The Variability Of A Statistic Is Described By
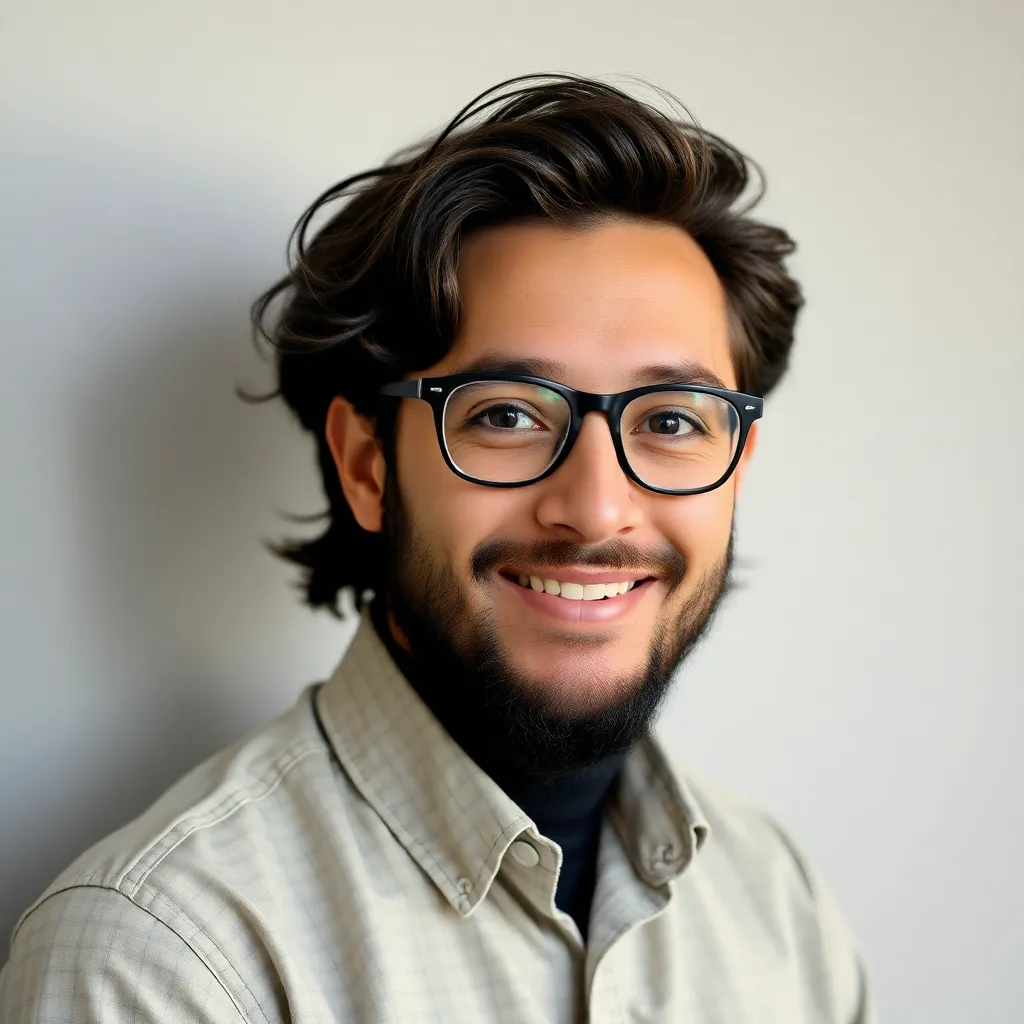
Onlines
Apr 22, 2025 · 6 min read

Table of Contents
The Variability of a Statistic: A Deep Dive into Dispersion and its Implications
The variability of a statistic is a crucial concept in statistics and data analysis. It describes how spread out or dispersed the data points are around a central tendency, such as the mean or median. Understanding variability is essential for drawing accurate conclusions and making informed decisions based on data. This article will delve into the various aspects of statistical variability, exploring its different measures, interpretations, and implications across various fields.
Understanding Statistical Variability
Variability, also known as dispersion or scatter, quantifies the extent to which individual data points deviate from a central value. A high degree of variability indicates a wide spread of data, while low variability suggests that data points cluster closely around the central value. This difference in spread significantly impacts the reliability and interpretation of statistical results. For example, a study showing a large variability in treatment effectiveness suggests that the treatment may not be consistently effective for all individuals.
Why is Understanding Variability Important?
Understanding variability is paramount for several reasons:
-
Accurate Inference: Variability directly impacts the precision of statistical inferences. High variability can lead to less precise estimates and wider confidence intervals, making it harder to draw definitive conclusions.
-
Risk Assessment: In finance, variability (often measured by standard deviation or variance) is crucial for assessing risk. Higher variability indicates greater uncertainty and potential for extreme outcomes.
-
Process Control: In manufacturing and other industrial processes, understanding variability helps identify and control sources of inconsistencies, leading to improved quality and efficiency.
-
Comparative Analysis: Comparing different groups or treatments requires understanding variability. Differences in means might be statistically significant only if the variability within each group is relatively low.
-
Predictive Modeling: Accurate predictive models require accounting for variability in the data. Models that fail to account for variability can lead to inaccurate predictions.
