The Study Of Statistics Rests On What Two Major Concepts
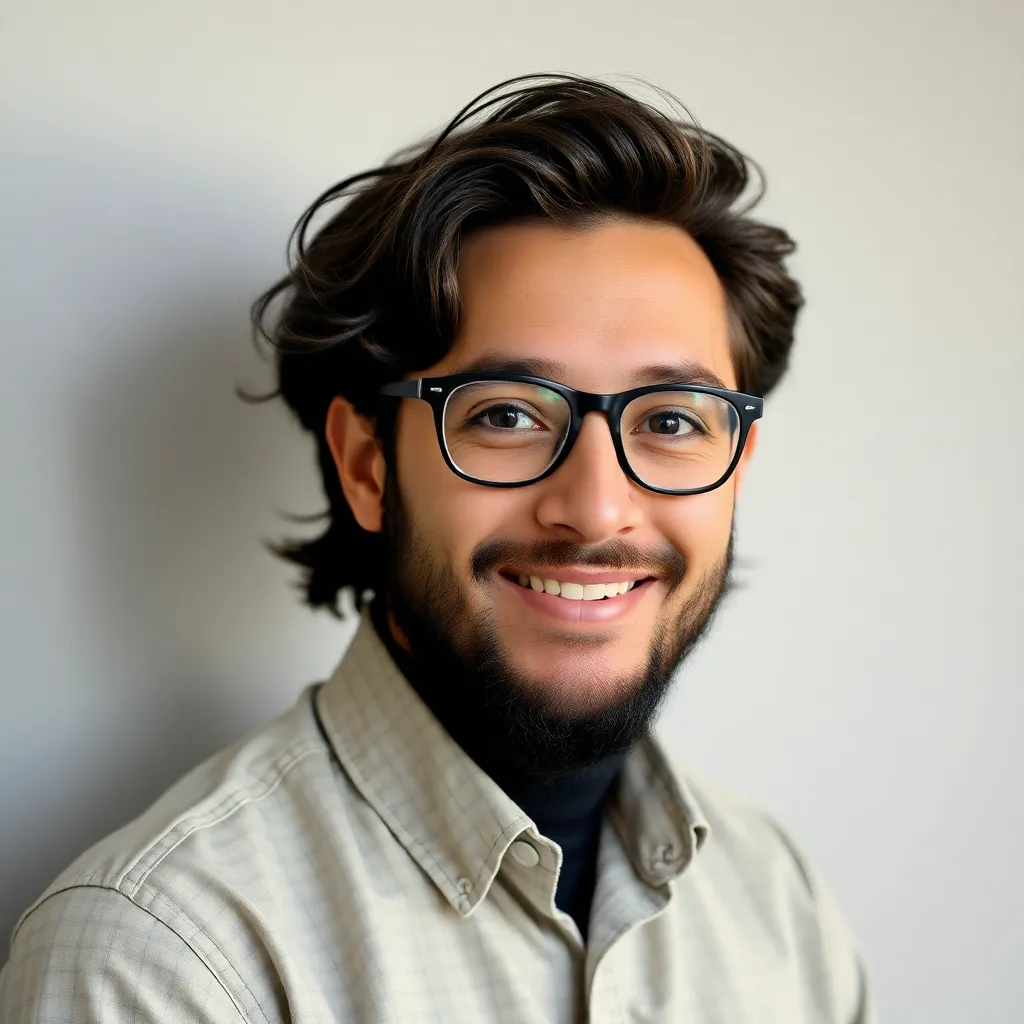
Onlines
May 12, 2025 · 6 min read
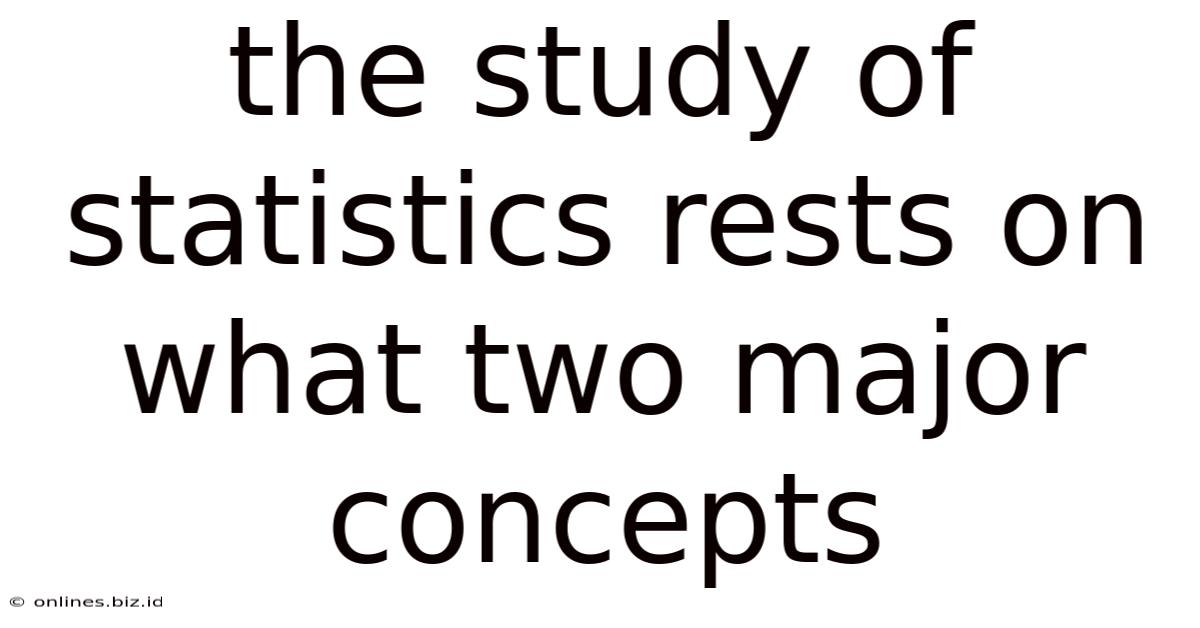
Table of Contents
The Study of Statistics Rests on What Two Major Concepts?
The field of statistics, a cornerstone of modern data analysis and decision-making, fundamentally rests upon two major interconnected concepts: probability and inference. While seemingly distinct, these concepts are deeply intertwined, forming the very foundation upon which statistical methods are built and applied across diverse disciplines. Understanding these concepts is crucial for anyone seeking to interpret data effectively, draw meaningful conclusions, and make informed decisions based on evidence.
Probability: The Foundation of Chance
Probability, at its core, deals with the quantification of uncertainty. It provides a framework for understanding and predicting the likelihood of different events occurring. Instead of dealing with definite outcomes, probability focuses on the chances of various possibilities, acknowledging the inherent randomness present in many real-world phenomena. This concept is fundamental to statistics because many statistical methods rely on probabilistic models to understand and analyze data.
Key Aspects of Probability:
-
Random Variables: These are variables whose values are numerical outcomes of random phenomena. For example, the number of heads observed when flipping a coin five times is a random variable. Understanding the behavior of random variables is central to many statistical analyses.
-
Probability Distributions: These are mathematical functions that describe the likelihood of different outcomes for a random variable. Common examples include the normal distribution (bell curve), binomial distribution (for binary outcomes), and Poisson distribution (for count data). The choice of appropriate probability distribution is crucial for accurate modeling and analysis.
-
