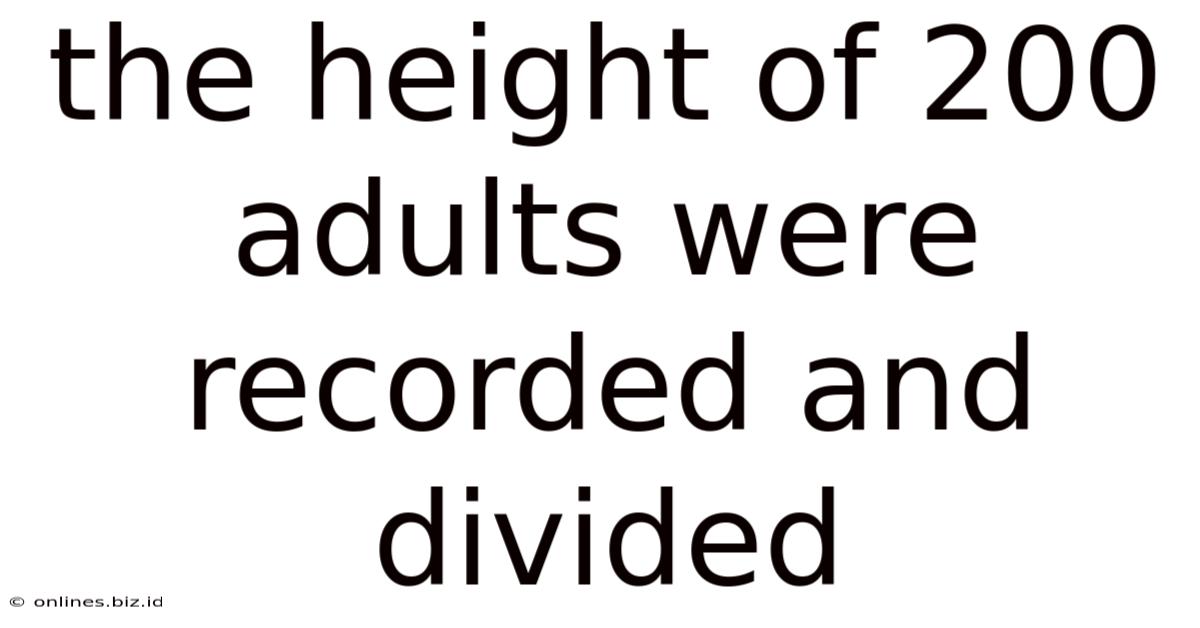
Table of Contents
The Height of 200 Adults: A Statistical Deep Dive
The seemingly simple act of recording the heights of 200 adults opens a door to a world of statistical analysis and inference. This data, seemingly mundane, becomes a powerful tool for understanding population characteristics, testing hypotheses, and illustrating core statistical concepts. This article will explore the potential analyses of such data, highlighting key statistical methods and their implications. We'll move beyond simple averages and delve into the richer tapestry of statistical insights hidden within this seemingly straightforward dataset.
Data Collection and Preparation
Before diving into the analysis, it's crucial to understand how the data was collected. A robust methodology ensures the reliability and validity of the subsequent analysis. Ideally, the 200 adults should represent a randomly selected sample from a defined population (e.g., adults in a specific city, a particular age group, etc.). This random sampling minimizes bias and allows for generalizations to the larger population.
The heights should be measured using a standardized method, ensuring consistency and accuracy. Any inconsistencies in measurement techniques (e.g., using different measuring tools, varying posture during measurement) can introduce error and compromise the data's integrity. The units of measurement (centimeters, inches, etc.) should be clearly defined and consistently applied throughout the dataset.
Once collected, the raw data needs to be organized and cleaned. This involves checking for outliers (unusually high or low values that might be due to errors), missing data, and inconsistencies. Outliers might require further investigation—were they measurement errors, or do they represent genuine extremes within the population? Missing data points might be handled through imputation (estimating missing values based on existing data), but this should be done cautiously and transparently.
Descriptive Statistics: Summarizing the Data
The first step in analyzing the height data is calculating descriptive statistics. These provide a concise summary of the dataset’s central tendency, dispersion, and shape.
Measures of Central Tendency
-
Mean: The average height, calculated by summing all heights and dividing by 200. This provides a single value representing the typical height.
-
Median: The middle value when the heights are arranged in ascending order. The median is less sensitive to outliers than the mean. If the number of data points is even, the median is the average of the two middle values.
-
Mode: The height that occurs most frequently. The mode might not be unique; a dataset could be bimodal (having two modes) or even multimodal.
The differences between the mean, median, and mode can reveal important information about the data's distribution. A significant difference between the mean and median suggests the presence of skewness (asymmetry) in the data.
Measures of Dispersion
-
Range: The difference between the highest and lowest heights. This provides a simple measure of the data's spread.