Measures of Variability
Several statistical measures quantify variability. The most common include:
1. Range
The range is the simplest measure of variability, representing the difference between the maximum and minimum values in a dataset. While easy to calculate, it's highly sensitive to outliers and doesn't consider the distribution of data points within the range. Therefore, it's often used as a preliminary measure or in conjunction with other measures.
Example: For the dataset {2, 5, 7, 9, 12}, the range is 12 - 2 = 10.
2. Interquartile Range (IQR)
The IQR is a more robust measure of variability than the range, as it's less sensitive to outliers. It represents the difference between the third quartile (Q3) and the first quartile (Q1) of a dataset. The IQR represents the spread of the middle 50% of the data.
Example: If Q1 = 5 and Q3 = 10, then the IQR = 10 - 5 = 5.
3. Variance
The variance is a measure of the average squared deviation of each data point from the mean. Squaring the deviations ensures that both positive and negative deviations contribute positively to the overall variability. The variance is denoted by σ² (sigma squared) for population variance and s² for sample variance. A higher variance indicates greater variability.
Formula (population variance): σ² = Σ(xᵢ - μ)² / N, where xᵢ are the individual data points, μ is the population mean, and N is the population size.
Formula (sample variance): s² = Σ(xᵢ - x̄)² / (n - 1), where xᵢ are the individual data points, x̄ is the sample mean, and n is the sample size. The (n-1) term is used for an unbiased estimator of the population variance.
4. Standard Deviation
The standard deviation is the square root of the variance. It's expressed in the same units as the original data, making it easier to interpret than the variance. Like the variance, a higher standard deviation indicates greater variability. It’s denoted by σ (sigma) for population standard deviation and s for sample standard deviation.
Formula (population standard deviation): σ = √σ²
Formula (sample standard deviation): s = √s²
5. Coefficient of Variation (CV)
The coefficient of variation is a relative measure of variability, expressed as a percentage. It's useful for comparing the variability of datasets with different means or units. It's calculated by dividing the standard deviation by the mean and multiplying by 100.
Formula: CV = (Standard Deviation / Mean) * 100
Interpreting Variability
The interpretation of variability depends on the context and the specific measure used. A large standard deviation indicates a wide spread of data, suggesting less precision and greater uncertainty. A small standard deviation indicates that data points cluster closely around the mean, suggesting higher precision and less uncertainty. The IQR provides a robust measure of spread, less affected by outliers. The range offers a quick but less informative measure. The coefficient of variation allows for comparisons across datasets with different scales.
Variability in Different Contexts
Variability plays a significant role in various fields:
1. Finance: Risk Management
In finance, variability, often measured by standard deviation, is a key indicator of risk. Higher variability in investment returns indicates greater uncertainty and potential for both higher gains and greater losses. Portfolio diversification aims to reduce overall variability by investing in assets with low correlations.
2. Quality Control: Process Capability
In manufacturing, variability in product characteristics (e.g., dimensions, weight) affects product quality. Statistical process control techniques aim to minimize variability and maintain consistent product quality. Process capability analysis assesses the ability of a process to meet specified tolerances.
3. Healthcare: Treatment Effectiveness
In healthcare, variability in treatment responses highlights the complexity of human biology and the importance of personalized medicine. Understanding variability helps identify factors that influence treatment outcomes and develop more effective treatment strategies.
4. Environmental Science: Climate Change
Variability in climate data (e.g., temperature, precipitation) is crucial for understanding climate change. Analyzing variability helps identify trends, predict future climate patterns, and assess the impacts of climate change on various ecosystems.
5. Social Sciences: Survey Research
In social sciences, variability in survey responses reflects the diversity of opinions and behaviors within a population. Understanding variability is crucial for drawing accurate inferences about the population based on sample data.
Reducing Variability
Depending on the context, there might be strategies to reduce variability:
-
Improved Measurement Techniques: More precise measurement tools and methods can reduce measurement error and variability.
-
Process Optimization: Identifying and eliminating sources of variability in manufacturing processes can lead to improved product quality.
-
Standardized Procedures: Implementing standardized procedures across different settings can reduce variability caused by inconsistencies in methods.
-
Data Cleaning: Removing outliers and erroneous data can reduce variability and improve the accuracy of analysis.
-
Experimental Design: Careful experimental design can minimize variability due to confounding factors.
Conclusion
The variability of a statistic is a fundamental concept with far-reaching implications across numerous fields. Understanding different measures of variability, their interpretations, and their role in various contexts is crucial for making informed decisions based on data. By appreciating the significance of variability, researchers, analysts, and decision-makers can better interpret results, make more accurate predictions, and ultimately, improve the quality and effectiveness of their work. The careful consideration and management of variability are essential components of robust and reliable data analysis.
Latest Posts
Latest Posts
-
Benedetta Y Ana Maria Son De Argentina Ellas Son
Apr 23, 2025
-
Everyday Use By Alice Walker Characters
Apr 23, 2025
-
The Elements In The Term Cardiomyopathy In Order Are
Apr 23, 2025
-
Single Replacement Reaction Stoichiometry And Percent Yield
Apr 23, 2025
-
Unit 6 Progress Check Mcq Ap Biology
Apr 23, 2025
Related Post
Thank you for visiting our website which covers about The Variability Of A Statistic Is Described By . We hope the information provided has been useful to you. Feel free to contact us if you have any questions or need further assistance. See you next time and don't miss to bookmark.