Expected Value and Variance: These are measures that summarize the central tendency and dispersion of a probability distribution. The expected value represents the average outcome, while the variance quantifies the spread or variability of the outcomes. These measures provide concise summaries of complex probability distributions.
-
Conditional Probability: This concept explores the probability of an event occurring given that another event has already occurred. It's crucial for understanding dependencies between variables and is fundamental to concepts like Bayes' theorem and statistical independence.
-
Bayes' Theorem: A cornerstone of Bayesian statistics, this theorem provides a way to update the probability of an event based on new evidence. It's used extensively in fields like machine learning, medical diagnosis, and risk assessment.
Probability in Statistical Methods:
Probability theory underpins many essential statistical techniques, including:
-
Hypothesis Testing: Determining whether observed data supports or refutes a specific hypothesis often involves calculating probabilities associated with the observed data under the assumption that the hypothesis is true.
-
Confidence Intervals: These intervals provide a range of plausible values for a population parameter, with a specified level of confidence. The calculation of confidence intervals relies heavily on probability distributions.
-
Regression Analysis: Predictive modeling techniques, such as linear regression, use probability to estimate the uncertainty associated with predictions.
-
Simulation Studies: Probability is used to simulate random processes and explore the behavior of statistical methods under different conditions.
Statistical Inference: Drawing Conclusions from Data
Statistical inference bridges the gap between observed data and broader conclusions about the population from which the data was drawn. It involves using sample data to make inferences about the characteristics of a larger population, acknowledging that the sample is only a partial representation of the whole. This process is fraught with uncertainty, and probability plays a critical role in quantifying that uncertainty.
