The R Value Must Always Be Between -1 And 1
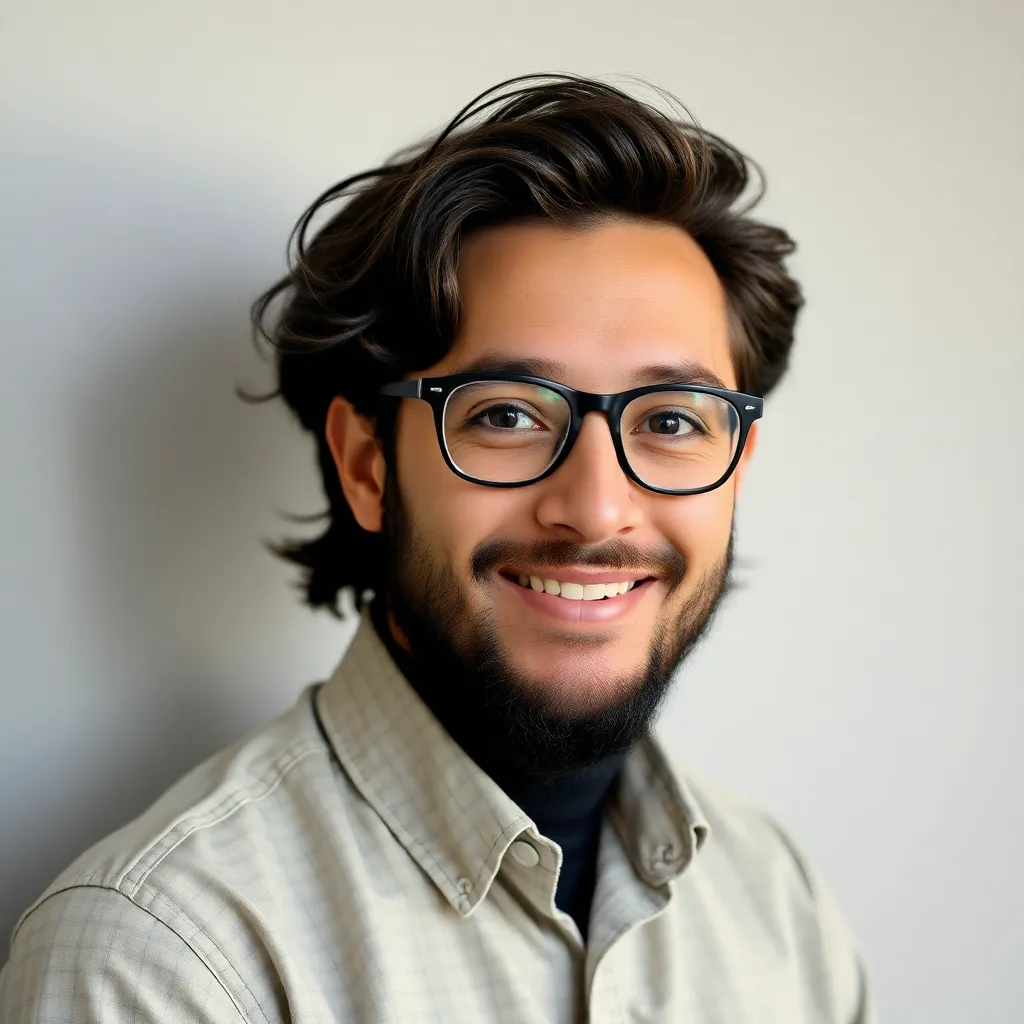
Onlines
Apr 25, 2025 · 5 min read

Table of Contents
The R Value: Always Between -1 and 1
The correlation coefficient, often represented as 'r', is a crucial statistical measure quantifying the strength and direction of a linear relationship between two variables. Understanding its properties, particularly the constraint that the r value must always be between -1 and 1, is essential for accurate interpretation and application in various fields, from finance and healthcare to environmental science and social research. This article delves deep into this fundamental concept, exploring its implications and practical applications.
Understanding the Correlation Coefficient (r)
Before diving into the range restriction, let's solidify our understanding of what 'r' represents. The correlation coefficient measures the linear association between two variables. This means it assesses how well the data points can be approximated by a straight line. A high correlation implies the data points cluster closely around a straight line, while a low correlation suggests a weaker, less linear relationship.
Key Characteristics of 'r':
- Strength: The absolute value of 'r' (|r|) indicates the strength of the linear relationship. An |r| close to 1 signifies a strong relationship, while an |r| close to 0 indicates a weak relationship.
- Direction: The sign of 'r' indicates the direction of the relationship. A positive 'r' suggests a positive correlation (as one variable increases, the other tends to increase), while a negative 'r' suggests a negative correlation (as one variable increases, the other tends to decrease).
Why the R Value is Always Between -1 and 1
The fact that -1 ≤ r ≤ 1 is not arbitrary; it's a direct consequence of the mathematical formula used to calculate the correlation coefficient. The formula involves standardizing the variables (subtracting the mean and dividing by the standard deviation) and then summing the products of these standardized values.