-
Variance: The average of the squared differences from the mean. It quantifies the variability around the mean. A larger variance indicates greater variability.
-
Standard Deviation: The square root of the variance. It's expressed in the same units as the data (e.g., centimeters) and provides a more interpretable measure of dispersion than the variance.
-
Interquartile Range (IQR): The difference between the 75th percentile (third quartile) and the 25th percentile (first quartile). The IQR is less sensitive to outliers than the range.
These measures of dispersion help understand how spread out the heights are. A small standard deviation indicates that the heights are clustered closely around the mean, while a large standard deviation suggests greater variability.
Data Visualization: Histograms and Box Plots
Visualizing the data is crucial for understanding its distribution.
-
Histograms: These graphical representations show the frequency distribution of heights. The x-axis represents the height intervals, and the y-axis represents the frequency (number of adults) in each interval. Histograms reveal the shape of the distribution (e.g., normal, skewed, bimodal).
-
Box Plots: These display the median, quartiles, and potential outliers. The box represents the IQR, the line inside the box represents the median, and whiskers extend to the minimum and maximum values within 1.5 times the IQR from the quartiles. Points beyond the whiskers are considered potential outliers.
By visually inspecting histograms and box plots, we gain valuable insights into the data's central tendency, spread, and potential outliers.
Inferential Statistics: Drawing Conclusions
Descriptive statistics summarize the observed data. Inferential statistics allow us to make inferences about the population from which the sample was drawn. For example, we might want to estimate the average height of all adults in the population based on our sample of 200. This involves constructing confidence intervals and hypothesis testing.
Confidence Intervals
A confidence interval provides a range of values within which the true population mean is likely to fall with a certain level of confidence (e.g., a 95% confidence interval). The width of the confidence interval depends on the sample size, the standard deviation, and the desired confidence level. A larger sample size generally leads to a narrower confidence interval, providing a more precise estimate of the population mean.
Hypothesis Testing
Hypothesis testing involves formulating a null hypothesis (e.g., the average height of the population is 170cm) and an alternative hypothesis (e.g., the average height of the population is not 170cm). We then use statistical tests (like a t-test) to determine whether the sample data provides sufficient evidence to reject the null hypothesis in favor of the alternative hypothesis. The p-value, a probability associated with the test, helps assess the strength of evidence against the null hypothesis. A low p-value (typically below 0.05) suggests strong evidence to reject the null hypothesis.
Exploring Relationships: (If applicable, based on additional data)
If additional data were collected alongside height (e.g., gender, age, ethnicity), we could investigate potential relationships between these variables and height. This could involve:
-
t-tests: Comparing the mean heights of different groups (e.g., males vs. females).
-
ANOVA (Analysis of Variance): Comparing the mean heights of three or more groups.
-
Correlation analysis: Examining the linear relationship between height and another continuous variable (e.g., weight). Correlation coefficients (e.g., Pearson's r) quantify the strength and direction of the linear relationship.
-
Regression analysis: Modeling the relationship between height and one or more predictor variables. This could help predict height based on other factors.
Advanced Statistical Techniques
More advanced statistical techniques might be employed depending on the research question and the characteristics of the data. These could include:
-
Non-parametric tests: These are used when the data doesn't meet the assumptions of parametric tests (e.g., normality). Examples include the Mann-Whitney U test and the Kruskal-Wallis test.
-
Multivariate analysis: Techniques like Principal Component Analysis (PCA) could be used to reduce the dimensionality of the data if multiple variables are involved.
-
Time series analysis: If the data were collected over time, time series analysis could be used to model trends and patterns in height over time.
Conclusion
The analysis of the heights of 200 adults offers a rich opportunity to apply a variety of statistical methods. From simple descriptive statistics to more advanced inferential techniques, the analysis can reveal valuable insights into the distribution of heights within the sample and potentially draw inferences about the broader population. The choice of statistical methods will depend on the research question, the characteristics of the data, and the assumptions that can be reasonably made. Remember that proper data collection and careful consideration of potential biases are crucial for ensuring the validity and reliability of any statistical analysis. Visualizing the data through histograms and box plots provides a crucial first step in understanding the data's distribution and informing subsequent analyses. Ultimately, this seemingly simple dataset provides a powerful learning tool for understanding the principles of statistical analysis and their practical applications.
Latest Posts
Latest Posts
-
Which Of The Following Statements Is True Of Urination
May 12, 2025
-
Science Enables Understanding At These Three Levels
May 12, 2025
-
Click The Profit Projection Worksheet Tab
May 12, 2025
-
Alessandro Wants To Use His Desktop Computer To Video
May 12, 2025
-
What Specific Advancement Topics Are Discussed At The Cdb
May 12, 2025
Related Post
Thank you for visiting our website which covers about The Height Of 200 Adults Were Recorded And Divided . We hope the information provided has been useful to you. Feel free to contact us if you have any questions or need further assistance. See you next time and don't miss to bookmark.