Key Aspects of Statistical Inference:
-
Sampling: The process of selecting a subset of individuals or observations from a larger population. The method of sampling is crucial for ensuring the sample is representative of the population and minimizing bias.
-
Estimators: These are statistical functions that provide estimates of population parameters based on sample data. Examples include the sample mean (estimating the population mean) and the sample variance (estimating the population variance).
-
Hypothesis Testing: This involves formulating a hypothesis about a population parameter and then using sample data to assess the evidence for or against that hypothesis. The process involves calculating a test statistic and comparing it to a critical value determined by the chosen significance level.
-
Confidence Intervals: These provide a range of plausible values for a population parameter, with a specified level of confidence. They incorporate the uncertainty inherent in estimating population parameters from sample data.
-
p-values: These represent the probability of observing data as extreme as, or more extreme than, the data actually observed, assuming the null hypothesis is true. P-values are used in hypothesis testing to assess the strength of evidence against the null hypothesis.
-
Type I and Type II Errors: These are errors that can occur in hypothesis testing. A Type I error occurs when the null hypothesis is rejected when it is actually true, while a Type II error occurs when the null hypothesis is not rejected when it is actually false. Understanding and minimizing these errors is crucial for accurate inference.
Types of Statistical Inference:
Statistical inference is broadly categorized into two main approaches:
-
Frequentist Inference: This approach interprets probabilities as long-run frequencies of events. It focuses on estimating population parameters and testing hypotheses using methods based on the sampling distribution of estimators. Confidence intervals and p-values are central to frequentist inference.
-
Bayesian Inference: This approach interprets probabilities as degrees of belief or subjective probabilities. It uses Bayes' theorem to update prior beliefs about population parameters in light of new data. Bayesian inference provides posterior distributions that represent the updated beliefs about the parameters.
Statistical Inference in Practice:
Statistical inference is applied widely across various fields, including:
-
Medical Research: Analyzing clinical trial data to assess the effectiveness of new treatments.
-
Social Sciences: Studying social phenomena and evaluating the impact of interventions.
-
Business and Finance: Making decisions based on market research, financial modeling, and risk management.
-
Environmental Science: Monitoring environmental changes and assessing the impact of human activities.
-
Engineering: Designing and testing products and systems.
The Interplay Between Probability and Inference
Probability and inference are not simply separate concepts; they are deeply intertwined and mutually supportive. Probability provides the theoretical framework for understanding uncertainty and randomness, while inference uses this framework to draw conclusions from data. Without probability, statistical inference would lack a rigorous foundation for quantifying uncertainty. Conversely, without inference, probability would remain largely a theoretical exercise, lacking practical application in real-world problems.
Examples of the Interplay:
-
Estimating a Population Mean: We use probability distributions (like the t-distribution) to construct confidence intervals for the population mean, which quantifies the uncertainty in our estimate based on sample data.
-
Testing a Hypothesis about a Population Proportion: We use probability to determine the p-value, which assesses the strength of evidence against the null hypothesis based on the probability of observing the sample data if the null hypothesis were true.
-
Regression Analysis: We use probability to model the relationship between variables, accounting for the random variation in the data. This allows us to make predictions and assess the uncertainty associated with those predictions.
Conclusion: A Powerful Duo for Data Analysis
In conclusion, the study of statistics is fundamentally grounded in the concepts of probability and inference. Probability provides the mathematical framework for quantifying uncertainty and randomness, while inference utilizes this framework to draw meaningful conclusions from data. The interplay between these two concepts is crucial for the development and application of statistical methods across diverse fields. A deep understanding of both probability and inference is essential for anyone seeking to analyze data effectively, make informed decisions, and contribute to evidence-based reasoning in their respective domains. Mastering these concepts empowers individuals to navigate the complexities of data-driven decision-making in the modern world, unlocking insights and driving progress across various sectors. The power of statistics lies in its ability to transform raw data into actionable knowledge, and this transformation relies fundamentally on the elegant interplay between probability and inference.
Latest Posts
Latest Posts
-
Diagnosis Involves The Ruling Out Of Alternative Diagnoses
May 12, 2025
-
After Performance Evaluations Are Completed For A Group Of Sailors
May 12, 2025
-
How Is The Surgery Section Organized
May 12, 2025
-
Barbara Strozzi Performed In The Academies In
May 12, 2025
-
Drag The Labels Onto The Diagram To Identify The Leukocytes
May 12, 2025
Related Post
Thank you for visiting our website which covers about The Study Of Statistics Rests On What Two Major Concepts . We hope the information provided has been useful to you. Feel free to contact us if you have any questions or need further assistance. See you next time and don't miss to bookmark.