Mathematical Proof (Simplified):
A rigorous mathematical proof requires advanced linear algebra and covariance concepts. However, a simplified intuitive explanation can be given. The correlation coefficient is essentially a normalized measure of covariance. Covariance itself measures how much two variables change together. However, covariance's scale is dependent on the scales of the variables, making comparisons difficult. The correlation coefficient normalizes this by dividing by the product of the standard deviations, thus creating a unitless measure that always falls within the range of -1 to 1.
Think of it this way: The standardization process ensures that the values being multiplied are always within a certain range. The sum of these products, when normalized, cannot exceed 1 (perfect positive correlation) or fall below -1 (perfect negative correlation). Any deviation from perfect correlation results in a value between these extremes.
Interpreting the Range of 'r'
Understanding the range of 'r' is vital for interpreting the strength and direction of the relationship:
- r = 1: Perfect positive linear correlation. All data points lie exactly on a straight line with a positive slope.
- 0 < r < 1: Positive linear correlation. The strength of the correlation increases as 'r' approaches 1.
- r = 0: No linear correlation. There's no linear relationship between the variables; the data points show no discernible trend. Note that this doesn't necessarily imply no relationship; it simply means there's no linear relationship. A non-linear relationship might exist.
- -1 < r < 0: Negative linear correlation. The strength of the correlation increases as 'r' approaches -1.
- r = -1: Perfect negative linear correlation. All data points lie exactly on a straight line with a negative slope.
Misinterpretations of 'r' and Common Pitfalls
While 'r' is a powerful tool, it's crucial to avoid common misinterpretations:
- Correlation does not equal causation: A strong correlation between two variables doesn't automatically imply that one causes the other. There might be a third, unobserved variable influencing both. For example, a strong correlation between ice cream sales and drowning incidents doesn't mean ice cream causes drowning; both are likely influenced by the hot weather.
- Non-linear relationships: 'r' only measures linear relationships. A strong non-linear relationship might exist even if 'r' is close to 0. Visualizing the data with scatter plots is essential to detect such relationships.
- Outliers: Outliers can significantly influence the value of 'r'. A single outlier can drastically alter the correlation coefficient, leading to misleading conclusions. Robust correlation methods exist to mitigate the impact of outliers.
- Sample size: The reliability of 'r' depends on the sample size. Larger samples generally provide more reliable estimates of the population correlation.
Applications of the Correlation Coefficient
The correlation coefficient finds widespread application in diverse fields:
- Finance: Assessing the relationship between stock prices, interest rates, and economic indicators. Portfolio diversification strategies are often based on correlation analysis.
- Healthcare: Studying the relationship between lifestyle factors (diet, exercise) and health outcomes (heart disease, diabetes).
- Environmental Science: Analyzing the relationship between pollution levels and environmental damage.
- Social Sciences: Investigating correlations between social factors (income, education) and various social outcomes (crime rates, health disparities).
- Engineering: Evaluating the relationship between different design parameters and performance metrics.
Beyond Pearson's r: Other Correlation Measures
While Pearson's correlation coefficient (the 'r' we've been discussing) is widely used, it's important to know that other correlation measures exist, each suited to specific data types and scenarios:
- Spearman's rank correlation: Used for non-parametric data (data that doesn't follow a normal distribution) or when the relationship is non-linear but monotonic (always increasing or always decreasing).
- Kendall's tau correlation: Another non-parametric measure, often preferred over Spearman's when dealing with ties in the data.
- Partial correlation: Measures the correlation between two variables while controlling for the influence of other variables.
The choice of correlation measure depends on the nature of the data and the research question.
Conclusion: The Importance of Understanding the R Value
The correlation coefficient, constrained to the range of -1 to 1, is a fundamental tool in statistical analysis. Understanding its limitations and appropriate interpretation is critical for drawing valid conclusions from data. While a strong correlation can suggest important relationships, it's crucial to avoid causal inferences and consider other factors that might influence the observed relationship. Careful consideration of the data, appropriate statistical methods, and visual inspection of the data are vital steps in interpreting the correlation coefficient and extracting meaningful insights. By mastering the intricacies of 'r', researchers and analysts can unlock valuable information hidden within their data, leading to better decision-making across numerous fields. Remember, the seemingly simple range of -1 to 1 encompasses a world of statistical power and interpretive nuance.
Latest Posts
Latest Posts
-
Pulmonary Choking Agents Primarily Enter The Victim By What Means
Apr 25, 2025
-
Algebra Nation Section 6 Topic 7 Answers
Apr 25, 2025
-
Unit 4 Progress Check Frq Part A
Apr 25, 2025
-
Armando Toma Clases En La Universidad
Apr 25, 2025
-
What Lymphatic Component Serves To Monitor The Bacterial Load
Apr 25, 2025
Related Post
Thank you for visiting our website which covers about The R Value Must Always Be Between -1 And 1 . We hope the information provided has been useful to you. Feel free to contact us if you have any questions or need further assistance. See you next time and don't miss to bookmark.